Human Pose, Shape and Action
3D Pose from Images
2D Pose from Images
Beyond Motion Capture
Action and Behavior
Body Perception
Body Applications
Pose and Motion Priors
Clothing Models (2011-2015)
Reflectance Filtering
Learning on Manifolds
Markerless Animal Motion Capture
Multi-Camera Capture
2D Pose from Optical Flow
Body Perception
Neural Prosthetics and Decoding
Part-based Body Models
Intrinsic Depth
Lie Bodies
Layers, Time and Segmentation
Understanding Action Recognition (JHMDB)
Intrinsic Video
Intrinsic Images
Action Recognition with Tracking
Neural Control of Grasping
Flowing Puppets
Faces
Deformable Structures
Model-based Anthropometry
Modeling 3D Human Breathing
Optical flow in the LGN
FlowCap
Smooth Loops from Unconstrained Video
PCA Flow
Efficient and Scalable Inference
Motion Blur in Layers
Facade Segmentation
Smooth Metric Learning
Robust PCA
3D Recognition
Object Detection
Multi-view Stereo
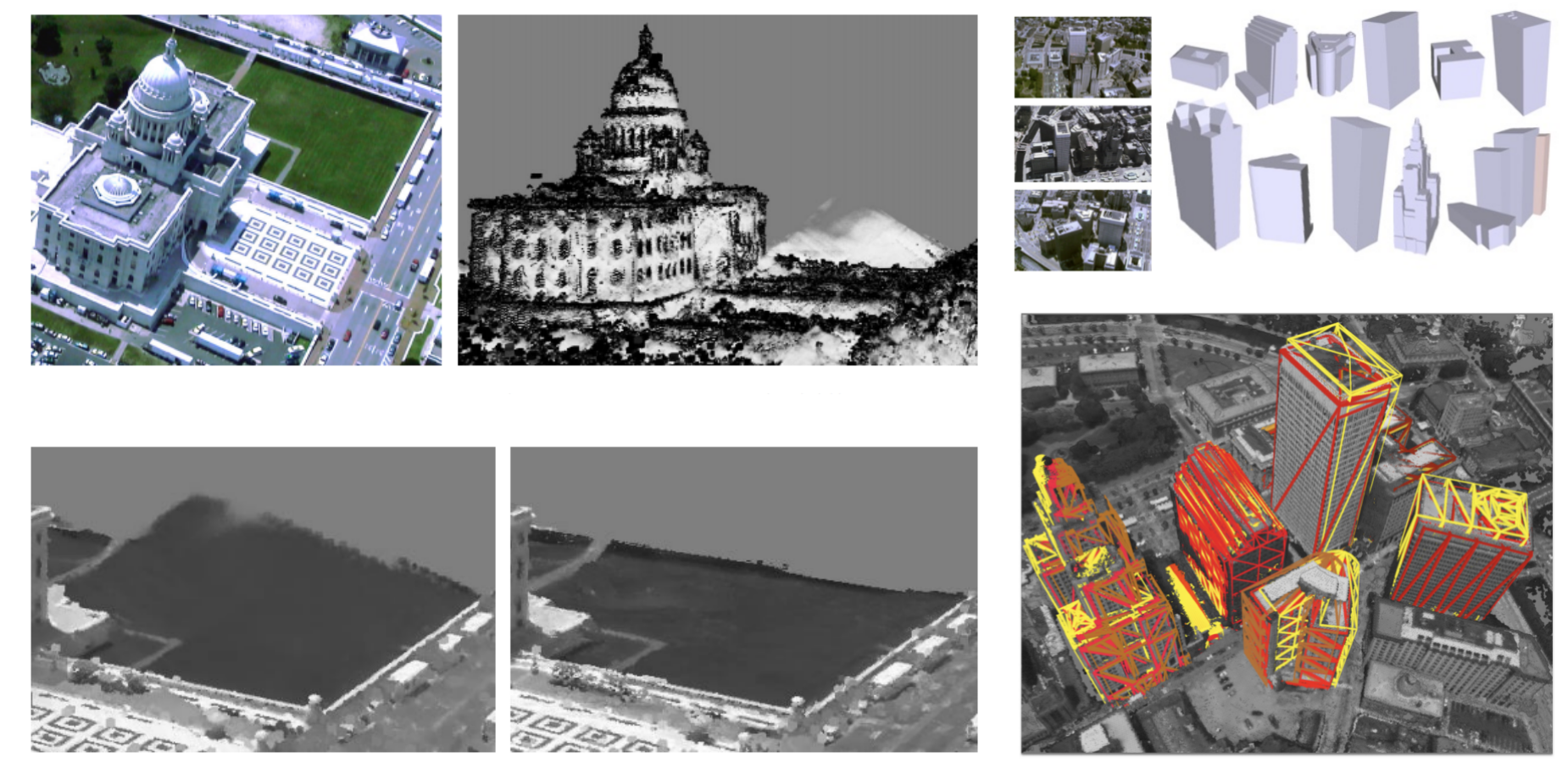
Dense 3D reconstruction from RGB images is a highly ill-posed problem due to occlusions, textureless or reflective surfaces, varied scene geometry, and spatial discontinuities. We propose algorithms that bring in various types of geometric information that imposes long-range, or semantic, knowlege to address these ambiguities.
Our work on Patches, Planes and Probabilities [] proposed a novel Markov random field model based on ray potentials and a non-local structured prior for volumetric multi-view 3D reconstruction. It was inspired by the planar nature of many elements in man-made environments, i.e., 3D range images of generic scenes can be approximated by piecewise smooth regions with discontinuities at object boundaries. The prior encourages planarity within image segments and regularizes over large voxel neighborhoods. The method was able to resolve reconstruction ambiguities of textureless and partially reflective surfaces and achieved state-of-the-art results in reconstruction accuracy for highly challenging aerial datasets.
In our work on Semantic Multi-view Stereo [], we address ambiguities in 3D reconstruction by presenting a probabilistic approach that integrates object-level shape priors with image-based 3D reconstruction. Our method can infer not only a dense 3D reconstruction of the scene but the existence and precise 3D pose of the objects in it as well. Thus our method not only yields an accurate mapping of the environment but also a semantic understanding in terms of the objects in the environment. The proposed prior allows for powerful regularization that can resolve large ambiguities common in 3D reconstruction. For instance, our shape prior can help reconstruct the back-side of an object even though it is occluded in the images.
Members
Publications