Reinforcement Learning and Control
Model-based Reinforcement Learning and Planning
Object-centric Self-supervised Reinforcement Learning
Self-exploration of Behavior
Causal Reasoning in RL
Equation Learner for Extrapolation and Control
Intrinsically Motivated Hierarchical Learner
Regularity as Intrinsic Reward for Free Play
Curious Exploration via Structured World Models Yields Zero-Shot Object Manipulation
Natural and Robust Walking from Generic Rewards
Goal-conditioned Offline Planning
Offline Diversity Under Imitation Constraints
Learning Diverse Skills for Local Navigation
Learning Agile Skills via Adversarial Imitation of Rough Partial Demonstrations
Combinatorial Optimization as a Layer / Blackbox Differentiation
Object-centric Self-supervised Reinforcement Learning
Symbolic Regression and Equation Learning
Representation Learning
Stepsize adaptation for stochastic optimization
Probabilistic Neural Networks
Learning with 3D rotations: A hitchhiker’s guide to SO(3)
Reinforcement Learning for Diverse Solutions
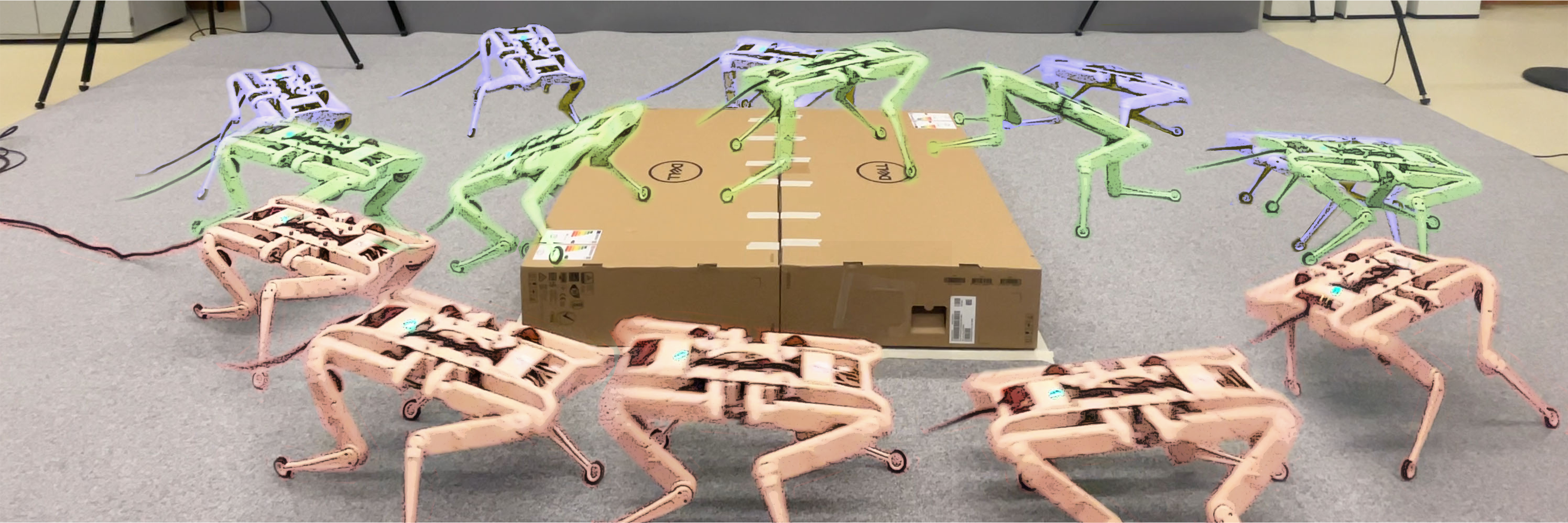
Learning diverse and useful skills is one of the major challenges in robotics. Imitation learning from state-only demonstrations is a promising approach to capture preferences and to easily specify tasks. We first study how to obtain agile behavior from only partial demonstrations []. We develop a Wasserstein discrimination method to compute the reward. This paper was one of the three best paper finalist at CoRL 2022.
To obtain diverse behaviors online, we developed an algorithm [] that learns versatile and controllable skills based on mutual information and information gain as diversity objective. Similar to our earlier work, it combines a GAIL-like imitation reward with task-specific rewards. The subsequent work [
] enhances diversity using a physics-inspired Van der Waals (VdW) force objective and encodes utilities as multiple constraints.
We then studied the offline setting, i.e., when only data from previous interactions are available and no further environmental interaction is possible. In [] we propose the first principled offline method for maximizing skill diversity under imitation constraints. The method requires access to an explorative dataset of the environment and a few demonstrations of solving a task. Here, we focus on the challenging and practically relevant setting where the demonstrations are provided only in terms of visited states, i.e., without actions. To generate diversity, based on an information-theoretic objective, the method trains a skill discriminator. The follow-up work [
], eliminates the need to learn a skill discriminator by providing an offline estimator of the VdW distance. We also use Functional Reward Encoding to better handle non-stationary rewards, allowing for stable, efficient training and zero-shot recall of all skills encountered during training.