Generative Rendering and Beyond
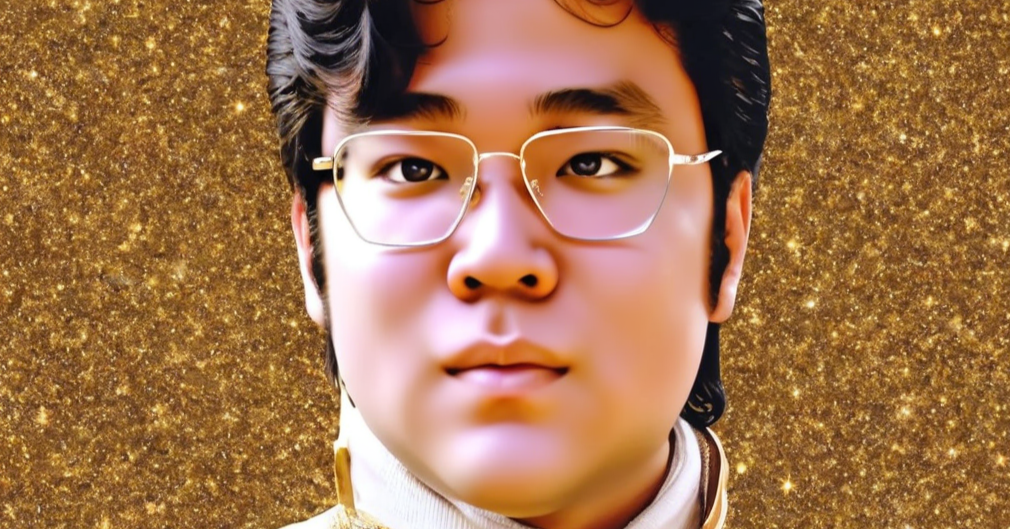
Traditional 3D content creation tools empower users to bring their imagination to life by giving them direct control over a scene's geometry, appearance, motion, and camera path. Creating computer-generated videos, however, is a tedious manual process, which can be automated by emerging text-to-video diffusion models (SORA). Despite great promise, video diffusion models are difficult to control, hindering users from applying their own creativity rather than amplifying it. In this talk, we present a novel approach called Generative Rendering that combines the controllability of dynamic 3D meshes with the expressivity and editability of emerging diffusion models. Our approach takes an animated, low-fidelity rendered mesh as input and injects the ground truth correspondence information obtained from the dynamic mesh into various stages of a pre-trained text-to-image generation model to output high-quality and temporally consistent frames. Going beyond, we will discuss the various challenges and goals towards achieving controllability in video diffusion models, and conclude with a preview of our ongoing consensus video generation efforts.
Speaker Biography
Shengqu Cai (Stanford University - Computer Science)
PhD Student
Shengqu Cai is a CS PhD student at the Stanford Computer Science and the Computational Imaging Lab, advised by Prof. Gordon Wetzstein and Prof. Leonidas Guibas. Previously, he completed his masters in CS at ETH Zürich supervised by Prof. Luc Van Gool. His research interests are in the areas of generative models, inverse rendering, unsupervised learning, and scene representations.