AI as a resource: strategy, uncertainty, and societal welfare
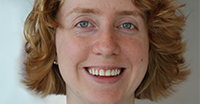
In recent years, humanity has been faced with a new resource - artificial intelligence. AI can be a boon to society, or can also have negative impacts, especially with inappropriate use. My research agenda studies the societal impact of AI, particularly focusing on AI as a resource and on the strategic decisions that agents make in deciding how to use it.
In this talk, I will consider some of the key strategic questions that arise in this framework: the decisions that agents make in jointly constructing and sharing AI models, and the decisions that they make in dividing tasks between their own expertise and the expertise of a model. The first of these questions has motivated my work on "model-sharing games", which models scenarios such as federated learning or data cooperatives. In this setting, we view agents with data as game-theoretic players and analyze questions of stability, optimality, and fairness. Secondly, I will describe some of my work in modeling human-algorithm collaboration. In particular, I will describe work on best-item recovery in categorical prediction, showing how differential accuracy rates and anchoring on algorithmic suggestions can influence overall performance and describe some ongoing work inspired by human-LLM interaction.
Speaker Biography
Kate Donahue (Cornell University)
PhD Candidate, Computer Science Department
Kate Donahue is a sixth year computer science PhD candidate at Cornell advised by Jon Kleinberg. She works on algorithmic problems relating to the societal impact of AI such as fairness, human/AI collaboration and game-theoretic models of federated learning. Her work has been supported by an NSF fellowship and recognized by a FAccT Best Paper award. During her PhD, she has interned at Amazon, Google, and Microsoft Research. In fall 2025, she will be starting as an assistant professor of CS at UIUC, and during the '24-'25 academic year she will be a METEOR postdoc at MIT and a long-term participant in the Simons year on LLMs.