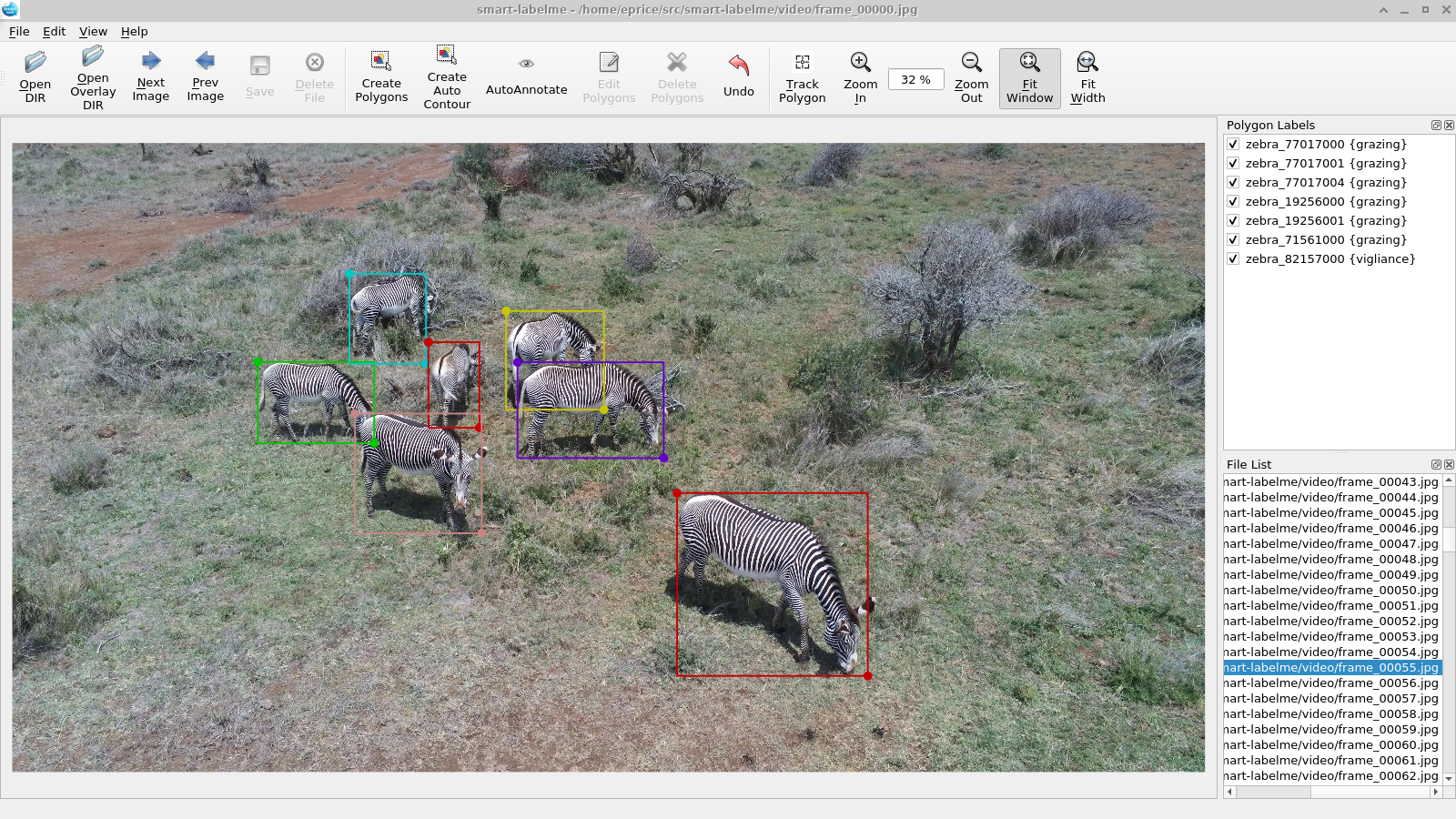
Annotating object ground truth in videos is vital for several downstream tasks in robot perception and machine learning, such as for evaluating the performance of an object tracker or training an image-based object detector. The accuracy of the annotated instances of the moving objects on every image frame in a video is crucially important. Achieving that through manual annotations is not only very time consuming and labor intensive, but is also prone to high error rate. State-of-the-art annotation methods depend on manually initializing the object bounding boxes only in the first frame and then use classical tracking methods, e.g., adaboost, or kernelized correlation filters, to keep track of those bounding boxes. These can quickly drift, thereby requiring tedious manual supervision. In this paper, we propose a new annotation method which leverages a combination of a learning-based detector (SSD) and a learning-based tracker (RE). Through this, we significantly reduce annotation drifts, and, consequently, the required manual supervision. We validate our approach through annotation experiments using our proposed annotation method and existing baselines on a set of drone video frames. Source code and detailed information on how to run the annotation program can be found at https://github.com/robot-perception-group/smarter-labelme
Author(s): | Price, Eric and Ahmad, Aamir |
Journal: | INTELLIGENT AUTONOMOUS SYSTEMS 18 |
Volume: | 2 |
Pages: | 141–153 |
Year: | 2024 |
Bibtex Type: | Article (article) |
DOI: | https://doi.org/10.1007/978-3-031-44981-9_12 |
State: | Published |
URL: | https://link.springer.com/chapter/10.1007/978-3-031-44981-9_12#citeas |
Digital: | True |
Event Name: | ICCV |
Links: |
BibTex
@article{PriceIAS_2024, title = {Accelerated Video Annotation Driven by Deep Detector and Tracker}, journal = {INTELLIGENT AUTONOMOUS SYSTEMS 18}, abstract = {Annotating object ground truth in videos is vital for several downstream tasks in robot perception and machine learning, such as for evaluating the performance of an object tracker or training an image-based object detector. The accuracy of the annotated instances of the moving objects on every image frame in a video is crucially important. Achieving that through manual annotations is not only very time consuming and labor intensive, but is also prone to high error rate. State-of-the-art annotation methods depend on manually initializing the object bounding boxes only in the first frame and then use classical tracking methods, e.g., adaboost, or kernelized correlation filters, to keep track of those bounding boxes. These can quickly drift, thereby requiring tedious manual supervision. In this paper, we propose a new annotation method which leverages a combination of a learning-based detector (SSD) and a learning-based tracker (RE). Through this, we significantly reduce annotation drifts, and, consequently, the required manual supervision. We validate our approach through annotation experiments using our proposed annotation method and existing baselines on a set of drone video frames. Source code and detailed information on how to run the annotation program can be found at https://github.com/robot-perception-group/smarter-labelme}, volume = {2}, pages = {141–153}, year = {2024}, slug = {priceias_2024}, author = {Price, Eric and Ahmad, Aamir}, url = {https://link.springer.com/chapter/10.1007/978-3-031-44981-9_12#citeas} }