Long-term Temporal Convolutions for Action Recognition
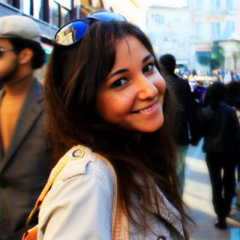
Typical human actions such as hand-shaking and drinking last several seconds and exhibit characteristic spatio-temporal structure. Recent methods attempt to capture this structure and learn action representations with convolutional neural networks. Such representations, however, are typically learned at the level of single frames or short video clips and fail to model actions at their full temporal scale. In this work we learn video representations using neural networks with long-term temporal convolutions. We demonstrate that CNN models with increased temporal extents improve the accuracy of action recognition despite reduced spatial resolution. We also study the impact of different low-level representations, such as raw values of video pixels and optical flow vector fields and demonstrate the importance of high-quality optical flow estimation for learning accurate action models. We report state-of-the-art results on two challenging benchmarks for human action recognition UCF101 and HMDB51.
Speaker Biography
Gül Varol (Department of Computer Science of École Normale Supérieure (ENS) and INRIA Paris)
PhD Student