Autonomous Robotic Manipulation
Modeling Top-Down Saliency for Visual Object Search
Interactive Perception
State Estimation and Sensor Fusion for the Control of Legged Robots
Probabilistic Object and Manipulator Tracking
Global Object Shape Reconstruction by Fusing Visual and Tactile Data
Robot Arm Pose Estimation as a Learning Problem
Learning to Grasp from Big Data
Gaussian Filtering as Variational Inference
Template-Based Learning of Model Free Grasping
Associative Skill Memories
Real-Time Perception meets Reactive Motion Generation
Autonomous Robotic Manipulation
Learning Coupling Terms of Movement Primitives
State Estimation and Sensor Fusion for the Control of Legged Robots
Inverse Optimal Control
Motion Optimization
Optimal Control for Legged Robots
Movement Representation for Reactive Behavior
Associative Skill Memories
Real-Time Perception meets Reactive Motion Generation
AirCap: 3D Motion Capture
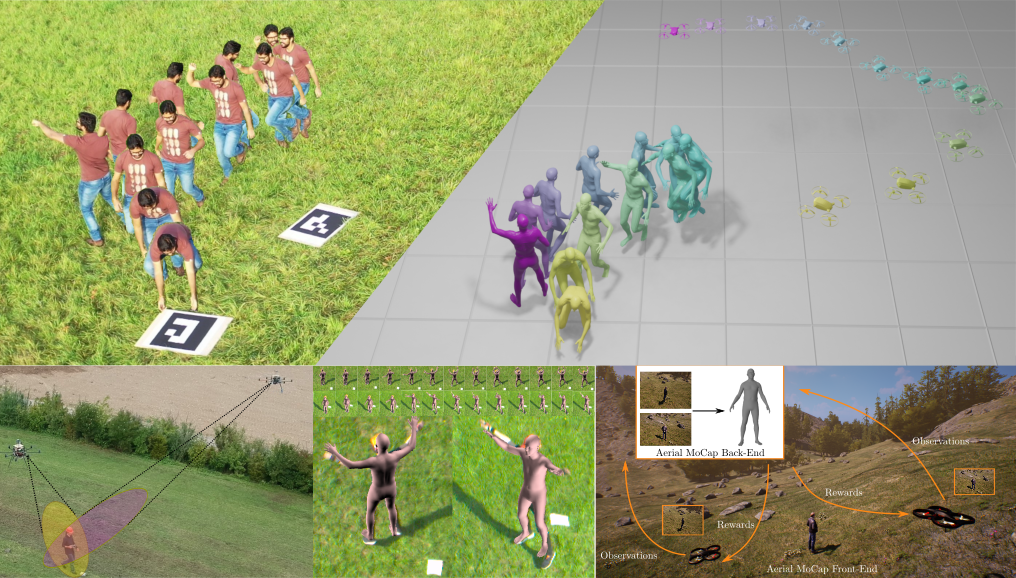
The goal of AirCap is markerless, unconstrained, human and animal motion capture (mocap) outdoors. To that end, we have developed a flying mocap system using a team of aerial vehicles (MAVs) with only on-board, monocular RGB cameras. In AirCap, mocap involves two phases: i) online data acquisition, and ii) offline pose and shape estimation.
During online data acquisition, the micro air vehicles (MAVs) detect and track the 3D position of a subject []. To do so, they perform on-board detection using a deep neural network (DNN). DNNs often fail to detect small people, which are typical in scenarios with aerial robots. By cooperatively tracking the person our system actively selects the relevant region of interest (ROI) in the images from each MAV. Then cropped high-resolution regions around the person are passed to the DNNs.
Then, human pose and shape are estimated offline using the RGB images and the MAV's self-localization (the camera extrinsics). Recent 3D human pose and shape regression methods produce noisy estimate of human pose. Our approach [] exploits multiple noisy 2D body joint detectors and noisy camera pose information. We then optimize for body shape, body pose, and camera extrinsics by fitting the SMPL body model to the 2D observations. This approach uses a strong body model to take low-level uncertainty into account and results in the first fully autonomous flying mocap system.
Offline mocap, does not enable active positioning of the MAVs to maximize the mocap accuracy. To address this, we introduce a deep reinforcement learning (RL) based multi-robot formation controller for MAVs. We formulate this problem as a sequential decision making task and solve it using an RL method [].
To enable fully on-board, online, mocap, we are developing a novel, distributed, multi-view fusion network for 3D pose and shape estimation of humans using uncalibrated moving cameras.
Members
Publications