Reinforcement Learning and Control
Model-based Reinforcement Learning and Planning
Object-centric Self-supervised Reinforcement Learning
Self-exploration of Behavior
Causal Reasoning in RL
Equation Learner for Extrapolation and Control
Intrinsically Motivated Hierarchical Learner
Regularity as Intrinsic Reward for Free Play
Curious Exploration via Structured World Models Yields Zero-Shot Object Manipulation
Natural and Robust Walking from Generic Rewards
Goal-conditioned Offline Planning
Offline Diversity Under Imitation Constraints
Learning Diverse Skills for Local Navigation
Learning Agile Skills via Adversarial Imitation of Rough Partial Demonstrations
Combinatorial Optimization as a Layer / Blackbox Differentiation
Object-centric Self-supervised Reinforcement Learning
Symbolic Regression and Equation Learning
Representation Learning
Stepsize adaptation for stochastic optimization
Probabilistic Neural Networks
Learning with 3D rotations: A hitchhiker’s guide to SO(3)
AirCap: 3D Motion Capture
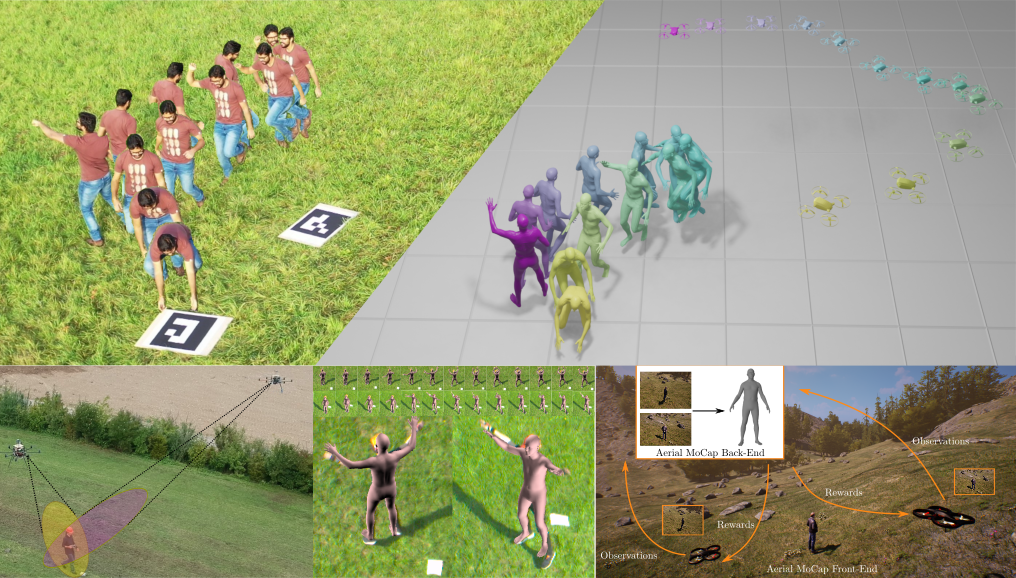
The goal of AirCap is markerless, unconstrained, human and animal motion capture (mocap) outdoors. To that end, we have developed a flying mocap system using a team of aerial vehicles (MAVs) with only on-board, monocular RGB cameras. In AirCap, mocap involves two phases: i) online data acquisition, and ii) offline pose and shape estimation.
During online data acquisition, the micro air vehicles (MAVs) detect and track the 3D position of a subject []. To do so, they perform on-board detection using a deep neural network (DNN). DNNs often fail to detect small people, which are typical in scenarios with aerial robots. By cooperatively tracking the person our system actively selects the relevant region of interest (ROI) in the images from each MAV. Then cropped high-resolution regions around the person are passed to the DNNs.
Then, human pose and shape are estimated offline using the RGB images and the MAV's self-localization (the camera extrinsics). Recent 3D human pose and shape regression methods produce noisy estimate of human pose. Our approach [] exploits multiple noisy 2D body joint detectors and noisy camera pose information. We then optimize for body shape, body pose, and camera extrinsics by fitting the SMPL body model to the 2D observations. This approach uses a strong body model to take low-level uncertainty into account and results in the first fully autonomous flying mocap system.
Offline mocap, does not enable active positioning of the MAVs to maximize the mocap accuracy. To address this, we introduce a deep reinforcement learning (RL) based multi-robot formation controller for MAVs. We formulate this problem as a sequential decision making task and solve it using an RL method [].
To enable fully on-board, online, mocap, we are developing a novel, distributed, multi-view fusion network for 3D pose and shape estimation of humans using uncalibrated moving cameras.
Members
Publications