Autonomous Robotic Manipulation
Modeling Top-Down Saliency for Visual Object Search
Interactive Perception
State Estimation and Sensor Fusion for the Control of Legged Robots
Probabilistic Object and Manipulator Tracking
Global Object Shape Reconstruction by Fusing Visual and Tactile Data
Robot Arm Pose Estimation as a Learning Problem
Learning to Grasp from Big Data
Gaussian Filtering as Variational Inference
Template-Based Learning of Model Free Grasping
Associative Skill Memories
Real-Time Perception meets Reactive Motion Generation
Autonomous Robotic Manipulation
Learning Coupling Terms of Movement Primitives
State Estimation and Sensor Fusion for the Control of Legged Robots
Inverse Optimal Control
Motion Optimization
Optimal Control for Legged Robots
Movement Representation for Reactive Behavior
Associative Skill Memories
Real-Time Perception meets Reactive Motion Generation
Causal Reasoning in RL
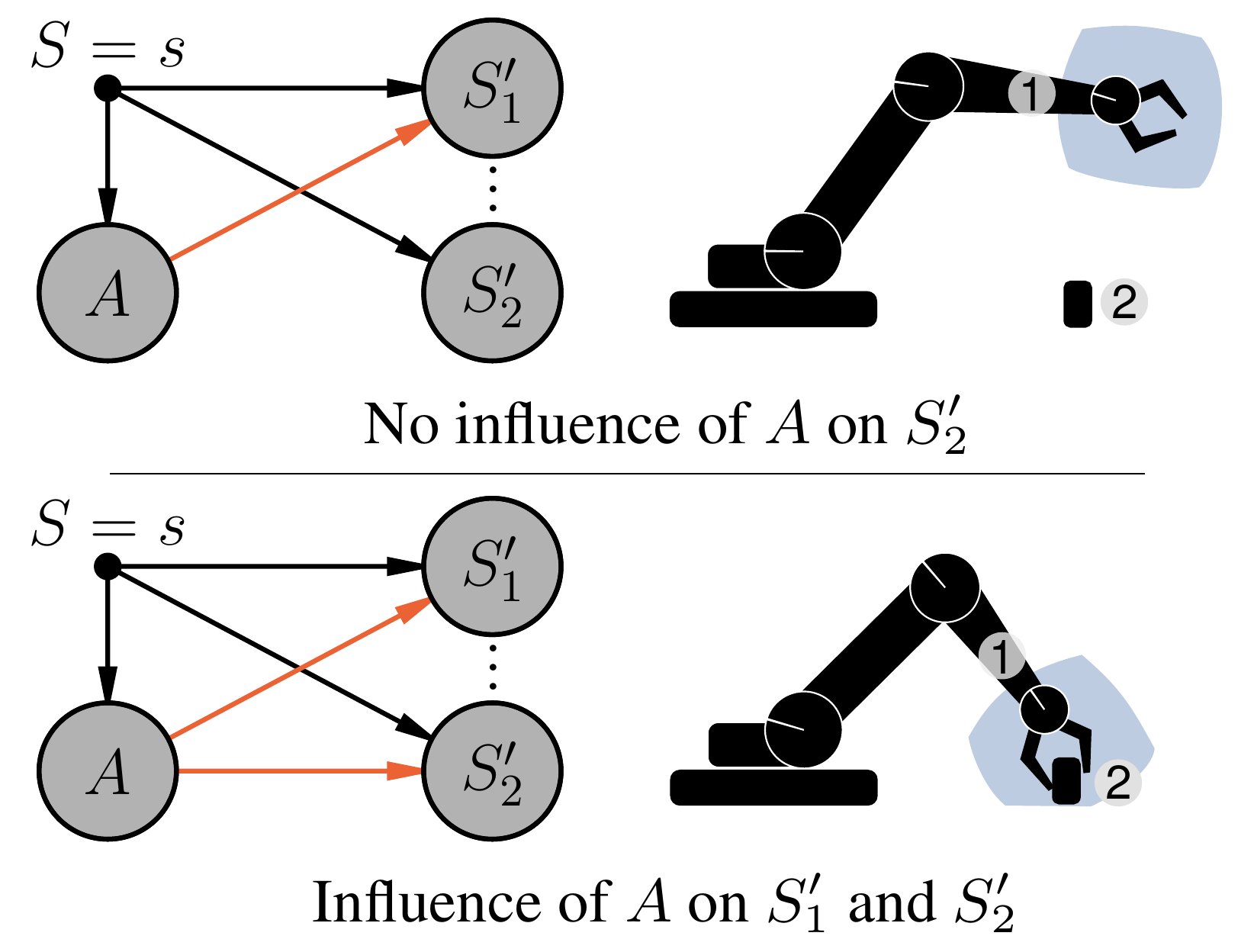
Reinforcement learning is fundamentally a causal endeavor. The agent intervenes in the environment through actions and observes their effects; learning through credit assignment involves the counterfactual question whether another action would have resulted in a better outcome. Causal Reasoning for RL is about learning and using causal knowledge to improve RL algorithms. We are interested in using tools from causality to make RL algorithms more robust and sample efficient.
In a first project [
, we showed how learning a causal model of agent-object interactions allows to infer whether the agent has causal influence on its environment. We then demonstrated how this information can be integrated into RL algorithms to steer the exploration process and improve sample-efficiency of off-policy training. Experiments on robotic manipulation benchmarks show a clear improvement over state-of-the-art.]
Members
Publications