Autonomous Robotic Manipulation
Modeling Top-Down Saliency for Visual Object Search
Interactive Perception
State Estimation and Sensor Fusion for the Control of Legged Robots
Probabilistic Object and Manipulator Tracking
Global Object Shape Reconstruction by Fusing Visual and Tactile Data
Robot Arm Pose Estimation as a Learning Problem
Learning to Grasp from Big Data
Gaussian Filtering as Variational Inference
Template-Based Learning of Model Free Grasping
Associative Skill Memories
Real-Time Perception meets Reactive Motion Generation
Autonomous Robotic Manipulation
Learning Coupling Terms of Movement Primitives
State Estimation and Sensor Fusion for the Control of Legged Robots
Inverse Optimal Control
Motion Optimization
Optimal Control for Legged Robots
Movement Representation for Reactive Behavior
Associative Skill Memories
Real-Time Perception meets Reactive Motion Generation
Astronomy
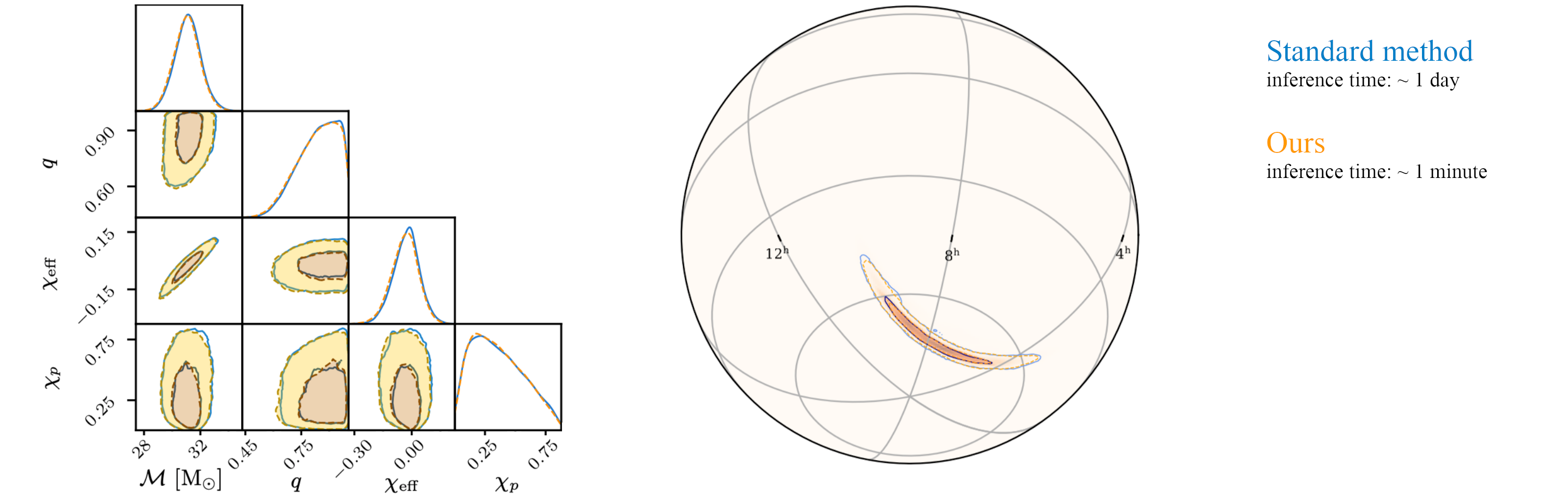
Exoplanet search is a thriving subfield of modern astrophysics, not least since the 2019 Nobel Prize in Physics recognized the first discovery of an extrasolar planet. Most of the 4500+ detections so far have used a method called transit photometry, where one observes the brightness of a star over time and searches for periodic "dips" in the light curves that occur when a planet passes in front of its host star and partly occludes it. These light curves are corrupted by systematic noise; however, since different stars are causally independent of each other (being light years apart) as well as of the instrument noise, we can denoise the signal of a single star by removing all information that the measurements of the other stars can explain. Our method, called half-sibling regression (HSR) [], has led to our discovery of 21 planets [
], including the celebrated K2-18b, the first exoplanet in the habitable zone where water vapor was detected in the atmosphere.
More recently, we have extended the HSR framework to direct imaging, an observation technique different from transit photometry, allowing studying the properties of a planet (e.g., atmospheric composition) in greater detail. Our method [] is the first post-processing algorithm for high-contrast imaging data that can also incorporate the observing conditions of an observation into the denoising process, once again demonstrating the flexibility of the HSR method.
Gravitational wave science The Nobel Prize-winning detection of gravitational waves (GW) from a binary black hole merger in 2015 was a milestone in physics. However, despite the unprecedented sensitivity of the LIGO detectors, GW detection and analysis remain challenging. Working with the MPI in Potsdam, we have developed an efficient dilated convolutional neural net to identify GW signals from black hole mergers in measurements from the LIGO detectors []. Many recent applications of ML to GW detection build upon this work.
GWs encode properties of their astrophysical generators. We have developed an approach to infer such information in real time by training normalizing flows to represent the Bayesian posterior over black hole parameters []. Our method reduces inference times from days to a minute per event without sacrificing accuracy. It is further more flexible than conventional methods, and could thereby enable model free treatment of detector noise and routine use of more physically realistic waveform models. We thus expect it to become a leading approach to gravitational-wave parameter inference.
Other Our work on predictive control for periodic error correction [] has been incorporated into PHD2 Guiding, a widely used autoguiding software in the astrophotography community.
Members
Publications