Versatile Skill Control via Self-supervised Adversarial Imitation of Unlabeled Mixed Motions
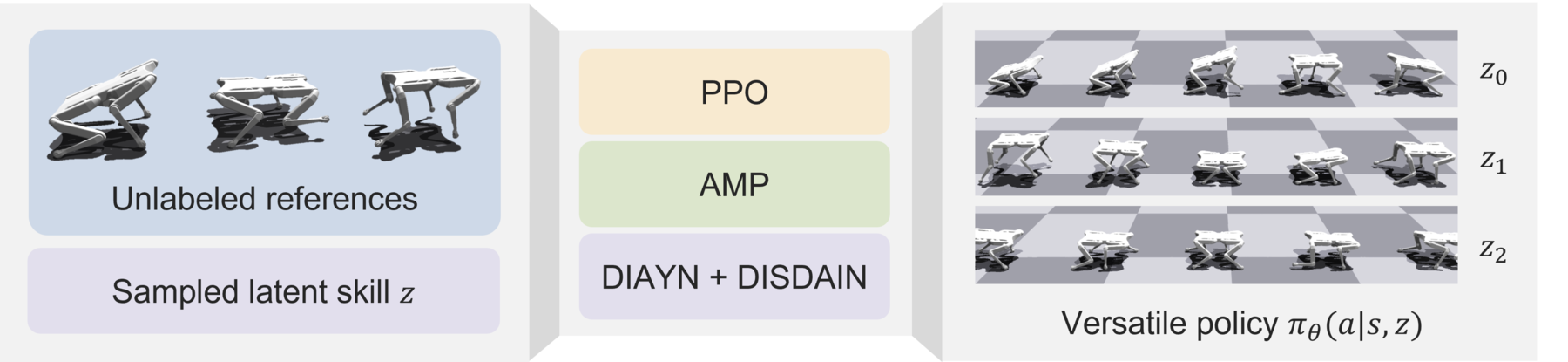
Learning diverse skills is one of the main challenges in robotics. To this end, imitation learning approaches have achieved impressive results. These methods require explicitly labeled datasets or assume consistent skill execution to enable learning and active control of individual behaviors, which limits their applicability. In this work, we propose a cooperative adversarial method for obtaining single versatile policies with controllable skill sets from unlabeled datasets containing diverse state transition patterns by maximizing their discriminability. Moreover, we show that by utilizing unsupervised skill discovery in the generative adversarial imitation learning framework, novel and useful skills emerge with successful task fulfillment. Finally, the obtained versatile policies are tested on an agile quadruped robot called Solo 8 and present faithful replications of diverse skills encoded in the demonstrations.
Author(s): | Li, Chenhao and Blaes, Sebastian and Kolev, Pavel and Vlastelica, Marin and Frey, Jonas and Martius, Georg |
Book Title: | Versatile Skill Control via Self-supervised Adversarial Imitation of Unlabeled Mixed Motions |
Pages: | 2944--2950 |
Year: | 2023 |
Publisher: | IEEE |
Bibtex Type: | Conference Paper (inproceedings) |
DOI: | 10.1109/ICRA48891.2023.10160421 |
State: | Accepted |
URL: | https://doi.org/10.1109/ICRA48891.2023.10160421 |
BibTex
@inproceedings{Li2023:Cassi, title = {Versatile Skill Control via Self-supervised Adversarial Imitation of Unlabeled Mixed Motions}, booktitle = {Versatile Skill Control via Self-supervised Adversarial Imitation of Unlabeled Mixed Motions}, abstract = {Learning diverse skills is one of the main challenges in robotics. To this end, imitation learning approaches have achieved impressive results. These methods require explicitly labeled datasets or assume consistent skill execution to enable learning and active control of individual behaviors, which limits their applicability. In this work, we propose a cooperative adversarial method for obtaining single versatile policies with controllable skill sets from unlabeled datasets containing diverse state transition patterns by maximizing their discriminability. Moreover, we show that by utilizing unsupervised skill discovery in the generative adversarial imitation learning framework, novel and useful skills emerge with successful task fulfillment. Finally, the obtained versatile policies are tested on an agile quadruped robot called Solo 8 and present faithful replications of diverse skills encoded in the demonstrations.}, pages = {2944--2950}, publisher = {IEEE}, year = {2023}, slug = {li2023-cassi}, author = {Li, Chenhao and Blaes, Sebastian and Kolev, Pavel and Vlastelica, Marin and Frey, Jonas and Martius, Georg}, url = {https://doi.org/10.1109/ICRA48891.2023.10160421} }