Articulated motion discovery using pairs of trajectories
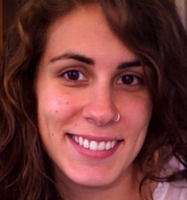
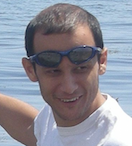
Most computer vision systems cannot take advantage of the abundance of Internet videos as training data. This is because current methods typically learn under strong supervision and require expensive manual annotations. (e.g. videos need to be temporally trimmed to cover the duration of a specific action, object bounding boxes, etc.). In this talk, I will present two techniques that can lead to learning the behavior and the structure of articulated object classes (e.g. animals) from videos, with as little human supervision as possible. First, we discover the characteristic motion patterns of an object class from videos of objects performing natural, unscripted behaviors, such as tigers in the wild. Our method generates temporal intervals that are automatically trimmed to one instance of the discovered behavior, and clusters them by type (e.g. running, turning head, drinking water). Second, we automatically recover thousands of spatiotemporal correspondences within the discovered clusters of behavior, which allow mapping pixels of an instance in one video to those of a different instance in a different video. Both techniques rely on a novel motion descriptor modeling the relative displacement of pairs of trajectories, which is more suitable for articulated objects than state-of-the-art descriptors using single trajectories. We provide extensive quantitative evaluation on our new dataset of tiger videos, which contains more than 100k fully annotated frames.