Reinforcement Learning and Control
Model-based Reinforcement Learning and Planning
Object-centric Self-supervised Reinforcement Learning
Self-exploration of Behavior
Causal Reasoning in RL
Equation Learner for Extrapolation and Control
Intrinsically Motivated Hierarchical Learner
Regularity as Intrinsic Reward for Free Play
Curious Exploration via Structured World Models Yields Zero-Shot Object Manipulation
Natural and Robust Walking from Generic Rewards
Goal-conditioned Offline Planning
Offline Diversity Under Imitation Constraints
Learning Diverse Skills for Local Navigation
Learning Agile Skills via Adversarial Imitation of Rough Partial Demonstrations
Combinatorial Optimization as a Layer / Blackbox Differentiation
Object-centric Self-supervised Reinforcement Learning
Symbolic Regression and Equation Learning
Representation Learning
Stepsize adaptation for stochastic optimization
Probabilistic Neural Networks
Learning with 3D rotations: A hitchhiker’s guide to SO(3)
Psychology
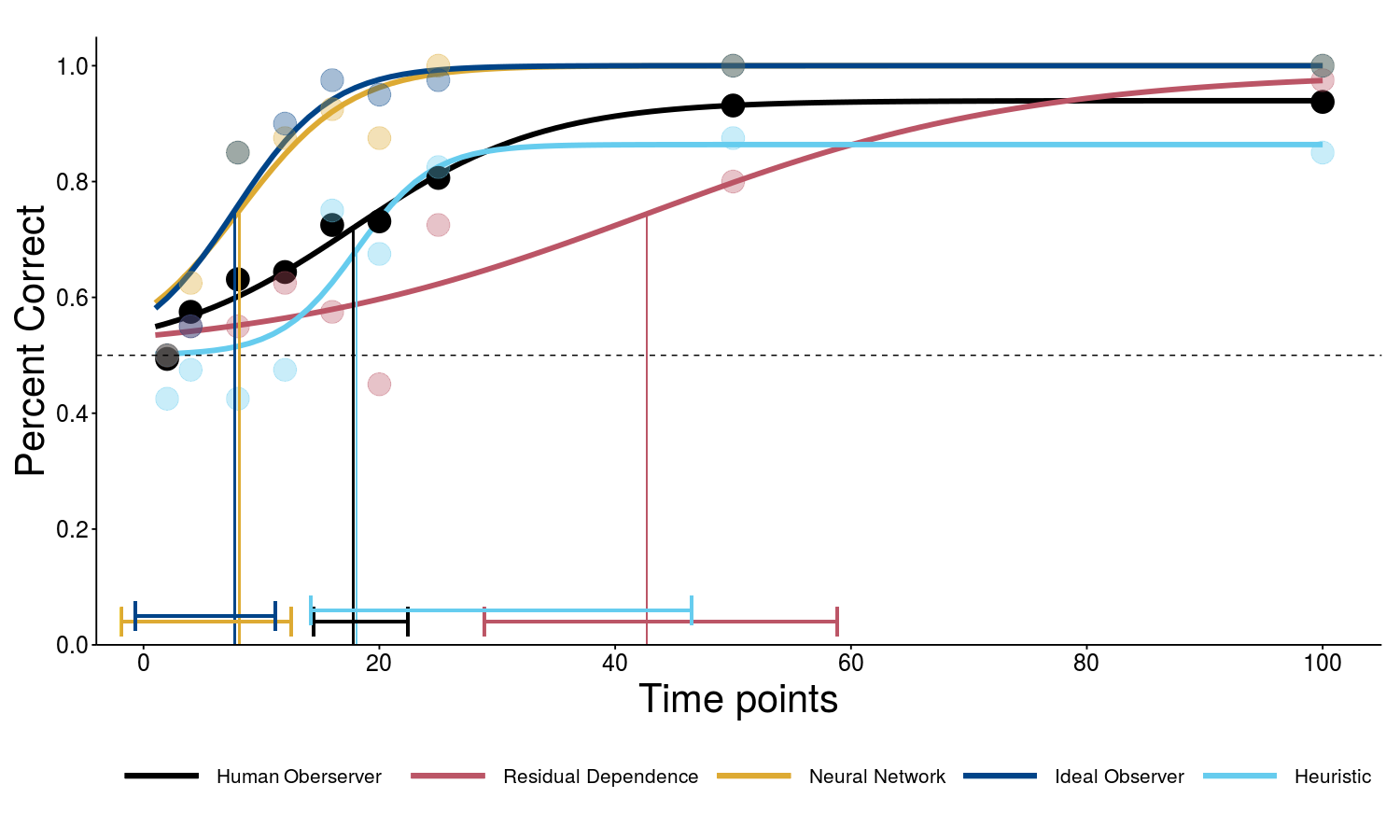
Much of the research in cognitive science and an increasing fraction of that in machine learning agree that truly intelligent behaviour requires causal representation of the world. A central research question is which behaviourally relevant input data support causal inference algorithms of human perception: what are the critical cues in complex, high-dimensional real-world data impinging on our sensory systems that our causal inference algorithms run upon?
Recent ML algorithms exploit the dependence structure of additive noise terms for inferring causal structures from observational data [], e.g., to detect the direction of time series; the arrow of time. This raises the question whether the subtle asymmetries between the time directions can also be perceived by humans.
We show [] that human observers can indeed discriminate forward and backward autoregressive motion with non-Gaussian additive independent noise, i.e., they appear sensitive to subtle asymmetries between the time directions. We employ a so-called frozen noise paradigm enabling us to compare human performance with four different recent ML algorithms. Our results suggest that all human observers use similar cues or strategies to solve the arrow of time motion discrimination task, but the human algorithm is significantly different from the three machine algorithms we compared it to.
Together with the results of an additional experiment []—using classical as well as modified Michotte launching displays—we now believe that the human ability to "see causes'' is at least in our settings an early, a perceptual rather than a late, or deliberate, cognitive ability.
Another project of interest to psychology studied how to enhance human learning in spaced repetition schemes as used, e.g., in language learning [].
Members
Publications