D-Grasp: Physically Plausible Dynamic Grasp Synthesis for Hand-Object Interactions
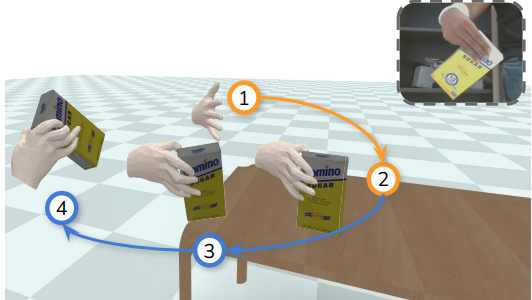
We introduce the dynamic grasp synthesis task: given an object with a known 6D pose and a grasp reference, our goal is to generate motions that move the object to a target 6D pose. This is challenging, because it requires reasoning about the complex articulation of the human hand and the intricate physical interaction with the object. We propose a novel method that frames this problem in the reinforcement learning framework and leverages a physics simulation, both to learn and to evaluate such dynamic interactions. A hierarchical approach decomposes the task into low-level grasping and high-level motion synthesis. It can be used to generate novel hand sequences that approach, grasp, and move an object to a desired location, while retaining human-likeness. We show that our approach leads to stable grasps and generates a wide range of motions. Furthermore, even imperfect labels can be corrected by our method to generate dynamic interaction sequences.
Author(s): | Christen, Sammy and Kocabas, Muhammed and Aksan, Emre and Hwangbo, Jemin and Song, Jie and Hilliges, Otmar |
Book Title: | 2022 IEEE/CVF Conference on Computer Vision and Pattern Recognition (CVPR 2022) |
Pages: | 20545--20554 |
Year: | 2022 |
Month: | June |
Publisher: | IEEE |
Bibtex Type: | Conference Paper (inproceedings) |
Address: | Piscataway, NJ |
DOI: | 10.1109/CVPR52688.2022.01992 |
Event Name: | IEEE/CVF Conference on Computer Vision and Pattern Recognition (CVPR 2022) |
Event Place: | New Orleans, Louisiana |
State: | Published |
Electronic Archiving: | grant_archive |
ISBN: | 978-1-6654-6947-0 |
Links: |
BibTex
@inproceedings{Christen:CVPR:2022, title = {{D-Grasp}: Physically Plausible Dynamic Grasp Synthesis for Hand-Object Interactions}, booktitle = {2022 IEEE/CVF Conference on Computer Vision and Pattern Recognition (CVPR 2022)}, abstract = {We introduce the dynamic grasp synthesis task: given an object with a known 6D pose and a grasp reference, our goal is to generate motions that move the object to a target 6D pose. This is challenging, because it requires reasoning about the complex articulation of the human hand and the intricate physical interaction with the object. We propose a novel method that frames this problem in the reinforcement learning framework and leverages a physics simulation, both to learn and to evaluate such dynamic interactions. A hierarchical approach decomposes the task into low-level grasping and high-level motion synthesis. It can be used to generate novel hand sequences that approach, grasp, and move an object to a desired location, while retaining human-likeness. We show that our approach leads to stable grasps and generates a wide range of motions. Furthermore, even imperfect labels can be corrected by our method to generate dynamic interaction sequences.}, pages = {20545--20554}, publisher = {IEEE}, address = {Piscataway, NJ}, month = jun, year = {2022}, slug = {christen-cvpr-2022}, author = {Christen, Sammy and Kocabas, Muhammed and Aksan, Emre and Hwangbo, Jemin and Song, Jie and Hilliges, Otmar}, month_numeric = {6} }