Geometric Image Synthesis
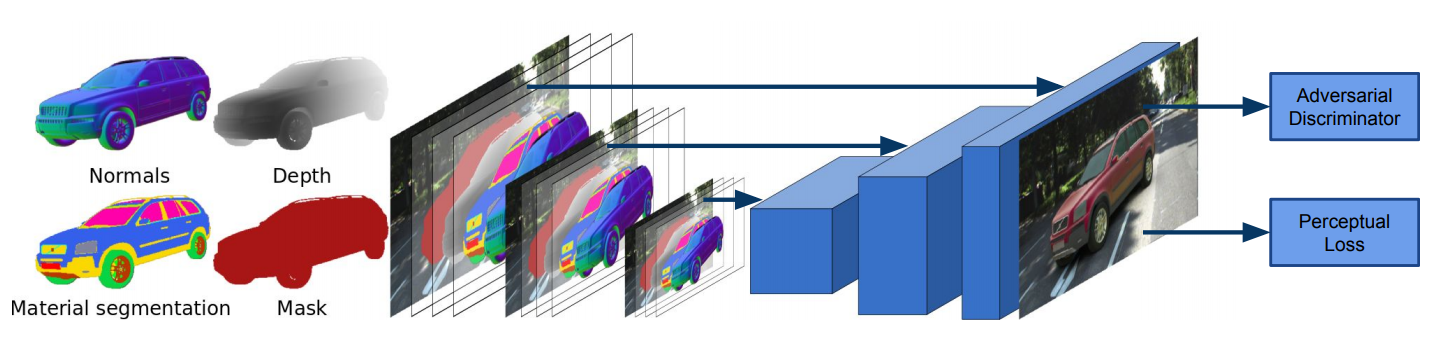
We have leveraged our insights on training generative models for tackling image synthesis tasks, with the ultimate goal of generating large amounts of training data with limited cost. We first demonstrate that data augmentation is a viable alternative to annotating real images or synthesizing entire scenes from scratch [][
]. Towards this goal, we augmented real images from the KITTI dataset with photo-realistically rendered 3D car models, yielding significant performance improvements when used for training deep neural networks on recognition tasks.
In [], we demonstrate that the rendering process itself can be learned. In particular, we used the output of OpenGL (depth, normals, materials) as input to a neural network which generates a rendering of the respective real-world object. Importantly, our generative model handles ambiguity in the ouptut (e.g., cars might have a different color) and learns to add realistic transparency, reflection and shadowing effects to the augmented objects.
Publications