Reinforcement Learning and Control
Model-based Reinforcement Learning and Planning
Object-centric Self-supervised Reinforcement Learning
Self-exploration of Behavior
Causal Reasoning in RL
Equation Learner for Extrapolation and Control
Intrinsically Motivated Hierarchical Learner
Regularity as Intrinsic Reward for Free Play
Curious Exploration via Structured World Models Yields Zero-Shot Object Manipulation
Natural and Robust Walking from Generic Rewards
Goal-conditioned Offline Planning
Offline Diversity Under Imitation Constraints
Learning Diverse Skills for Local Navigation
Learning Agile Skills via Adversarial Imitation of Rough Partial Demonstrations
Combinatorial Optimization as a Layer / Blackbox Differentiation
Object-centric Self-supervised Reinforcement Learning
Symbolic Regression and Equation Learning
Representation Learning
Stepsize adaptation for stochastic optimization
Probabilistic Neural Networks
Learning with 3D rotations: A hitchhiker’s guide to SO(3)
Self-exploration of Behavior
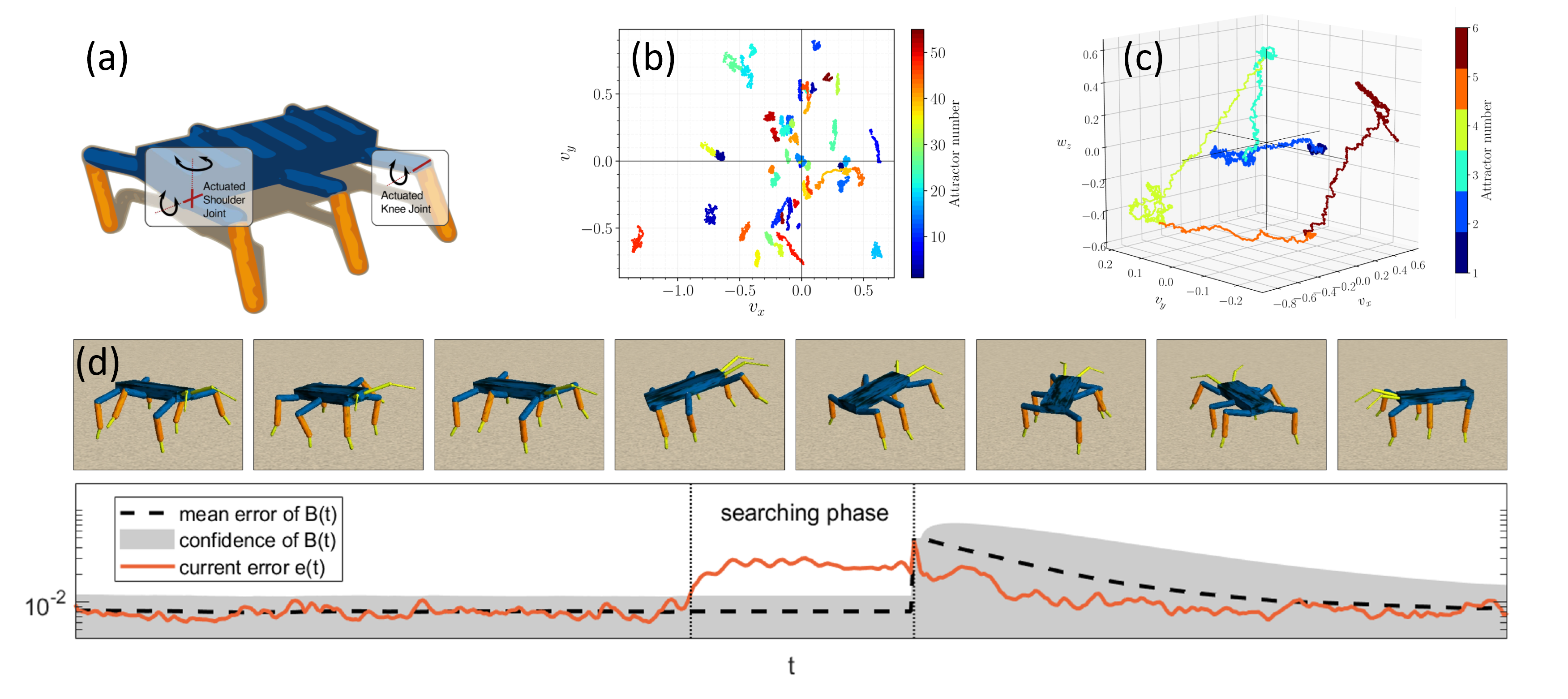
Humans and other animals need to learn behavior from exploring their sensorimotor capabilities. In this project we aim to understand how robotic systems can generate structured behavior, without specifying any reward nor objective function. Instead, the systems should explore behavior purely based on their embodiment and their interactions with the environment.
One way to bootstrap goal-free explorative process, is to use differential extrinsic plasticity (DEP)[]. DEP is a biologically plausible synaptic mechanism that can be used within very simple neural network controllers. When applying the DEP-controller to embodied agents, highly coordinated, rhythmic behaviors can emerge. These behaviors correspond to attractors of a dynamical system. When combining DEP with a “repelling potential” an agent can actively explore all its attractor behaviors in a systematic way [
]. As a result, highly complex robotic systems, e.g, a Hexapod robot (Fig. a), discover a variety of meaningful behaviors (Fig. b), such as various modes of locomotion.
Such self-organizing behavior can be used to discover and learn a repertoire of behavioral primitives from scratch. Our SUBMODES system [] explores behavior using the DEP-controller. During exploration, internal models are trained to predict the motor commands and the resulting sensory consequences of the performed behavior. The SUBMODES system use an unexpected increase in prediction error to detect transitions between behaviors and switch between internal models (Fig. d). In this way, the system is able systematically structure its sensorimotor experience on-line into compositional models of behavior that can later be used for planning and control.
Video
Members
Publications