Reinforcement Learning and Control
Model-based Reinforcement Learning and Planning
Object-centric Self-supervised Reinforcement Learning
Self-exploration of Behavior
Causal Reasoning in RL
Equation Learner for Extrapolation and Control
Intrinsically Motivated Hierarchical Learner
Regularity as Intrinsic Reward for Free Play
Curious Exploration via Structured World Models Yields Zero-Shot Object Manipulation
Natural and Robust Walking from Generic Rewards
Goal-conditioned Offline Planning
Offline Diversity Under Imitation Constraints
Learning Diverse Skills for Local Navigation
Learning Agile Skills via Adversarial Imitation of Rough Partial Demonstrations
Combinatorial Optimization as a Layer / Blackbox Differentiation
Object-centric Self-supervised Reinforcement Learning
Symbolic Regression and Equation Learning
Representation Learning
Stepsize adaptation for stochastic optimization
Probabilistic Neural Networks
Learning with 3D rotations: A hitchhiker’s guide to SO(3)
Super-resolution Sensing for Haptics
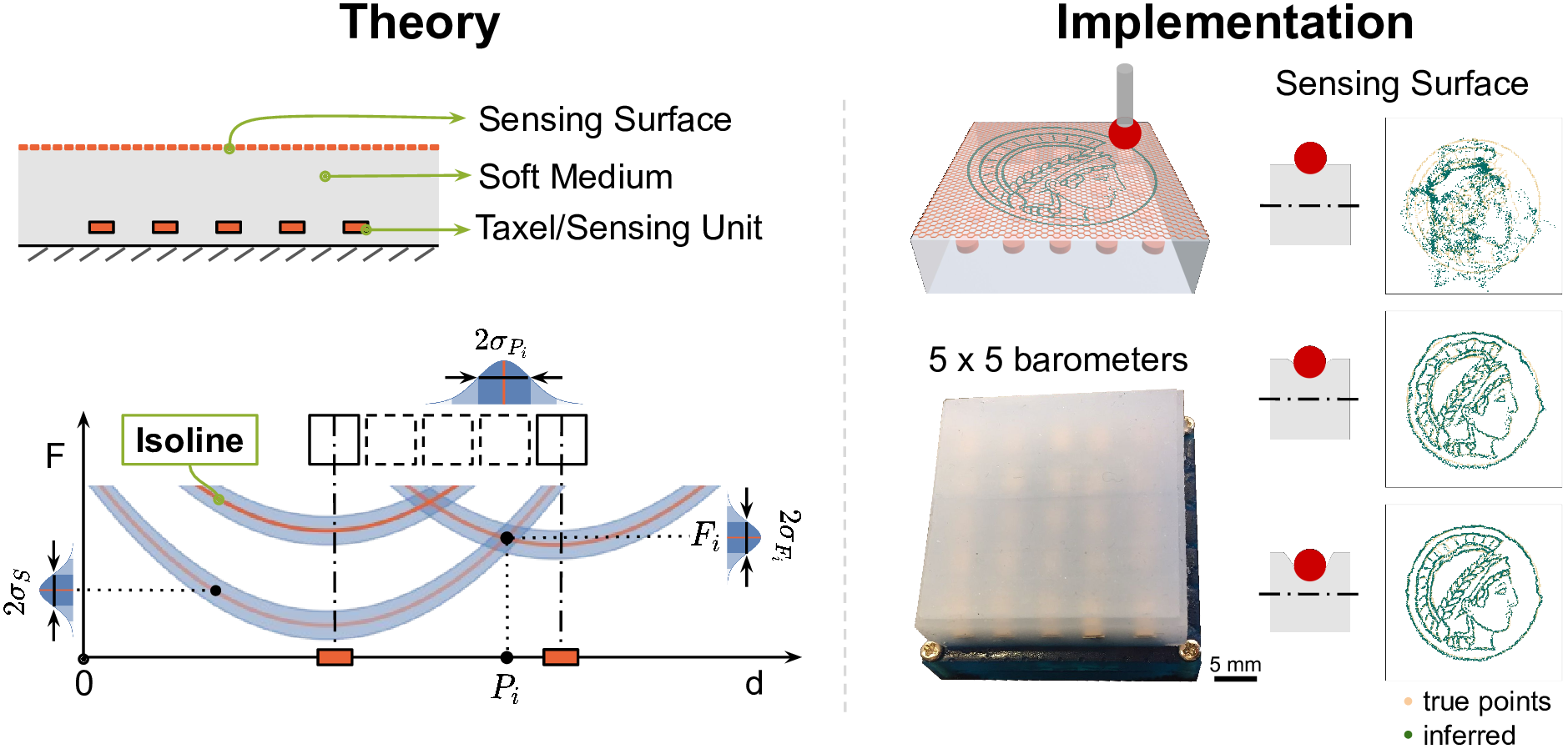
Haptic feedback is essential to make robots more dexterous and effective in unstructured environments. Nevertheless, high-resolution tactile sensors are still not widely available. When using a large number of physical sensing units we often face manufacturing challenges and wiring problems. Such complex hardware can also lead to durability issues as each additional component constitutes a potential point of failure.
We pursue a route towards high-resolution and robust tactile skins by embedding only a few sensor units (taxels) into a flexible surface material and use signal processing to achieve sensing with super-resolution accuracy. We first rely on the empirical knowledge that overlapping multiple taxels' perception fields enables super-resolution behavior and design a sensing system for robotic applications []. We then propose a theory for geometric super-resolution to guide the development of tactile sensors of this kind and link it to machine learning techniques for signal processing []. This theory is based on sensor isolines and allows us to predict force sensitivity and accuracy in contact position and force magnitude as a spatial quantity. We evaluate the influence of different factors, such as elastic properties of the material, structure design, and transduction methods, using finite element simulations and implemented real sensors. Using machine learning methods to infer contact information, our sensors obtain an unparalleled average super-resolution factor of over 1000. Our theory can guide future haptic sensor designs and inform various design choices.
Members
Publications