Autonomous Robotic Manipulation
Modeling Top-Down Saliency for Visual Object Search
Interactive Perception
State Estimation and Sensor Fusion for the Control of Legged Robots
Probabilistic Object and Manipulator Tracking
Global Object Shape Reconstruction by Fusing Visual and Tactile Data
Robot Arm Pose Estimation as a Learning Problem
Learning to Grasp from Big Data
Gaussian Filtering as Variational Inference
Template-Based Learning of Model Free Grasping
Associative Skill Memories
Real-Time Perception meets Reactive Motion Generation
Autonomous Robotic Manipulation
Learning Coupling Terms of Movement Primitives
State Estimation and Sensor Fusion for the Control of Legged Robots
Inverse Optimal Control
Motion Optimization
Optimal Control for Legged Robots
Movement Representation for Reactive Behavior
Associative Skill Memories
Real-Time Perception meets Reactive Motion Generation
Interactive Perception
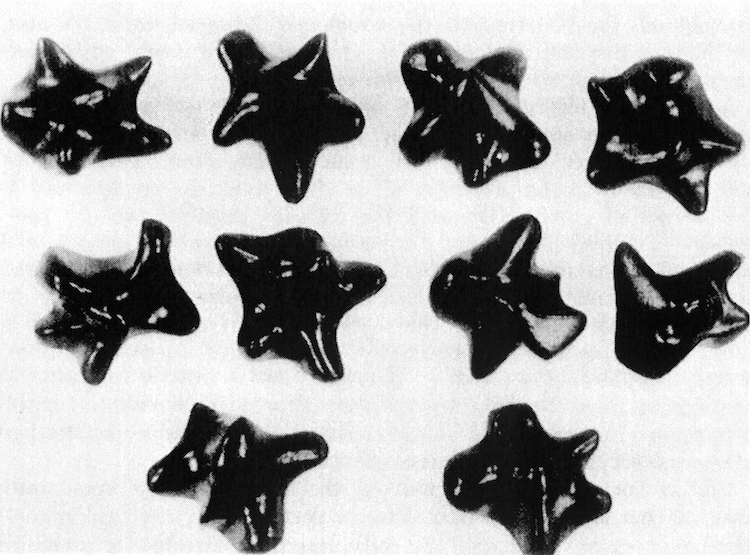
There is compelling evidence that perception in humans and animals is an active and exploratory process. For example, Gibson showed that physical interaction further augments perceptual processing beyond what can be achieved by just looking at the environment. In the specific experiment, human subjects had to find a reference object among a set of irregularly-shaped, three-dimensional objects as visualized in the above figure. They achieved an average accuracy of 49% if these objects were shown in a single image. This accuracy increased to 72% when subjects viewed rotating versions of the objects. They achieved nearly perfect performance (99%) when touching and rotating the objects in their hands. This example illustrates that biological perception and perceptually-guided behavior intrinsically rely on active exploration and knowledge of the relation between action and sensory response.
Recent approaches in robotics follow the insight that perception is facilitated by interaction with the environment. These approaches are subsumed under the term of Interactive Perception (IP). It provides the following benefits: (i) interaction with the environment creates a rich sensory signal that would otherwise not be present and (ii) knowledge of the regularity in the combined space of sensory data and action parameters facilitate the prediction and interpretation of the signal.
Members
Publications