Deep, Probabilistic and Semantic 3D Reconstruction
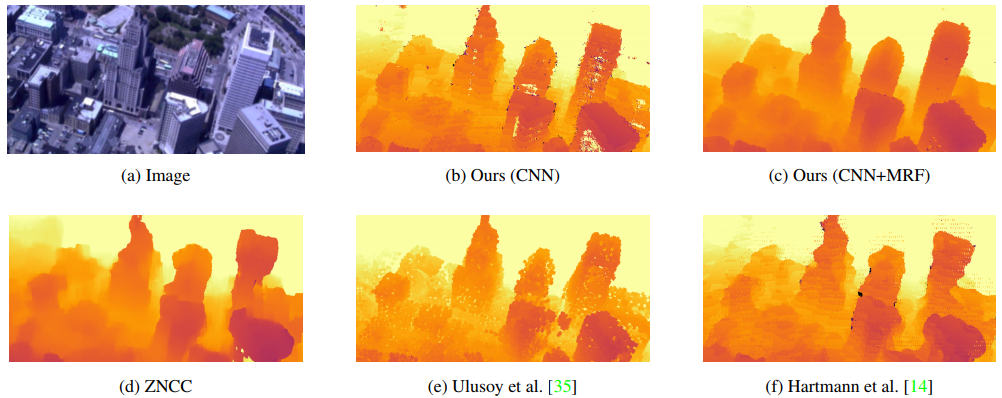
Convolutional networks working on images are often agnostic of the image formation process such as perspective geometry and occlusion; it is often only of marginal interest. However, classical approaches to 3D reconstruction benefit greatly from explicit knowledge about 3D geometry and light propagation, as we have also shown [][
].
With RayNet [], we have presented the first approach that integrates this knowledge into a deep 3D reconstruction model by unrolling a high-order CRF as layers in a convolutional network, combining the strengths of both frameworks.
Furthermore, knowledge about the types of objects being reconstructed constrains the possible set of outcomes. For this reason, we have leveraged the combination of semantic classification in the context of 3D reconstruction. In an initial phase, we have shown the benefit for classical techniques []. In the same spirit as RayNet, we have subsequently leveraged the power of deep learning to perform the semantic 3D reconstruction jointly [
].
Members
Publications