Enhanced Flexible Mold Lifetime for Roll‐to‐Roll Scaled‐Up Manufacturing of Adhesive Complex Microstructures
Bioinspired Microstructured Adhesives with Facile and Fast Switchability for Part Manipulation in Dry and Wet Conditions
Smart Materials for manipulation and actuation of small-scale structures
3D nanofabrication of various materials for advanced multifunctional microrobots
Liquid Crystal Mesophase of Supercooled Liquid Gallium And Eutectic Gallium–Indium
Machine Learning-Based Pull-off and Shear Optimal Adhesive Microstructures
Information entropy to detect order in self-organizing systems
Individual and collective manipulation of multifunctional bimodal droplets in three dimensions
Microrobot collectives with reconfigurable morphologies and functions
Self-organization in heterogeneous and non-reciprocal regime
Biomimetic Emulsion Systems
Giant Unilamellar Vesicles for Designing Cell-like Microrobots
Bioinspired self-assembled colloidal collectives drifting in three dimensions underwater
Data-driven design of shape-programmable magnetic soft materials
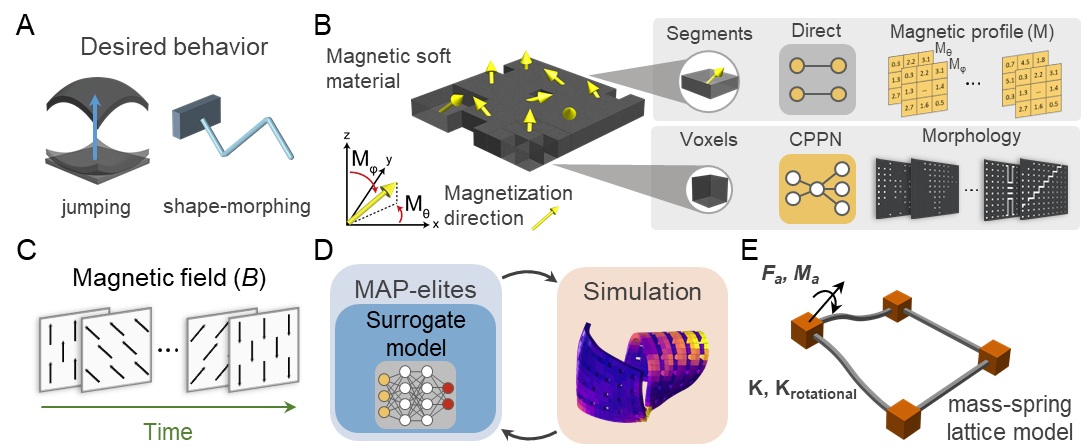
Magnetically responsive soft materials with spatially encoded magnetic and material properties enable unprecedented shape-morphing capabilities with a plethora of applications, ranging from soft medical robots to bio-interfaces. Despite the vast design space enabled by the high-resolution encoding of three-dimensional (3D) magnetic and material properties, their coupled nature renders exploration of such large design spaces with current trial and error-based approaches infeasible. Here, we introduce a data-driven design strategy to search the design space of distributed spatial magnetization profile and morphology for desired shape-morphing and behavior of magnetic soft materials. The developed approach explores the design space relying on stochastic design alterations while guided by a predictive neural network and the promising candidates are evaluated in a cost-efficient simulation environment. Using our data-driven strategy, we demonstrate non-intuitive designs for two-dimensional (2D) magnetic soft structures, which can morph into complex 2D/3D structures inconceivable previously. The data-driven approach further enables design optimization for desired morphological behaviors, including maximizing rotation around the longitudinal axis and minimizing the bounding volume of soft structures. The superior performance of data-driven strategy-generated designs is showcased in comparison to an intuitive design adapted from the literature for soft robot jumping behavior. Fabrication, programming, and scale agnostic nature of the data-driven strategy is further highlighted by the design of inherently 3D multi-material soft magnetic structures for robotic behaviors of traversing and jumping, as well as configurability of these behaviors through additional stimuli. Overall, the introduced data-driven approach establishes a generic framework for efficient exploration of the design space for stimuli-responsive soft materials, enabling functional shape-morphing and behaviors, thus paving the way for next-generation soft robots and devices.
Members