Reinforcement Learning and Control
Model-based Reinforcement Learning and Planning
Object-centric Self-supervised Reinforcement Learning
Self-exploration of Behavior
Causal Reasoning in RL
Equation Learner for Extrapolation and Control
Intrinsically Motivated Hierarchical Learner
Regularity as Intrinsic Reward for Free Play
Curious Exploration via Structured World Models Yields Zero-Shot Object Manipulation
Natural and Robust Walking from Generic Rewards
Goal-conditioned Offline Planning
Offline Diversity Under Imitation Constraints
Learning Diverse Skills for Local Navigation
Learning Agile Skills via Adversarial Imitation of Rough Partial Demonstrations
Combinatorial Optimization as a Layer / Blackbox Differentiation
Object-centric Self-supervised Reinforcement Learning
Symbolic Regression and Equation Learning
Representation Learning
Stepsize adaptation for stochastic optimization
Probabilistic Neural Networks
Learning with 3D rotations: A hitchhiker’s guide to SO(3)
Robust and Affordable Haptic Sensation with Sparse Sensor Configuration
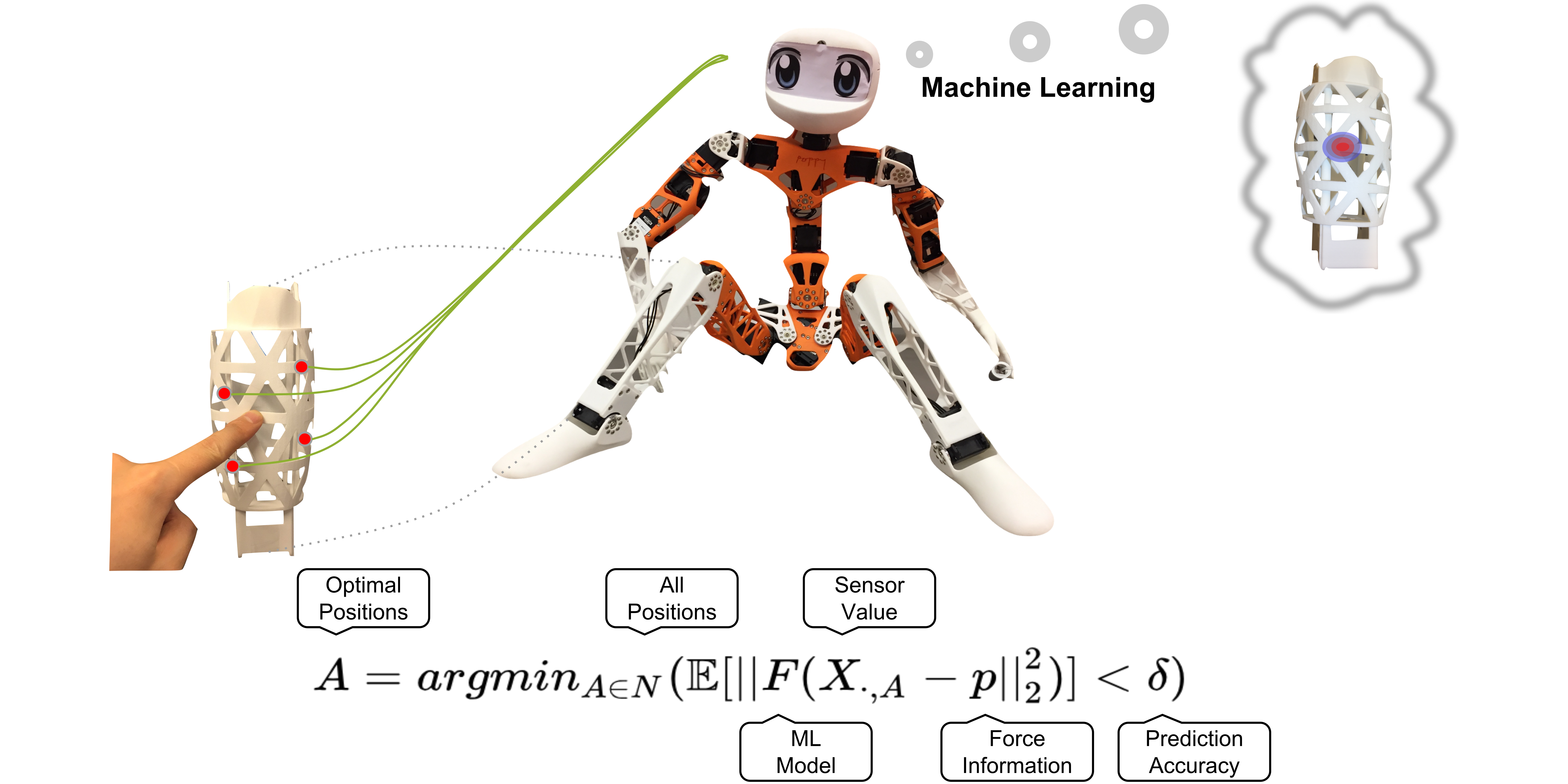
During the rapid development of robot technologies, actuators and sensors have become increasingly compact and powerful. Nevertheless, robots are still far from matching human capabilities especially when it comes to touch sensation. For this, haptic sensors have to be robust to sustain long-lasting experiments. Besides robustness, another important aspect of robotic hardware is its price, availability, and performance. A low cost makes robotic technologies widely accessible and thus facilitates research.
In this project, we aim at providing a low-cost, robust and sufficiently precise method for inferring haptic forces on the surface of 3D robot limbs. Instead of applying dense array-shaped sensors, we opt for a small number of sensors measuring internal deformation. This offers a couple of conceptual advantages: (1) The system is robust to environmental impacts because sensors are placed internally; (2) The surface shape can be freely designed; (3) Only a few channels have to be read out which reduces both the energy consumption and the data rate. On the downside, a measurement of the sensors does not directly correspond to the impacting force. Instead, an inference mechanism is required to estimate the force. We propose a data-driven approach using machine learning algorithms to perform this inference efficiently. To require as few sensors as possible, we employ several optimization schemes to determine optimal sensor placement.
The contributions of this project [] are as follows: On the theory side, we propose a new way of implementing a whole surface haptic sensor and provide a method for determining the optimal number and position of sensors using finite element method. On the application side, we provide a method to assemble the strain gauges, designed a hardware system to systematically collect data and demonstrated the sensing system on a robotic limb.
Members
Publications