Research Overview
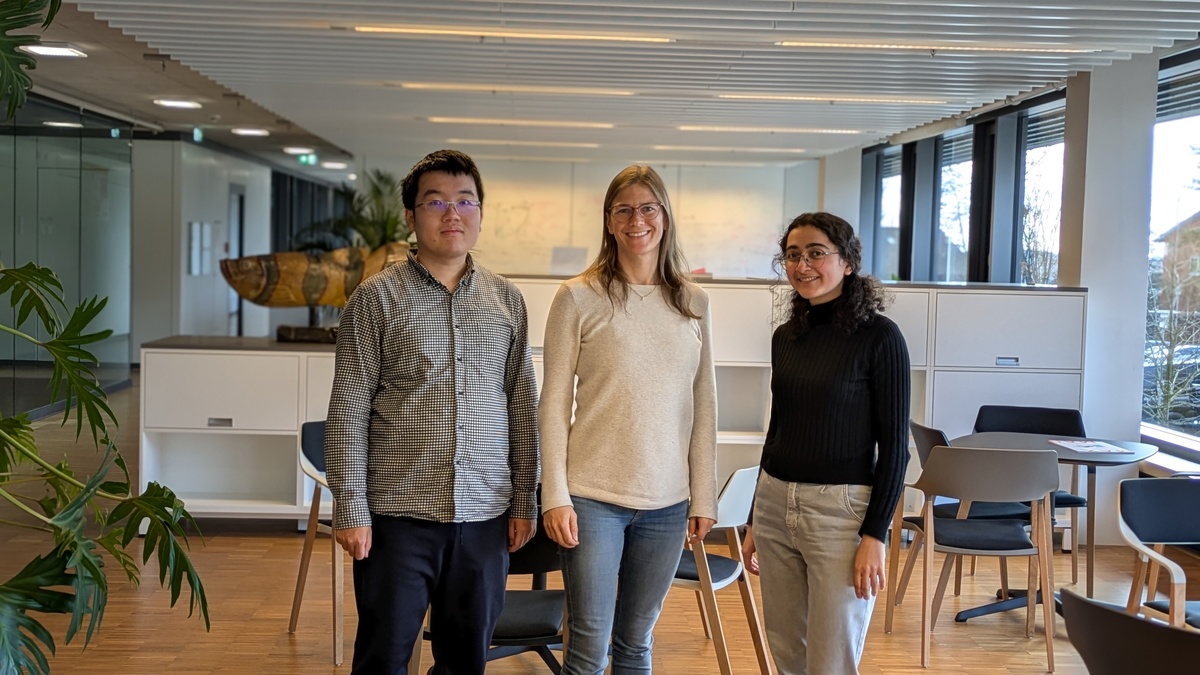
Our research revolves around machine learning with a focus on the societal embedding of technology, broadly scoped. We examine the role of AI systems in the social world and incorporate these insights into the fundamentals of how we design, study and use learning systems. Such systems range from small-scale decision-support systems to complex industry-scale machine learning applications, recommender systems, and digital platform markets.
AI systems power services, platforms, infrastructure, and markets at societal scale. But despite major breakthroughs in the field of computer science, the societal implications of the technology it develops remain a major concern. When deployed in practical applications, AI systems interact with people in ways that are often neither foreseen nor foreseeable. We observe this when algorithmic predictions drive consumption, shape preferences, induce strategic behavior and determine life outcomes. These dynamic interactions between learning systems and populations often invalidates fundamental statistical assumptions under which AI systems are designed and developed. In particular, predictive accuracy as an evaluation criterion is vastely incomplete. This can surface as negative externalites that are outside the scope of what current theories of machine learning are able to describe. Similarly, social aspects influence system behavior when economic power interplays with learning objectives and economic incentives conflict with user interests. As AI systems touch nearly every aspect of our lives, the answers to these social questions have become an integral part of what determines and will continue to determine the success of the technology we develop today.
Our vision. The primary goal of our research is to reconcile machine learning with societal values to help navigate the complex challenges that inevitably arise as the technology advances. In particular, we strive to advance our understanding of AI as part of a broader sociotechnical ecosystem and develop the technical tools and the conceptual repertoire necessary to ensure safety, reliability, equity and trustworthiness of AI systems. In this pursuit our work draws on interdisciplinary ideas from computer science, statistics, economics and social sciences. It develops concepts, frameworks and theoretical guarantees, as well as practical tools and empirical insights.