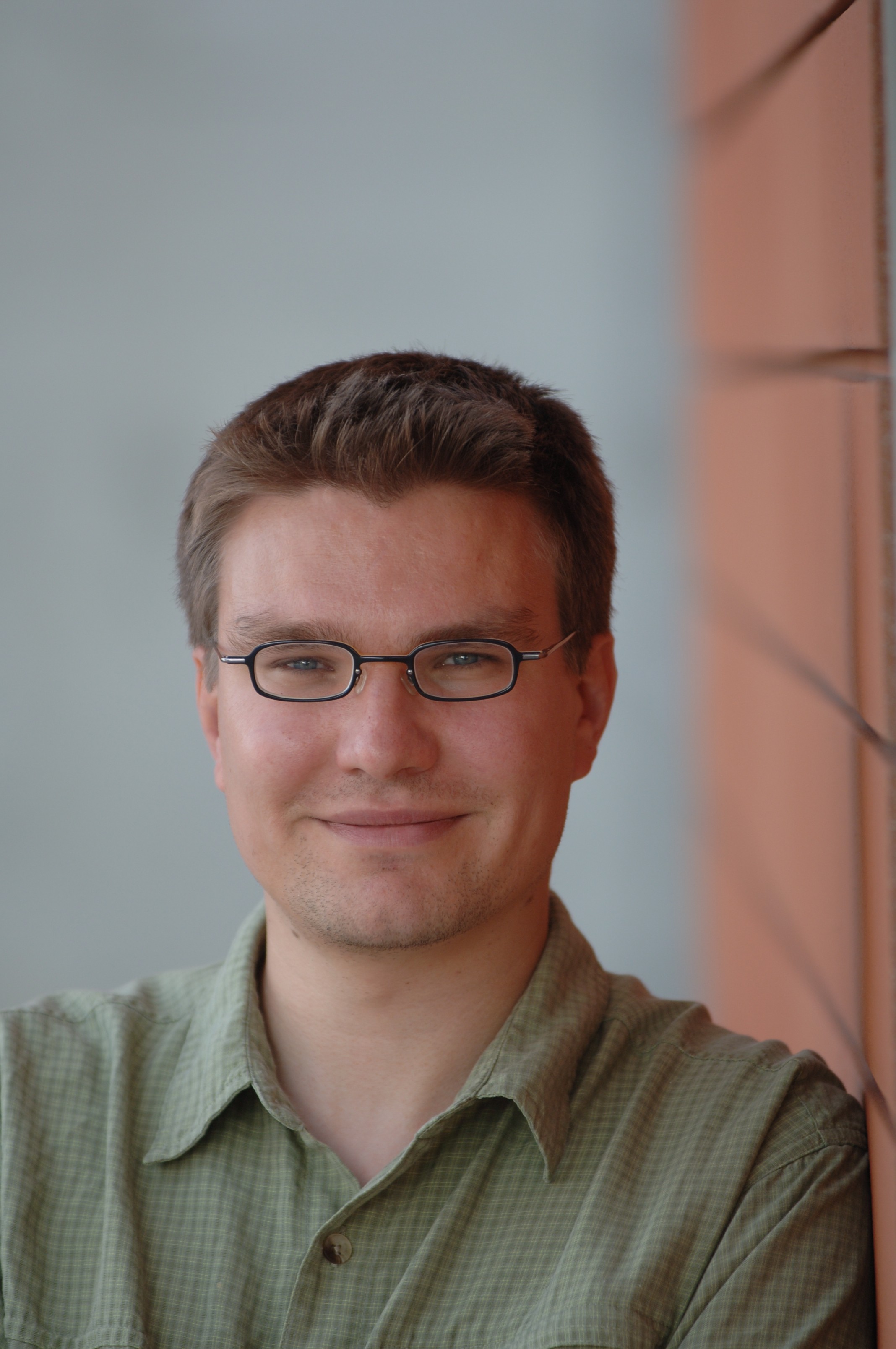
In this talk I will present two lines of research which are both applied to the problem of stereo matching. The first line of research tries to make progress on the very traditional problem of stereo matching. In BMVC 11 we presented the PatchmatchStereo work which achieves surprisingly good results with a simple energy function consisting of unary terms only. As optimization engine we used the PatchMatch method, which was designed for image editing purposes. In BMVC 12 we extended this work by adding to the energy function the standard pairwise smoothness terms. The main contribution of this work is the optimization technique, which we call PatchMatch-BeliefPropagation (PMBP). It is a special case of max-product Particle Belief Propagation, with a new sampling schema motivated by Patchmatch.
The method may be suitable for many energy minimization problems in computer vision, which have a non-convex, continuous and potentially high-dimensional label space. The second line of research combines the problem of stereo matching with the problem of object extracting in the scene. We show that both tasks can be solved jointly and boost the performance of each individual task. In particular, stereo matching improves since objects have to obey physical properties, e.g. they are not allowed to fly in the air. Object extracting improves, as expected, since we have additional information about depth in the scene.