Reinforcement Learning and Control
Model-based Reinforcement Learning and Planning
Object-centric Self-supervised Reinforcement Learning
Self-exploration of Behavior
Causal Reasoning in RL
Equation Learner for Extrapolation and Control
Intrinsically Motivated Hierarchical Learner
Regularity as Intrinsic Reward for Free Play
Curious Exploration via Structured World Models Yields Zero-Shot Object Manipulation
Natural and Robust Walking from Generic Rewards
Goal-conditioned Offline Planning
Offline Diversity Under Imitation Constraints
Learning Diverse Skills for Local Navigation
Learning Agile Skills via Adversarial Imitation of Rough Partial Demonstrations
Combinatorial Optimization as a Layer / Blackbox Differentiation
Object-centric Self-supervised Reinforcement Learning
Symbolic Regression and Equation Learning
Representation Learning
Stepsize adaptation for stochastic optimization
Probabilistic Neural Networks
Learning with 3D rotations: A hitchhiker’s guide to SO(3)
Causal Reasoning in RL
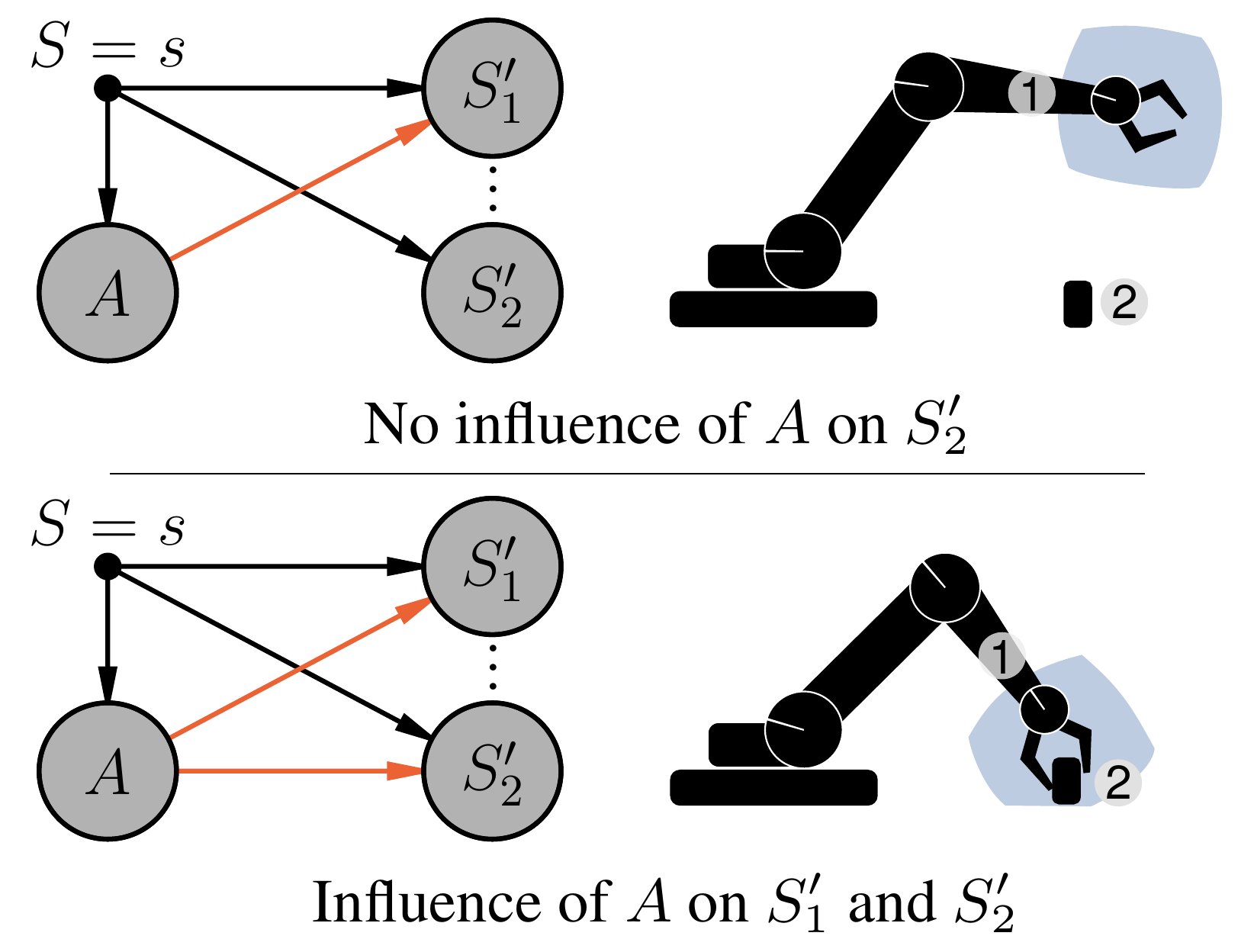
Reinforcement learning is fundamentally a causal endeavor. The agent intervenes in the environment through actions and observes their effects; learning through credit assignment involves the counterfactual question whether another action would have resulted in a better outcome. Causal Reasoning for RL is about learning and using causal knowledge to improve RL algorithms. We are interested in using tools from causality to make RL algorithms more robust and sample efficient.
In a first project [
, we showed how learning a causal model of agent-object interactions allows to infer whether the agent has causal influence on its environment. We then demonstrated how this information can be integrated into RL algorithms to steer the exploration process and improve sample-efficiency of off-policy training. Experiments on robotic manipulation benchmarks show a clear improvement over state-of-the-art.]
Members
Publications