Lost! Leveraging the Crowd for Probabilistic Visual Self-Localization
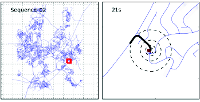
In this paper we propose an affordable solution to self- localization, which utilizes visual odometry and road maps as the only inputs. To this end, we present a probabilis- tic model as well as an efficient approximate inference al- gorithm, which is able to utilize distributed computation to meet the real-time requirements of autonomous systems. Because of the probabilistic nature of the model we are able to cope with uncertainty due to noisy visual odometry and inherent ambiguities in the map ( e.g ., in a Manhattan world). By exploiting freely available, community devel- oped maps and visual odometry measurements, we are able to localize a vehicle up to 3m after only a few seconds of driving on maps which contain more than 2,150km of driv- able roads.
Award: | (CVPR13 Best Paper Runner-Up) |
Author(s): | Marcus A. Brubaker and Andreas Geiger and Raquel Urtasun |
Book Title: | IEEE Conf. on Computer Vision and Pattern Recognition (CVPR 2013) |
Pages: | 3057-3064 |
Year: | 2013 |
Month: | June |
Publisher: | IEEE |
Bibtex Type: | Conference Paper (inproceedings) |
Address: | Portland, OR |
Award Paper: | CVPR13 Best Paper Runner-Up |
Electronic Archiving: | grant_archive |
Links: |
BibTex
@inproceedings{Brubaker2013CVPR, title = {Lost! Leveraging the Crowd for Probabilistic Visual Self-Localization}, aword_paper = {CVPR13 Best Paper Runner-Up}, booktitle = {IEEE Conf. on Computer Vision and Pattern Recognition (CVPR 2013)}, abstract = {In this paper we propose an affordable solution to self- localization, which utilizes visual odometry and road maps as the only inputs. To this end, we present a probabilis- tic model as well as an efficient approximate inference al- gorithm, which is able to utilize distributed computation to meet the real-time requirements of autonomous systems. Because of the probabilistic nature of the model we are able to cope with uncertainty due to noisy visual odometry and inherent ambiguities in the map ( e.g ., in a Manhattan world). By exploiting freely available, community devel- oped maps and visual odometry measurements, we are able to localize a vehicle up to 3m after only a few seconds of driving on maps which contain more than 2,150km of driv- able roads.}, pages = {3057-3064}, publisher = {IEEE}, address = {Portland, OR}, month = jun, year = {2013}, slug = {brubaker2013cvpr}, author = {Brubaker, Marcus A. and Geiger, Andreas and Urtasun, Raquel}, month_numeric = {6} }