Exemplar-based Prediction of Object Properties from Local Shape Similarity
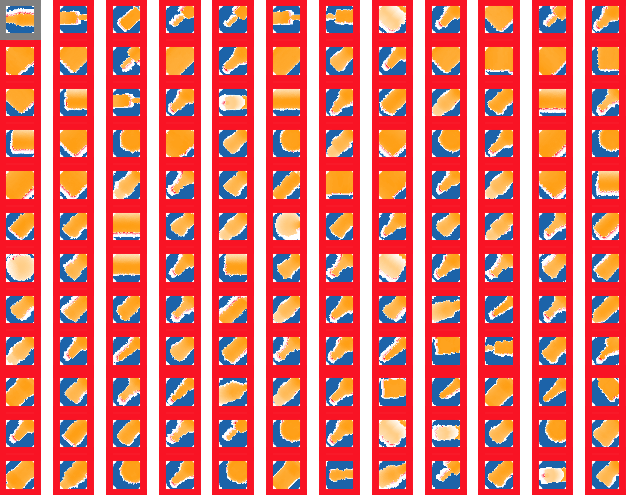
We propose a novel method that enables a robot to identify a graspable object part of an unknown object given only noisy and partial information that is obtained from an RGB-D camera. Our method combines the benefits of local with the advantages of global methods. It learns a classifier that takes a local shape representation as input and outputs the probability that a grasp applied at this location will be successful. Given a query data point that is classified in this way, we can retrieve all the locally similar training data points and use them to predict latent global object shape. This information may help to further prune positively labeled grasp hypotheses based on, e.g. relation to the predicted average global shape or suitability for a specific task. This prediction can also guide scene exploration to prune object shape hypotheses. To learn the function that maps local shape to grasp stability we use a Random Forest Classifier. We show that our method reaches the same classification performance as the current state-of-the-art on this dataset which uses a Convolutional Neural Network. Additionally, we exploit the natural ability of the Random Forest to cluster similar data. For a positively predicted query data point, we retrieve all the locally similar training data points that are associated with the same leaf nodes of the Random Forest. The main insight from this work is that local object shape that affords a grasp is also a good predictor of global object shape. We empirically support this claim with quantitative experiments. Additionally, we demonstrate the predictive capability of the method on some real data examples.
Author(s): | Bohg, Jeannette and Kappler, Daniel and Schaal, Stefan |
Book Title: | Proceedings of the IEEE International Conference on Robotics and Automation (ICRA) 2016 |
Year: | 2016 |
Month: | May |
Day: | 16-21 |
Publisher: | IEEE |
Project(s): | |
Bibtex Type: | Conference Paper (inproceedings) |
DOI: | 10.1109/ICRA.2016.7487516 |
Event Name: | IEEE International Conference on Robotics and Automation |
Event Place: | Stockholm, Sweden |
State: | Published |
Electronic Archiving: | grant_archive |
Attachments: |
BibTex
@inproceedings{2016_ICRA_bks, title = {Exemplar-based Prediction of Object Properties from Local Shape Similarity}, booktitle = {Proceedings of the IEEE International Conference on Robotics and Automation (ICRA) 2016}, abstract = {We propose a novel method that enables a robot to identify a graspable object part of an unknown object given only noisy and partial information that is obtained from an RGB-D camera. Our method combines the benefits of local with the advantages of global methods. It learns a classifier that takes a local shape representation as input and outputs the probability that a grasp applied at this location will be successful. Given a query data point that is classified in this way, we can retrieve all the locally similar training data points and use them to predict latent global object shape. This information may help to further prune positively labeled grasp hypotheses based on, e.g. relation to the predicted average global shape or suitability for a specific task. This prediction can also guide scene exploration to prune object shape hypotheses. To learn the function that maps local shape to grasp stability we use a Random Forest Classifier. We show that our method reaches the same classification performance as the current state-of-the-art on this dataset which uses a Convolutional Neural Network. Additionally, we exploit the natural ability of the Random Forest to cluster similar data. For a positively predicted query data point, we retrieve all the locally similar training data points that are associated with the same leaf nodes of the Random Forest. The main insight from this work is that local object shape that affords a grasp is also a good predictor of global object shape. We empirically support this claim with quantitative experiments. Additionally, we demonstrate the predictive capability of the method on some real data examples.}, publisher = {IEEE}, month = may, year = {2016}, slug = {2016_icra_bks}, author = {Bohg, Jeannette and Kappler, Daniel and Schaal, Stefan}, month_numeric = {5} }