Human Pose, Shape and Action
3D Pose from Images
2D Pose from Images
Beyond Motion Capture
Action and Behavior
Body Perception
Body Applications
Pose and Motion Priors
Clothing Models (2011-2015)
Reflectance Filtering
Learning on Manifolds
Markerless Animal Motion Capture
Multi-Camera Capture
2D Pose from Optical Flow
Body Perception
Neural Prosthetics and Decoding
Part-based Body Models
Intrinsic Depth
Lie Bodies
Layers, Time and Segmentation
Understanding Action Recognition (JHMDB)
Intrinsic Video
Intrinsic Images
Action Recognition with Tracking
Neural Control of Grasping
Flowing Puppets
Faces
Deformable Structures
Model-based Anthropometry
Modeling 3D Human Breathing
Optical flow in the LGN
FlowCap
Smooth Loops from Unconstrained Video
PCA Flow
Efficient and Scalable Inference
Motion Blur in Layers
Facade Segmentation
Smooth Metric Learning
Robust PCA
3D Recognition
Object Detection
The AI animator
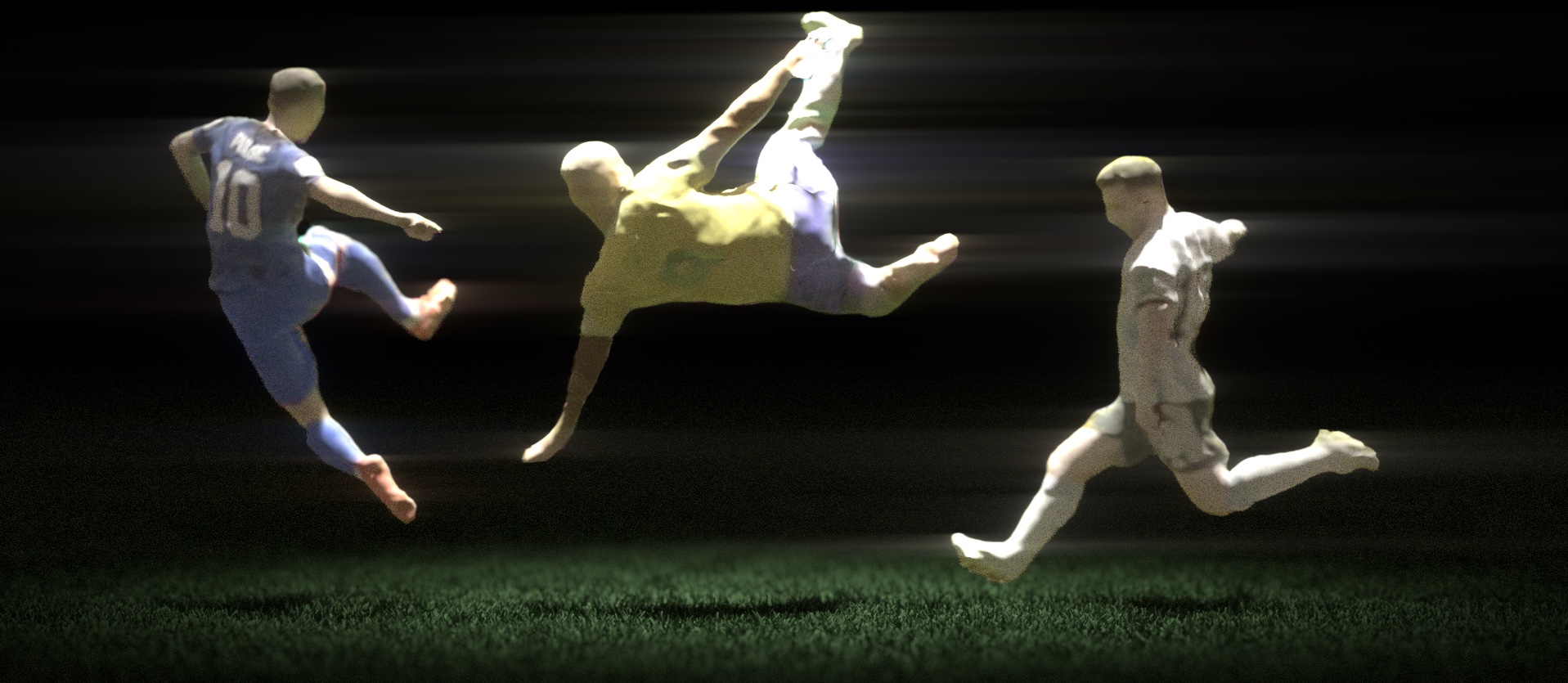
Generative AI is evolving rapidly and many argue that GenAI will fully replace traditional graphics. There is nothing really wrong, however, with traditional graphics except that it requires extensive experience and time to create realistic images and video. So, instead, can we use GenAI to make traditional graphics methods more accessible?
Hair: Traditional hair models are based on strands and creating hairstyles with strands requires experience. To automate this, MonoHair [] performs high-fidelity hair reconstruction of strands from a monocular video, while Gaussian Haircut [
] exploits Gaussian splatting to reconstruct hairstyles from video in the form of strand-aligned 3D Gaussians. With HAAR [
], we train the first text-conditioned diffusion model that outputs hairstyles using guide strands represented in a latent space. We upsample these strands and the result can be rendered using off-the-shelf computer graphics techniques.
Clothing: Designing, draping, and animating clothing is time consuming. HOOD [] leverages graph neural networks (GNN), multi-level message passing, and unsupervised training to enable real-time prediction of realistic clothing dynamics. By modeling clothing as a GNN we maintain all the benefits of meshes while enabling changes in topology due to things like buttons and zippers. ContourCraft [
] goes further to simulate complex multi-layer outfits. Using a novel Intersection Contour loss term, it can both prevent and resolve intersections in neural cloth simulations.
Head avatars: We have explored methods for estimating animatable 3D head avatars using implicit functions (IMavatar) [], point clouds (PointAvatar) [
], meshes (FLARE) [
], and a mix of meshes and radiance fields (TECA [
], INSTA [
]). We have also worked to reduce racial bias in skin texture estimates under varied lighting (TRUST) [
].
Full-body avatars: Our goal is to make avatar creation so simple that it can be done from a single photo, a text description, or even a personal photo collection. We have led the field over the reporting period with papers that explore numerous ways of creating avatars (generative and regression) and numerous representations (implicit, points, meshes, and hybrids). ICON [], ECON [
], SCARF [
], TeCH [
], TADA! [
], AG3D [
], gDNA [
], Fast-SNARF [
], SCULPT [
] and PuzzleAvatar [
] are just some of the methods we developed. All these methods combine the animation benefits of SMPL-style models with richer shape and appearance representations.