ContourCraft: Learning to Resolve Intersections in Neural Multi-Garment Simulations
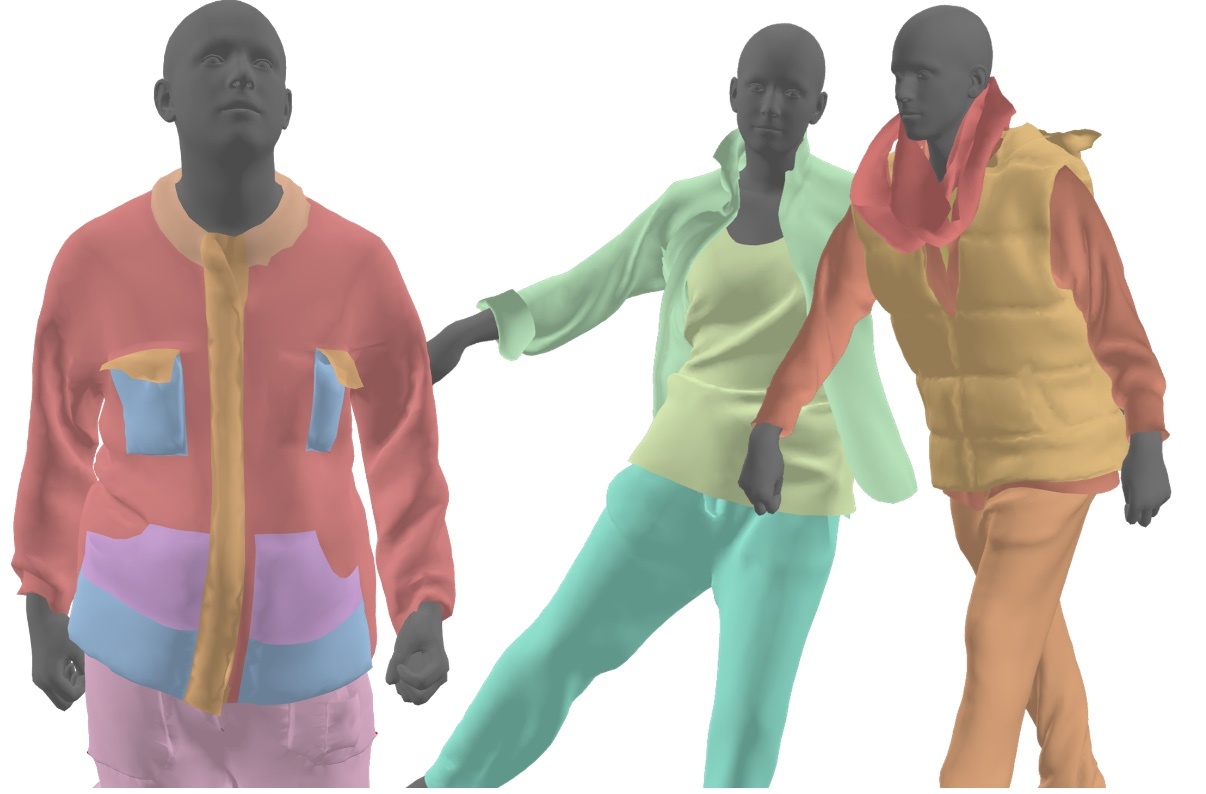
Learning-based approaches to cloth simulation have started to show their potential in recent years. However, handling collisions and intersections in neural simulations remains a largely unsolved problem. In this work, we present ContourCraft, a learning-based solution for handling intersections in neural cloth simulations. Unlike conventional approaches that critically rely on intersection-free inputs, ContourCraft robustly recovers from intersections introduced through missed collisions, self-penetrating bodies, or errors in manually designed multi-layer outfits. The technical core of ContourCraft is a novel intersection contour loss that penalizes interpenetrations and encourages rapid resolution thereof. We integrate our intersection loss with a collision-avoiding repulsion objective into a neural cloth simulation method based on graph neural networks (GNNs). We demonstrate our method’s ability across a challenging set of diverse multi-layer outfits under dynamic human motions. Our extensive analysis indicates that ContourCraft significantly improves collision handling for learned simulation and produces visually compelling results.
Author(s): | Grigorev, Artur and Becherini, Giorgio and Black, Michael and Hilliges, Otmar and Thomaszewski, Bernhard |
Book Title: | ACM SIGGRAPH 2024 Conference Papers |
Pages: | 1--10 |
Year: | 2024 |
Month: | July |
Series: | SIGGRAPH '24 |
Publisher: | Association for Computing Machinery |
Project(s): | |
Bibtex Type: | Conference Paper (inproceedings) |
Address: | New York, NY, USA |
DOI: | 10.1145/3641519.3657408 |
State: | Published |
URL: | https://dolorousrtur.github.io/contourcraft/ |
Article Number: | 81 |
Links: |
BibTex
@inproceedings{ContourCraft:2024, title = {{ContourCraft}: Learning to Resolve Intersections in Neural Multi-Garment Simulations}, booktitle = {ACM SIGGRAPH 2024 Conference Papers}, abstract = {Learning-based approaches to cloth simulation have started to show their potential in recent years. However, handling collisions and intersections in neural simulations remains a largely unsolved problem. In this work, we present ContourCraft, a learning-based solution for handling intersections in neural cloth simulations. Unlike conventional approaches that critically rely on intersection-free inputs, ContourCraft robustly recovers from intersections introduced through missed collisions, self-penetrating bodies, or errors in manually designed multi-layer outfits. The technical core of ContourCraft is a novel intersection contour loss that penalizes interpenetrations and encourages rapid resolution thereof. We integrate our intersection loss with a collision-avoiding repulsion objective into a neural cloth simulation method based on graph neural networks (GNNs). We demonstrate our method’s ability across a challenging set of diverse multi-layer outfits under dynamic human motions. Our extensive analysis indicates that ContourCraft significantly improves collision handling for learned simulation and produces visually compelling results.}, pages = {1--10}, series = {SIGGRAPH '24}, publisher = {Association for Computing Machinery}, address = {New York, NY, USA}, month = jul, year = {2024}, slug = {10-1145-3641519-3657408}, author = {Grigorev, Artur and Becherini, Giorgio and Black, Michael and Hilliges, Otmar and Thomaszewski, Bernhard}, url = {https://dolorousrtur.github.io/contourcraft/}, month_numeric = {7} }