Precise Imprecision
Probabilistic Numerical Methods assign Uncertainty to Deterministic Computations
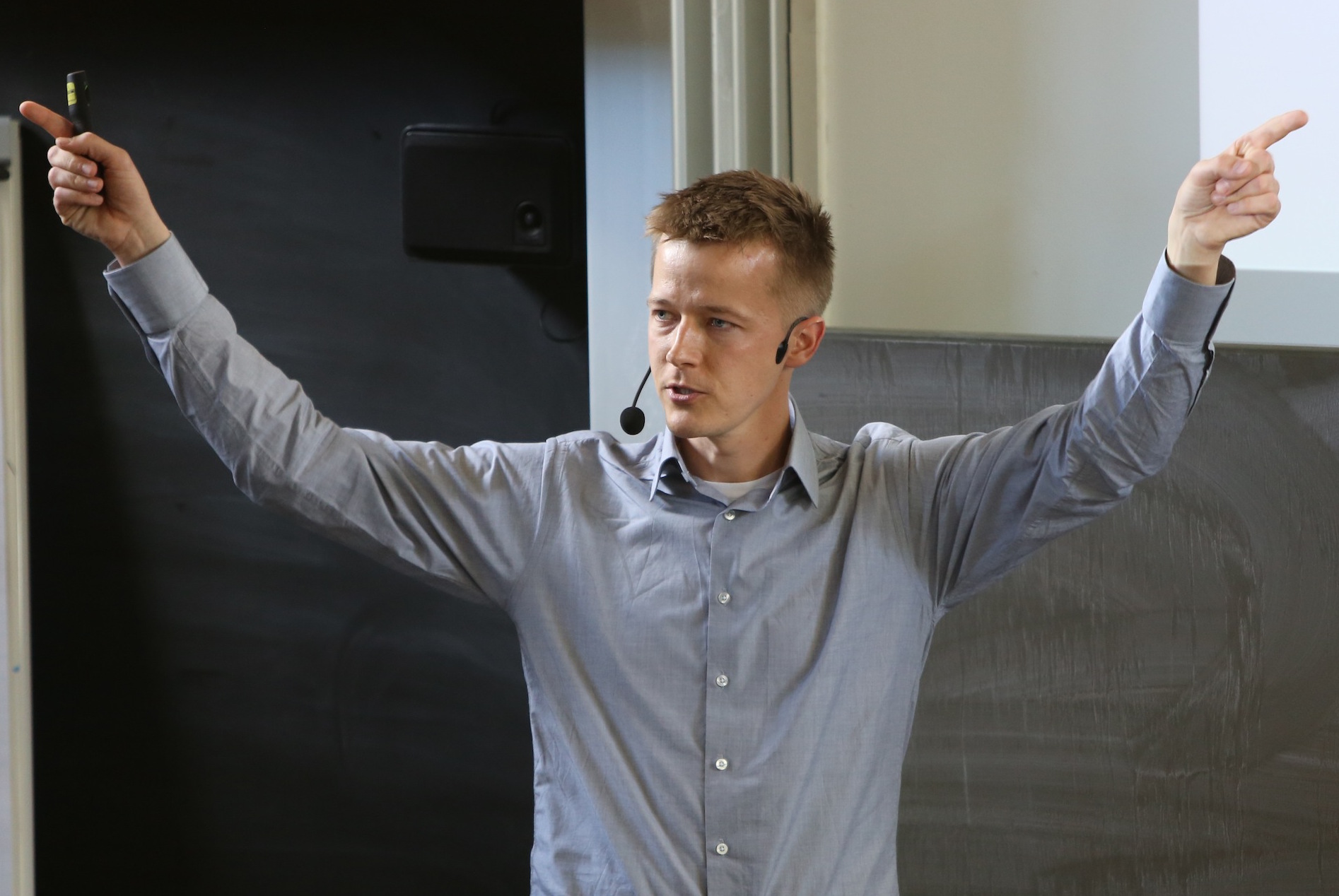
With a new approach, Scientists of the Max Planck Institute for Intelligent Systems aim to make numerical algorithms more efficient. During the next five years, this research project will be supported by the Emmy-Noether-Programme of the German Research Foundation (DFG) with nearly a million Euros. The applicant Dr. Philipp Hennig prevailed in a competitive process. With the start of two new PhD students in April this Emmy Noether Group takes up its research activities.
Intelligence, the ability to act under uncertainty, spans not just physical, but also computational scales: Modern machine learning algorithms, capable of building highly structured models and taking complicated decisions, rely on low-level computational routines for tasks like integration, optimization, and elementary algebraic computations. These methods are often taken as black boxes and not given much thought. The scientists in the newly established group believe there is still plenty of room for improvement at this bottom end of the intelligence hierarchy.
The insight that numerical methods are learning machines is not new, but only beginning to achieve its full impact. A crucial insight in the preparatory work that led to the award of the grant is that many classic algorithms can be interpreted in a mathematically precise way as statistical estimators using certain implicit modelling assumptions. This insight gives a firm mathematical foundation to stand on: The classic algorithms, used and trusted in a myriad inner loops every day, are performing statistical inference, at low cost and high reliability. Extending from this basis, the group builds modified algorithms that can share information between related computations, propagate uncertainty through chains of computations, and use tangible prior information to tailor their behaviour to specific, challenging problems.
A larger question is whether the probabilistic interpretation can also shed light on big old questions in numerics. The notion of a probabilistic prior is a powerful tool to describe an algorithm's implicit assumpti