Towards Scalable Information Elicitation for Oversight in Human-AI Systems
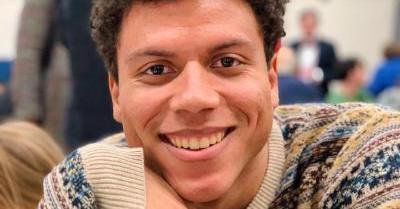
The growing complexity of AI outputs, particularly those generated by large language models, poses challenges for comprehensive human oversight. In this work, we propose a scalable information elicitation mechanism to incentivize truthful and consistent reasoning in human-AI systems. Our approach leverages pre-trained language models to estimate mutual information between agent outputs using the Difference of Entropies (DoE) estimator. Through theoretical analysis, we demonstrate the mechanism's incentive-compatibility properties and examine the scaling laws of its implementability. We evaluate the DoE estimator on two datasets: machine translation and structured paper reviews. Our results show that the estimator effectively detects manipulated and inconsistent model outputs, exhibiting sensitivity to various manipulation strategies and levels of information content. This work highlights the potential of information-theoretic approaches for scalable oversight in language model applications. We discuss the limitations of our approach, including model refusal and potential collusion, and outline future research directions in efficient estimation techniques and value alignment.
Speaker Biography
Zachary Robertson (Stanford University)
Ph.D. student Computer Science
Zachary Robertson is a Computer Science Ph.D. student at Stanford University, studying human-AI alignment. His work focuses on creating better ways for humans and AI to work together safely and effectively. Zachary combines ideas from different fields like economics, information theory, and complex systems in his research. He has a master's degree from the University of Illinois and a bachelor's from the University of Chicago. Before Stanford, Zachary gained experience through internships at Google and other tech companies, working on various AI and machine learning projects. His goal is to develop AI systems that can understand and follow human preferences more accurately.