Reinforcement Learning and Control
Model-based Reinforcement Learning and Planning
Object-centric Self-supervised Reinforcement Learning
Self-exploration of Behavior
Causal Reasoning in RL
Equation Learner for Extrapolation and Control
Intrinsically Motivated Hierarchical Learner
Regularity as Intrinsic Reward for Free Play
Curious Exploration via Structured World Models Yields Zero-Shot Object Manipulation
Natural and Robust Walking from Generic Rewards
Goal-conditioned Offline Planning
Offline Diversity Under Imitation Constraints
Learning Diverse Skills for Local Navigation
Learning Agile Skills via Adversarial Imitation of Rough Partial Demonstrations
Combinatorial Optimization as a Layer / Blackbox Differentiation
Object-centric Self-supervised Reinforcement Learning
Symbolic Regression and Equation Learning
Representation Learning
Stepsize adaptation for stochastic optimization
Probabilistic Neural Networks
Learning with 3D rotations: A hitchhiker’s guide to SO(3)
Neural Rendering
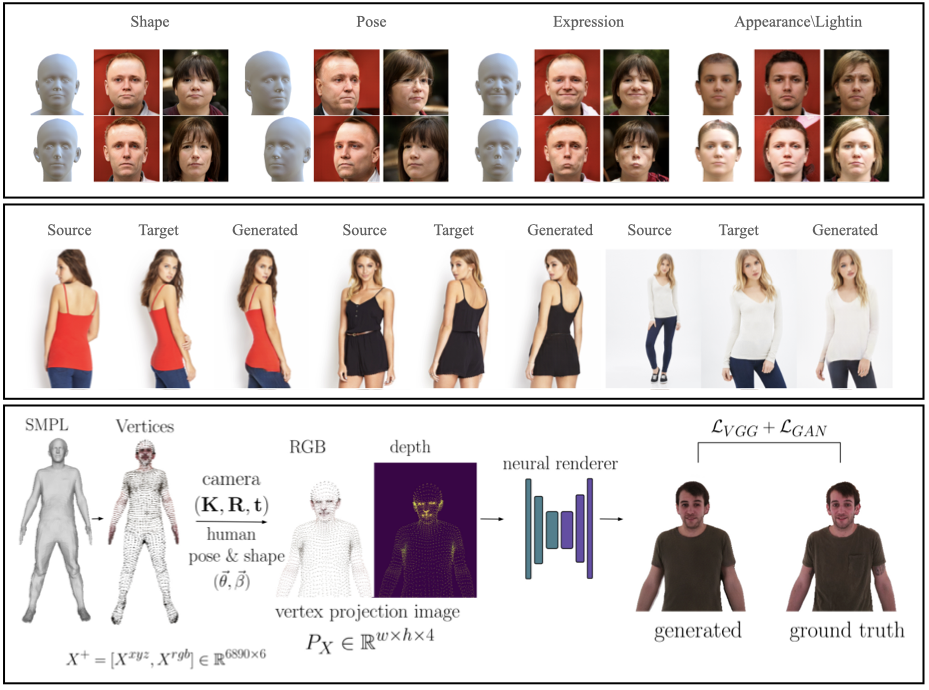
Conventional graphics pipelines take a 3D model like SMPL, apply texture and material properties, light it, and render it as an image. Without expensive artist involvement, this results in unrealistic images that fall into the "uncanny valley". To address this, we develop neural rendering methods that keep the 3D body model but replace the rendering pipeline with neural networks. This approach keeps the flexibility of parametric models while producing realistic looking images without artist intervention.
GIF [] generates realistic images of faces, by conditioning StyleGAN2 on the FLAME face model [
]. Given FLAME parameters for shape, pose, expressions, plus parameters for appearance, lighting, and an additional style vector, GIF outputs photo-realistic face images.
To generate images of people with realistic hair and clothing, we train SMPLpix [] to transform a sparse set of 3D mesh vertices and their RGB values into photorealistic images. The 3D mesh vertices are controllable with the pose and shape parameters of SMPL.
SPICE [] takes a different approach and synthesizes an image of a person in a novel pose given a source image of the person and a target pose. In contrast to typical approaches that require paired training data, SPICE uses only unpaired data. This is enabled by a novel cycle-GAN training method that exploits information about the 3D SMPL body.
The combination of parametric 3D models with neural rendering enables realistic human rendering with intuitive animation controls.
Members
Publications