Systematic self-exploration of behaviors for robots in a dynamical systems framework
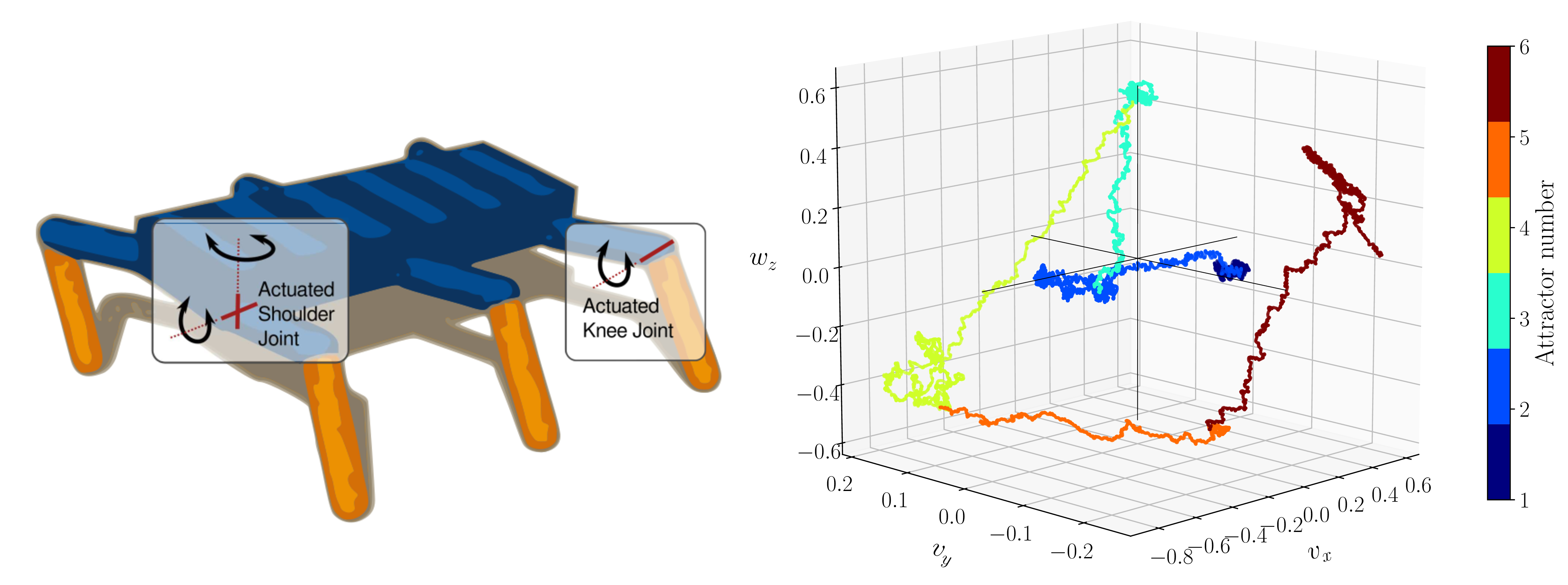
One of the challenges of this century is to understand the neural mechanisms behind cognitive control and learning. Recent investigations propose biologically plausible synaptic mechanisms for self-organizing controllers, in the spirit of Hebbian learning. In particular, differential extrinsic plasticity (DEP) [Der and Martius, PNAS 2015], has proven to enable embodied agents to self-organize their individual sensorimotor development, and generate highly coordinated behaviors during their interaction with the environment. These behaviors are attractors of a dynamical system. In this paper, we use the DEP rule to generate attractors and we combine it with a “repelling potential” which allows the system to actively explore all its attractor behaviors in a systematic way. With a view to a self-determined exploration of goal-free behaviors, our framework enables switching between different motion patterns in an autonomous and sequential fashion. Our algorithm is able to recover all the attractor behaviors in a toy system and it is also effective in two simulated environments. A spherical robot discovers all its major rolling modes and a hexapod robot learns to locomote in 50 different ways in 30min.
Author(s): | Cristina Pinneri and Georg Martius |
Book Title: | Proc. Artificial Life XI |
Pages: | 319-326 |
Year: | 2018 |
Publisher: | MIT Press, Cambridge, MA |
Project(s): | |
Bibtex Type: | Conference Paper (inproceedings) |
DOI: | 10.1162/isal_a_00062 |
URL: | https://www.mitpressjournals.org/doi/abs/10.1162/isal_a_00062 |
Electronic Archiving: | grant_archive |
BibTex
@inproceedings{PinneriMartius2018:Repeller, title = {Systematic self-exploration of behaviors for robots in a dynamical systems framework}, booktitle = {Proc.\ Artificial Life XI}, abstract = {One of the challenges of this century is to understand the neural mechanisms behind cognitive control and learning. Recent investigations propose biologically plausible synaptic mechanisms for self-organizing controllers, in the spirit of Hebbian learning. In particular, differential extrinsic plasticity (DEP) [Der and Martius, PNAS 2015], has proven to enable embodied agents to self-organize their individual sensorimotor development, and generate highly coordinated behaviors during their interaction with the environment. These behaviors are attractors of a dynamical system. In this paper, we use the DEP rule to generate attractors and we combine it with a “repelling potential” which allows the system to actively explore all its attractor behaviors in a systematic way. With a view to a self-determined exploration of goal-free behaviors, our framework enables switching between different motion patterns in an autonomous and sequential fashion. Our algorithm is able to recover all the attractor behaviors in a toy system and it is also effective in two simulated environments. A spherical robot discovers all its major rolling modes and a hexapod robot learns to locomote in 50 different ways in 30min.}, pages = {319-326}, publisher = {MIT Press, Cambridge, MA}, year = {2018}, slug = {systematic_self_exploration}, author = {Pinneri, Cristina and Martius, Georg}, url = {https://www.mitpressjournals.org/doi/abs/10.1162/isal_a_00062} }