Reinforcement Learning and Control
Model-based Reinforcement Learning and Planning
Object-centric Self-supervised Reinforcement Learning
Self-exploration of Behavior
Causal Reasoning in RL
Equation Learner for Extrapolation and Control
Intrinsically Motivated Hierarchical Learner
Regularity as Intrinsic Reward for Free Play
Curious Exploration via Structured World Models Yields Zero-Shot Object Manipulation
Natural and Robust Walking from Generic Rewards
Goal-conditioned Offline Planning
Offline Diversity Under Imitation Constraints
Learning Diverse Skills for Local Navigation
Learning Agile Skills via Adversarial Imitation of Rough Partial Demonstrations
Combinatorial Optimization as a Layer / Blackbox Differentiation
Object-centric Self-supervised Reinforcement Learning
Symbolic Regression and Equation Learning
Representation Learning
Stepsize adaptation for stochastic optimization
Probabilistic Neural Networks
Learning with 3D rotations: A hitchhiker’s guide to SO(3)
Beyond Motion Capture
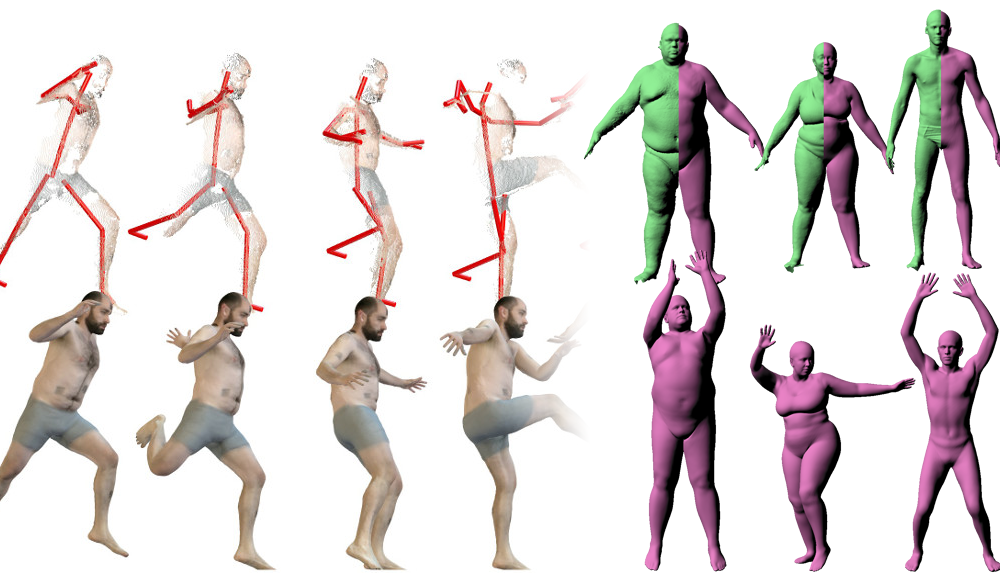
Accurately capturing human body shape and motion is important for many applications in computer vision and graphics. Traditional motion capture (mocap) focuses on extracting a skeleton from a sparse set of markers. Our work pushes the boundaries of motion capture to use new sensors and to extract richer information about body shape and human movement.
Traditional mocap uses a set of sparse markers placed on the body to estimate skeleton motion. These markers are typically places on parts of the body that move rigidly to try to minimize the effects of soft tissue motion. In this process nuanced information about surface motion is lost and animations using mocap often feel lifeless or eerie. MoSh (Motion and Shape capture) [] addresses this problem by directly estimating a 3D parametric body model from 3D markers. Given a standard marker set, MoSh simultaneously estimates the marker locations on the 3D model and recovers body shape and pose. By allowing body shape to vary over time, MoSh can also capture the non-rigid motion of soft tissue. From a small set of markers MoSh is able to recover a remarkably accurate 3D model of the body. The motions can then be retargetting to new characters, resulting in realistic, lifelike, animations.
In comparison with mocap, consumer RGB-D devices provide denser observations of the body, but these scans are incomplete (taken from a single view) and noisy. By using RGB-D sequences of bodies in motion, we can extract more detailed information about body shape and motion []. To do so, we introduce a multi-resolution body model and exploit time continuity of human motion and RGB appearance to estimate accurate body shape, pose and appearance. The approach can track arbitrary challenging motions, and extracts highly realistic 3D textured avatars with an accuracy rivaling high-cost laser scanners.
Members
Publications