Autonomous Robotic Manipulation
Modeling Top-Down Saliency for Visual Object Search
Interactive Perception
State Estimation and Sensor Fusion for the Control of Legged Robots
Probabilistic Object and Manipulator Tracking
Global Object Shape Reconstruction by Fusing Visual and Tactile Data
Robot Arm Pose Estimation as a Learning Problem
Learning to Grasp from Big Data
Gaussian Filtering as Variational Inference
Template-Based Learning of Model Free Grasping
Associative Skill Memories
Real-Time Perception meets Reactive Motion Generation
Autonomous Robotic Manipulation
Learning Coupling Terms of Movement Primitives
State Estimation and Sensor Fusion for the Control of Legged Robots
Inverse Optimal Control
Motion Optimization
Optimal Control for Legged Robots
Movement Representation for Reactive Behavior
Associative Skill Memories
Real-Time Perception meets Reactive Motion Generation
Movement Representation for Reactive Behavior
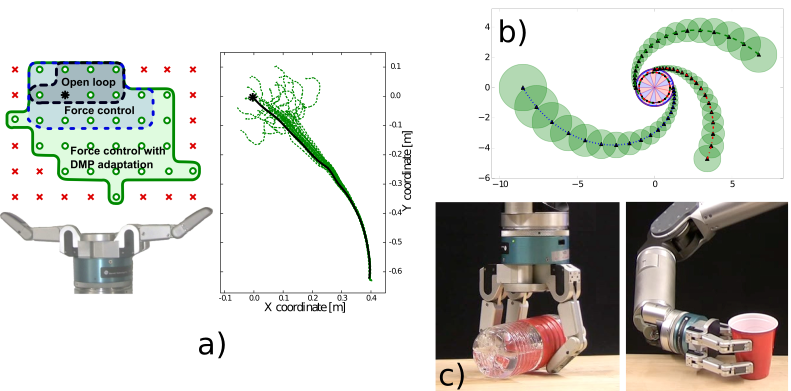
An important part of our research is concerned with the problem of movement representations. The way motion and contacts are represented is crucial to derive efficient planning and control algorithms, for example by significantly simplifying the underlying optimization problems. The way sensory information can be integrated to generate very reactive behavior is also dependent on the chosen representation. For example representing a motion as the solution of a differential equation, as it is done with Central Pattern Generators (CPGs) or Dynamic Movement Primitives (DMPs), allows one to directly include sensory feedback to the planned trajectory. Good representations are certainly not unique and different tasks and contexts require different types of representations to optimize the capabilities of the system. This becomes a crucial component when dealing with high dimensional sensory information and redundancy, like in a humanoid robot.
In our earlier work, we have explored movement representations for legged locomotion, where we used CPGs as an efficient representation to integrate sensory information into trajectory generation []. More recently, we have explored the problem of generating reactive behaviors during manipulation tasks. We have shown how the use of movement primitives in conjunction with local sensory models acquired from experience could create very reactive behaviors []. This research is connected to the the idea of associative skill memories, but looks at the problem from a feedback control point of view. We have also extended representations for rhythmic movements based on DMPs such that they can also encode transcient behaviors in limit cycle systems []. These representations are useful as they not only encode the rhythmic motion but also the "way to transition to the rhythmic motion".
Members