Reinforcement Learning and Control
Model-based Reinforcement Learning and Planning
Object-centric Self-supervised Reinforcement Learning
Self-exploration of Behavior
Causal Reasoning in RL
Equation Learner for Extrapolation and Control
Intrinsically Motivated Hierarchical Learner
Regularity as Intrinsic Reward for Free Play
Curious Exploration via Structured World Models Yields Zero-Shot Object Manipulation
Natural and Robust Walking from Generic Rewards
Goal-conditioned Offline Planning
Offline Diversity Under Imitation Constraints
Learning Diverse Skills for Local Navigation
Learning Agile Skills via Adversarial Imitation of Rough Partial Demonstrations
Combinatorial Optimization as a Layer / Blackbox Differentiation
Object-centric Self-supervised Reinforcement Learning
Symbolic Regression and Equation Learning
Representation Learning
Stepsize adaptation for stochastic optimization
Probabilistic Neural Networks
Learning with 3D rotations: A hitchhiker’s guide to SO(3)
Predicting brain activity (fMRI)
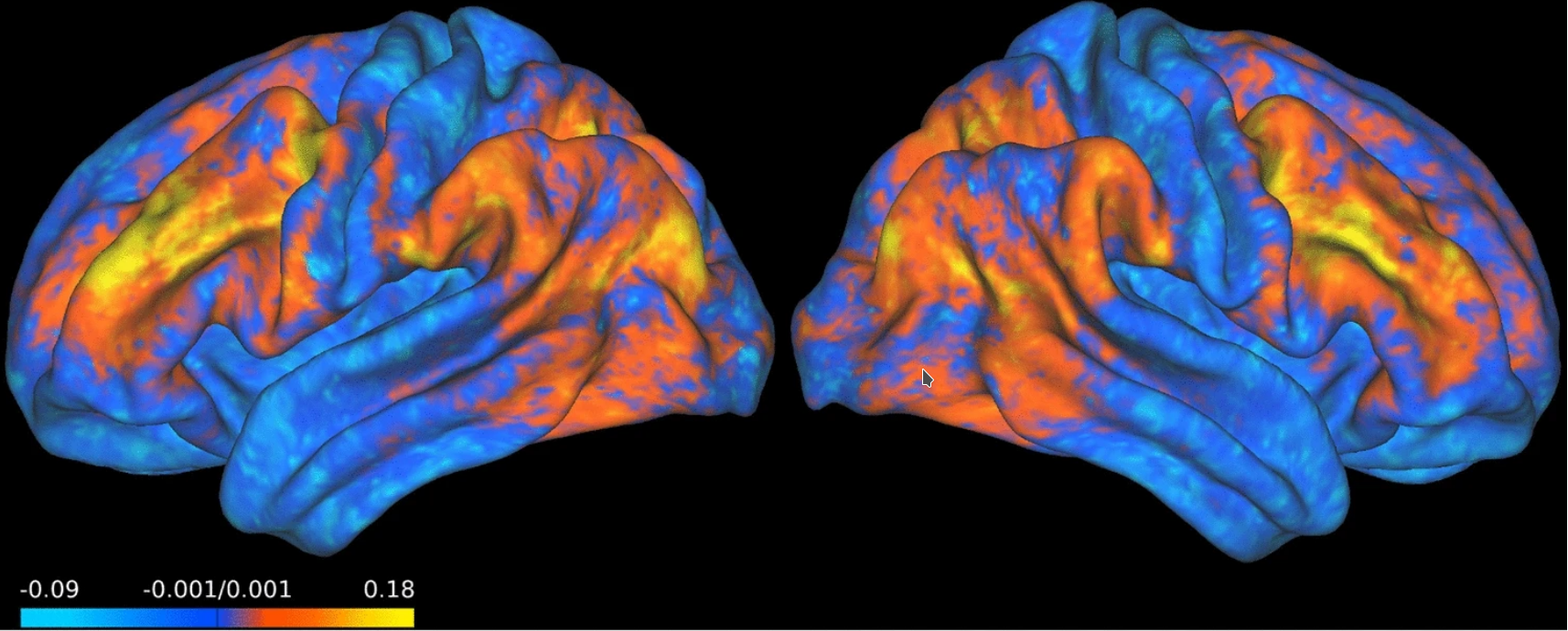
In this project, we look at the prediction of brain activity, measured by activation maps in fMRI, that occur while performing a task from the activation that occurs during resting. The latter means the patients are asked to rest in the fMRI scanner. Prior work has claimed significant prediction capabilities.
In the project, we check existing methods and find that with a stringent machine learning methodology many of the results are actually not better than a very simple baseline and did indeed not predict anything meaningful. We find that with improved techniques some of the prediction performance can be regained.
Members
Publications