Autonomous Robotic Manipulation
Modeling Top-Down Saliency for Visual Object Search
Interactive Perception
State Estimation and Sensor Fusion for the Control of Legged Robots
Probabilistic Object and Manipulator Tracking
Global Object Shape Reconstruction by Fusing Visual and Tactile Data
Robot Arm Pose Estimation as a Learning Problem
Learning to Grasp from Big Data
Gaussian Filtering as Variational Inference
Template-Based Learning of Model Free Grasping
Associative Skill Memories
Real-Time Perception meets Reactive Motion Generation
Autonomous Robotic Manipulation
Learning Coupling Terms of Movement Primitives
State Estimation and Sensor Fusion for the Control of Legged Robots
Inverse Optimal Control
Motion Optimization
Optimal Control for Legged Robots
Movement Representation for Reactive Behavior
Associative Skill Memories
Real-Time Perception meets Reactive Motion Generation
Bodies from RGB-D
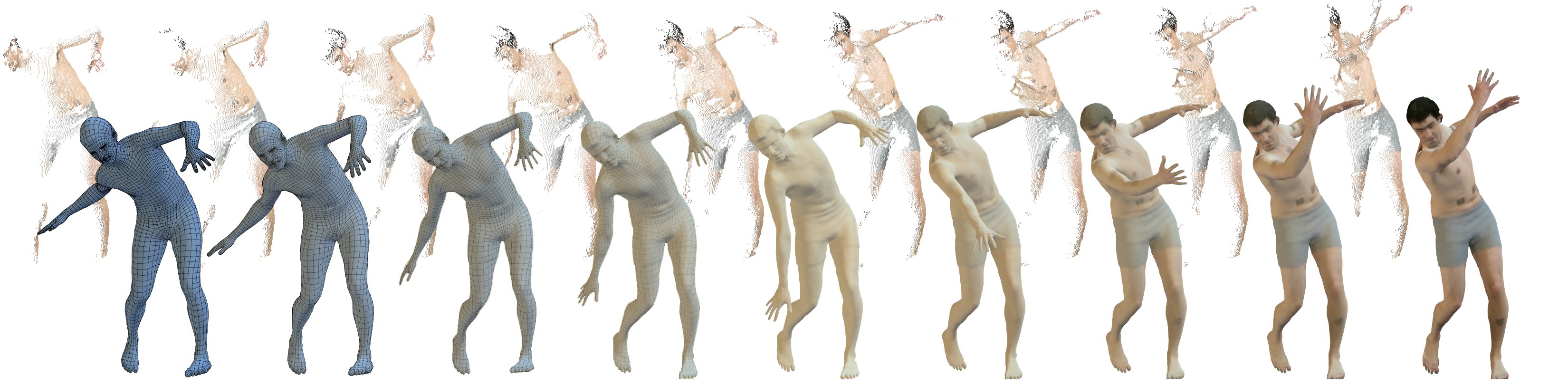
Accurate 3D body shape and appearance capture is useful for applications ranging from special effects, to fashion, to medicine. High-resolution scanners can capture human body shape and texture in great detail but these are bulky and expensive. In contrast, inexpensive RGB-D sensors are proliferating but are of much lower resolution. Scanning a full body from multiple partial views requires that the subject stands still or that the system precisely registers deforming point clouds captured from a non-rigid and articulated body.
We developed the first method to estimate human body shape from Kinect data []. The approach fits a body model to depth and image silhouettes to estimate body shape and pose from static scans of a subject in one or more static poses. We have since improved this greatly and our latest method estimates body shape with the realism of a high-resolution body scanner by allowing a user to move freely in front of a single commodity RGB-D sensor [
]
To achieve this, we develop a new parametric 3D body model, Delta, that is based on SCAPE but contains several important innovations. First, we define a parametric shape model at multiple resolutions that enables the estimation of body shape and pose in a coarse-to-fine process. Second, we define a variable-detail shape model that models facial shape with higher detail than body shape; this is important for realistic avatars. Third, we combine a relatively-low polygon count mesh with a high-resolution displacement map to capture realistic shape details, and a high-resolution texture map estimated from the sequence.
We bring color and range data in each frame into alignment with our body model adopting a coarse-to-fine approach. The method exploits geometry and image texture over time to obtain accurate shape, pose, and appearance information despite unconstrained motion, partial views, varying resolution, occlusion, and soft tissue deformation.
Our recovered models are competitive with high-resolution scans from a professional 3D scanning system. Our system creates accurate 3D avatars from challenging motion sequences and even captures soft tissue dynamics.
Members
Publications