Autonomous Robotic Manipulation
Modeling Top-Down Saliency for Visual Object Search
Interactive Perception
State Estimation and Sensor Fusion for the Control of Legged Robots
Probabilistic Object and Manipulator Tracking
Global Object Shape Reconstruction by Fusing Visual and Tactile Data
Robot Arm Pose Estimation as a Learning Problem
Learning to Grasp from Big Data
Gaussian Filtering as Variational Inference
Template-Based Learning of Model Free Grasping
Associative Skill Memories
Real-Time Perception meets Reactive Motion Generation
Autonomous Robotic Manipulation
Learning Coupling Terms of Movement Primitives
State Estimation and Sensor Fusion for the Control of Legged Robots
Inverse Optimal Control
Motion Optimization
Optimal Control for Legged Robots
Movement Representation for Reactive Behavior
Associative Skill Memories
Real-Time Perception meets Reactive Motion Generation
Learning Coupling Terms of Movement Primitives
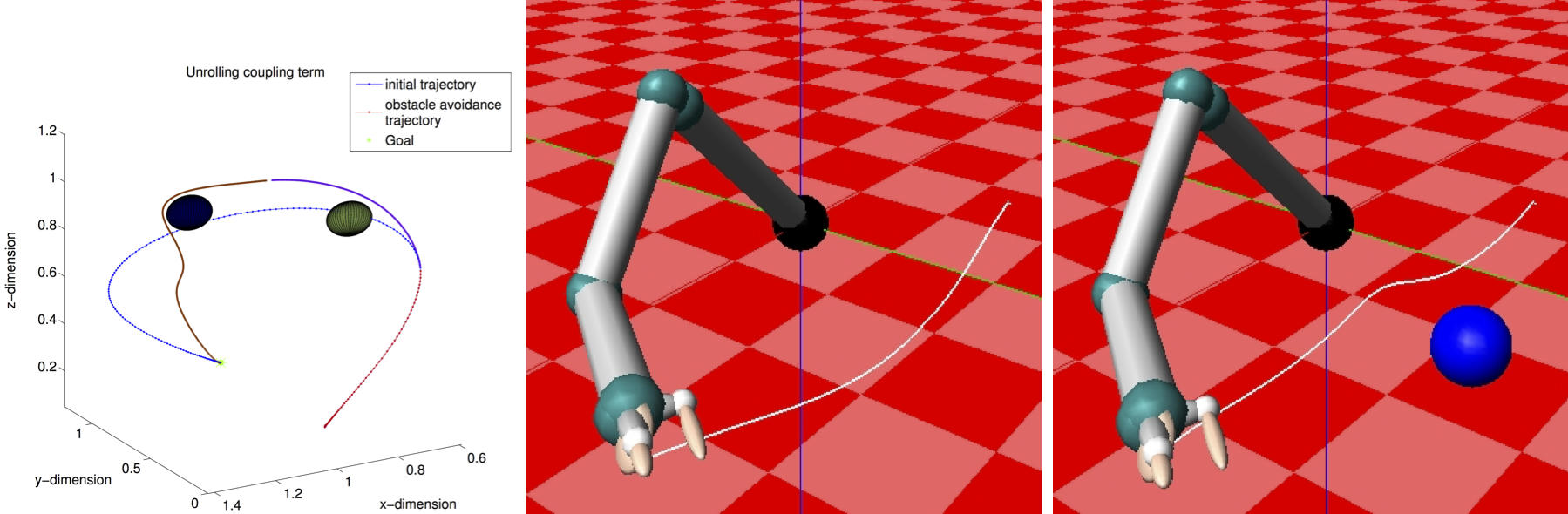
In order to perform well in day to day tasks, humanoid robots need to be able to adapt to the changes in their work environments. In response to a change in the environment, the robot can pursue at least two different strategies: First, it could re-plan, a process that is often computationally expensive and time consuming, such that rapid reactions are hard to accomplish. Alternatively, the robot could try to modify its on-going control policy with reactive strategies, which are usually very fast to compute, but suboptimal.
In this research project, we employ a reactive strategy by modifying an online control policy in the form of Dynamic Movement Primitives (DMPs) when changes in the environment occur, starting with the presence/absence of obstacles in the robot’s work environment. We envision to achieve this online modification of the movement primitive using nonlinear terms - called coupling terms - which modify the originally planned trajectory in the presence of an obstacle, and remain dormant otherwise.
Towards this goal we investigate
- How to design or learn appropriate features that can generate sufficiently powerful coupling terms for tasks like obstacle avoidance [
] .
- How to generalize the coupling term to go beyond obstacle avoidance, such that avoidance of joint-angle limits, force control on contact, bi-manual task coordination, etc., can become part of reactive feedback control.
Members
Publications