Predictive vs causal targeting of social interventions
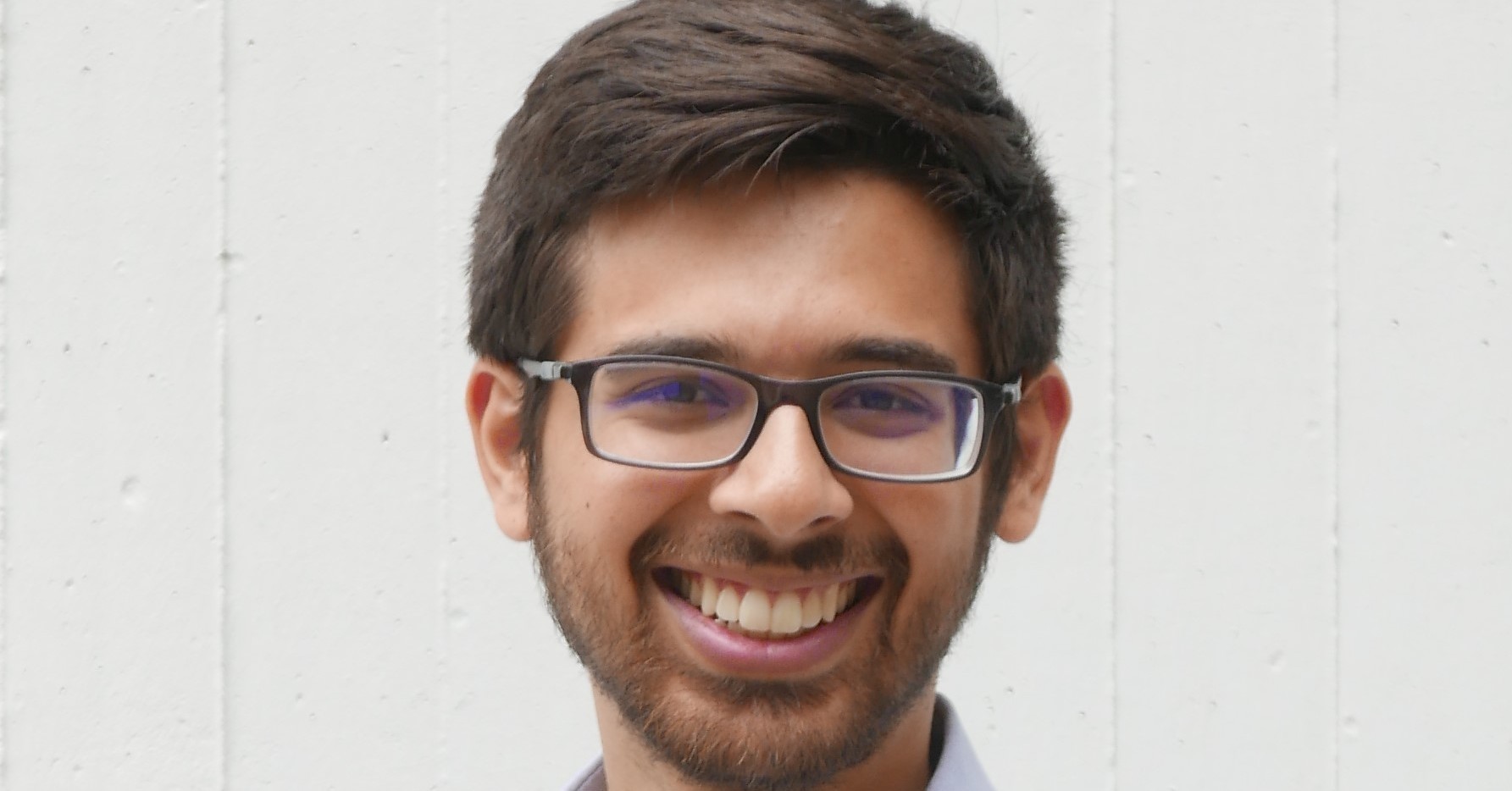
Machine learning is increasingly used to inform which people receive limited interventions in a wide range of domains, including healthcare, human services, education, development, and more. What is the right quantity for such models to predict?
In most applications, practitioners develop risk models which predict whether an individual will suffer an adverse outcome in the status quo. While it is often hoped that such individuals will also experience the largest benefit from an intervention, there is little empirical evidence in policy settings to verify this intuition. On the other hand, while causal targeting strategies could in principle improve welfare, they are difficult to learn without large randomized controlled trials which are rare in practice. We first provide an empirical assessment of the causal merits of predictive targeting strategies in a set of real-world policy domains where RCTs were conducted. In the domains we study, risk predictions are at best a noisy proxy for treatment effects. We then turn to the design of policies which optimally balance targeting high-risk individuals with gathering randomized data to learn causal effects. We give sample complexity guarantees and efficient algorithms to learn policies on the Pareto frontier between these goals. Finally, we use real human services data from Pittsburgh to illustrate how our proposed designs achieve the best of both worlds: public service providers can learn causal effects at near-optimal rates with only a modest reduction in immediate performance.
Speaker Biography
Bryan Wilder (Carnegie Mellon University)
Assistant Professor, Machine Learning Department
Bryan Wilder's research focuses on AI for equitable, data-driven decision making in high-stakes social settings, integrating methods from machine learning, optimization, and causal inference. Much of his work is motivated by applications in public health, medicine, and human services. He completed my PhD in Computer Science at Harvard University. Before joining CMU, he was a postdoctoral Schmidt Science Fellow at the Harvard School of Public Health. At CMU he directs the Lab for AI and Social Impact (LASI). The group’s work focuses on developing machine learning methods that support robust and equitable decision making. Current domains include maternal and child health, human services, and infectious diseases. The research is funded by awards from Schmidt Futures, NSF, NIH, and the CDC. Bryan Wilder serves as Chair of the Board of Directors for EAAMO and the associated ACM EAAMO conference.