Our research uses Computer Vision to learn digital humans that can perceive, learn, and act in virtual 3D worlds. This involves capturing the shape, appearance, and motion of real people as well as their interactions with each other and the 3D scene using monocular video. We leverage this to learn generative models of people and their behavior and evaluate these models by synthesizing realistic looking humans behaving in virtual worlds.
This work combines Computer Vision, Machine Learning, and Computer Graphics.
Director
Admin Team
+49 7071 601 1800
Department Highlights
Publication
Perceiving Systems
2024-09-01
GraspXL: Generating Grasping Motions for Diverse Objects at Scale
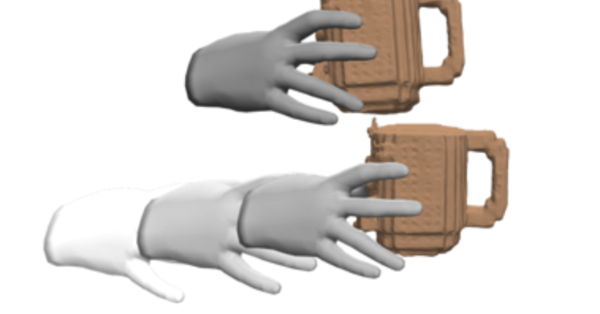