Social Foundations of Computation
Members
Publications
Don’t Label Twice: Quantity Beats Quality when Comparing Binary Classifiers on a Budget
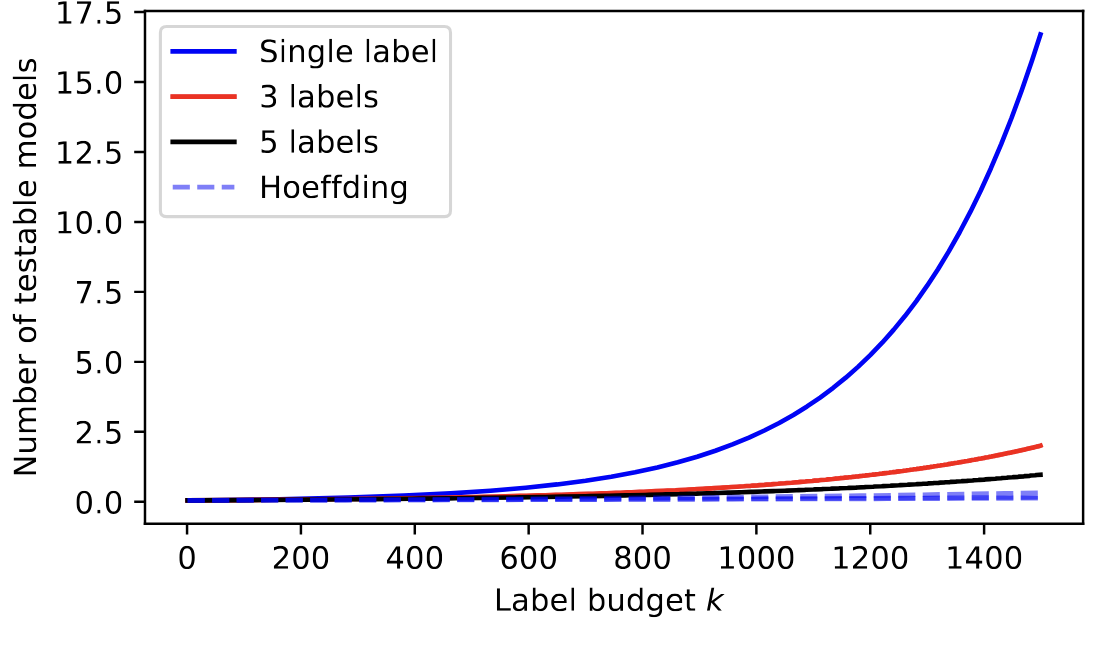
It’s widely believed that clean labels are important for benchmarking purposes. Dataset creators therefore often take a majority vote over multiple labels per data point. Contrary to conventional wisdom, we prove that when labels are costly and may contain errors, picking one label per data point works best for comparing the accuracy of two models.
Members
Publications
Social Foundations of Computation
Conference Paper
Don’t Label Twice: Quantity Beats Quality when Comparing Binary Classifiers on a Budget
Dorner, F. E., Hardt, M.
In Proceedings of the 41st International Conference on Machine Learning (ICML 2024), PMLR, The Forty-First International Conference on Machine Learning (ICML), July 2024 (Published)
ArXiv
URL
BibTeX