Reinforcement Learning and Control
Model-based Reinforcement Learning and Planning
Object-centric Self-supervised Reinforcement Learning
Self-exploration of Behavior
Causal Reasoning in RL
Equation Learner for Extrapolation and Control
Intrinsically Motivated Hierarchical Learner
Regularity as Intrinsic Reward for Free Play
Curious Exploration via Structured World Models Yields Zero-Shot Object Manipulation
Natural and Robust Walking from Generic Rewards
Goal-conditioned Offline Planning
Offline Diversity Under Imitation Constraints
Learning Diverse Skills for Local Navigation
Learning Agile Skills via Adversarial Imitation of Rough Partial Demonstrations
Combinatorial Optimization as a Layer / Blackbox Differentiation
Object-centric Self-supervised Reinforcement Learning
Symbolic Regression and Equation Learning
Representation Learning
Stepsize adaptation for stochastic optimization
Probabilistic Neural Networks
Learning with 3D rotations: A hitchhiker’s guide to SO(3)
MOVER – Reconstructing 3D Scenes and People using Interaction
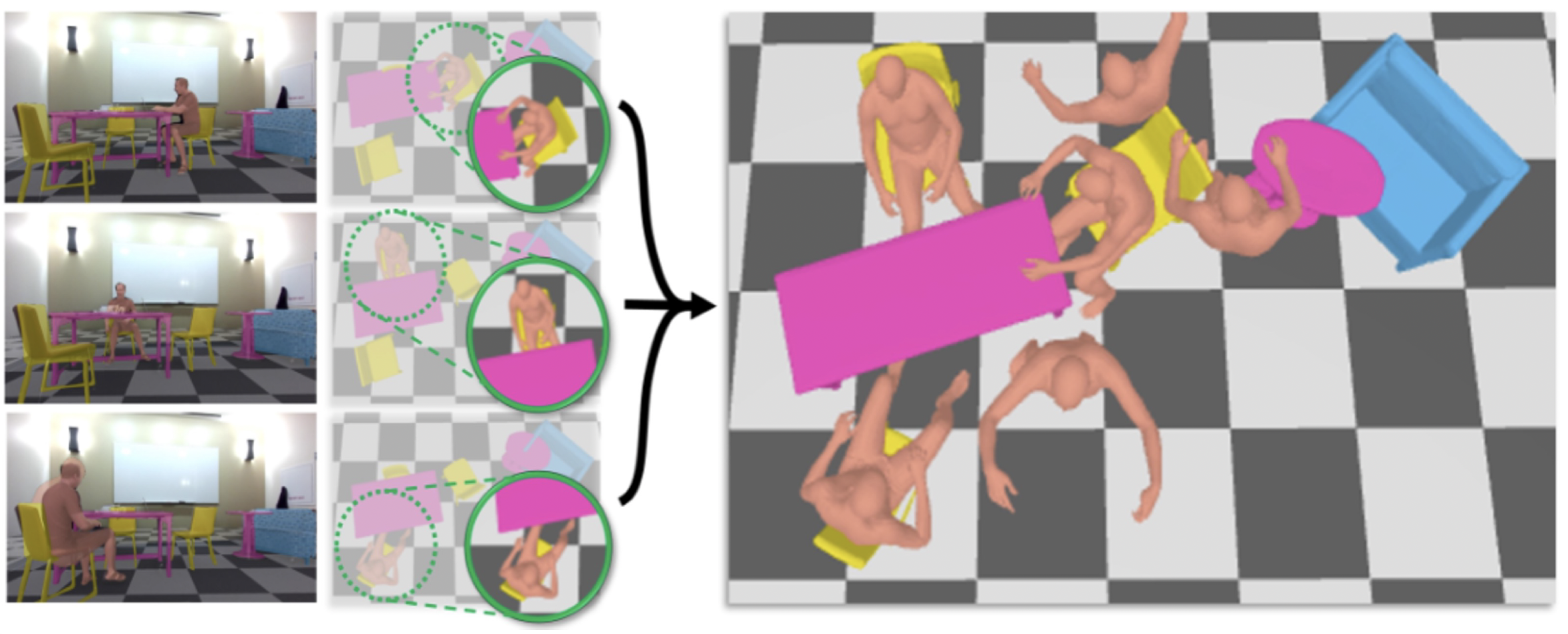
Humans are in constant contact with the world as they move through it and interact with it. This contact is a vital source of information for understanding 3D humans, 3D scenes, and the interactions between them. In fact, we demonstrate that these human-scene interactions (HSIs) can be leveraged to improve the 3D reconstruction of a scene from a monocular RGB video. Our key idea is that, as a person moves through a scene and interacts with it, we accumulate HSIs across multiple input images, and optimize the 3D scene to reconstruct a consistent, physically plausible and functional 3D scene layout. Our optimization-based approach exploits three types of HSI constraints: (1) humans that move in a scene are occluded or occlude objects, thus, defining the depth ordering of the objects, (2) humans move through free space and do not interpenetrate objects, (3) when humans and objects are in contact, the contact surfaces occupy the same place in space. Using these constraints in an optimization formulation across all observations, we significantly improve the 3D scene layout reconstruction. Furthermore, we show that our scene reconstruction can be used to refine the initial 3D human pose and shape (HPS) estimation. We evaluate the 3D scene layout reconstruction and HPS estimation qualitatively and quantitatively using the PROX and PiGraphs datasets. The code and data are available for research purposes at https://mover.is.tue.mpg.de/.
Members
Publications