Human Pose, Shape and Action
3D Pose from Images
2D Pose from Images
Beyond Motion Capture
Action and Behavior
Body Perception
Body Applications
Pose and Motion Priors
Clothing Models (2011-2015)
Reflectance Filtering
Learning on Manifolds
Markerless Animal Motion Capture
Multi-Camera Capture
2D Pose from Optical Flow
Body Perception
Neural Prosthetics and Decoding
Part-based Body Models
Intrinsic Depth
Lie Bodies
Layers, Time and Segmentation
Understanding Action Recognition (JHMDB)
Intrinsic Video
Intrinsic Images
Action Recognition with Tracking
Neural Control of Grasping
Flowing Puppets
Faces
Deformable Structures
Model-based Anthropometry
Modeling 3D Human Breathing
Optical flow in the LGN
FlowCap
Smooth Loops from Unconstrained Video
PCA Flow
Efficient and Scalable Inference
Motion Blur in Layers
Facade Segmentation
Smooth Metric Learning
Robust PCA
3D Recognition
Object Detection
3D Pose from Images
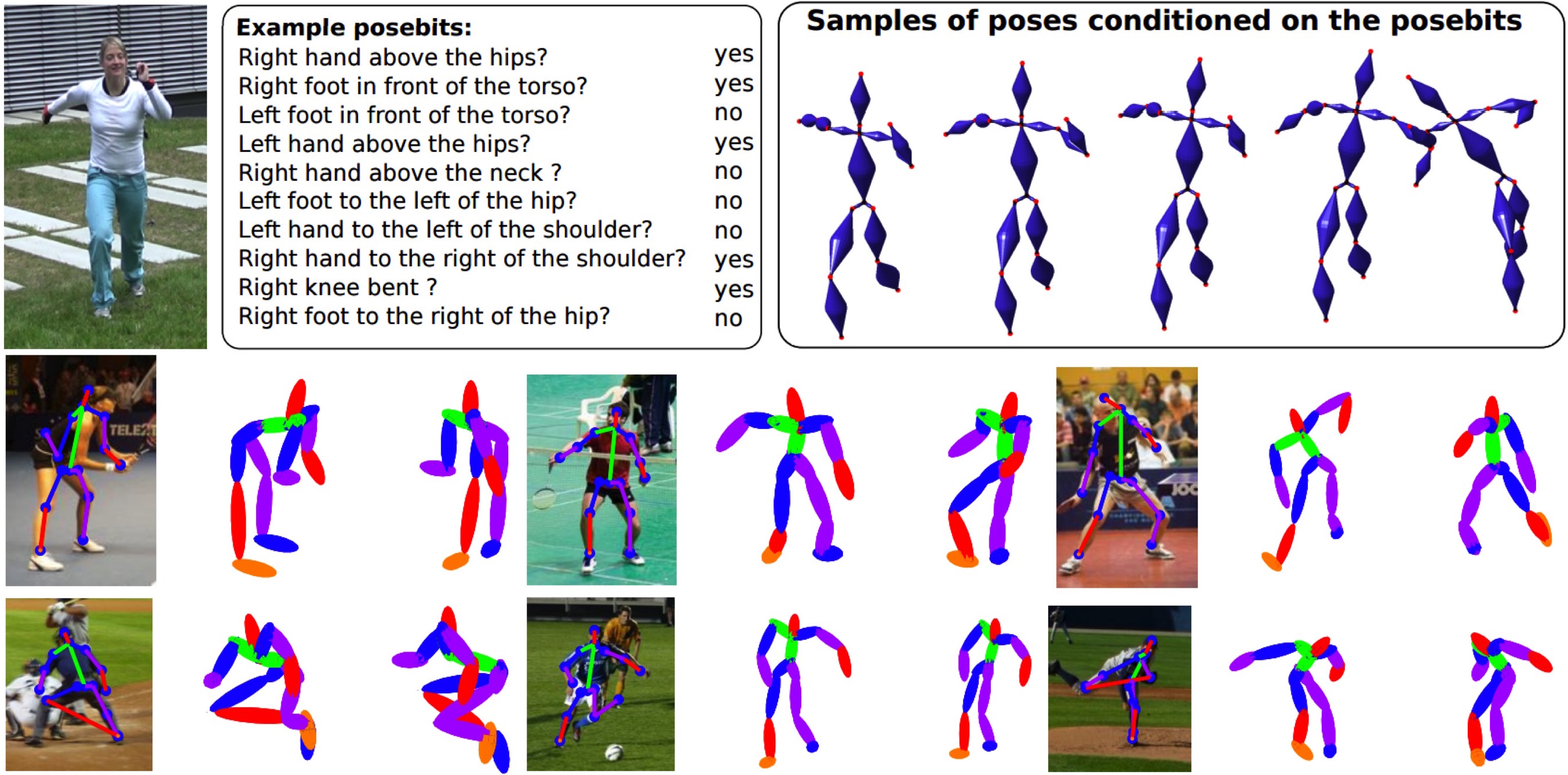
The estimation of 3D human pose from 2D images is inherently ambiguous. To that end, we develop inference methods and human pose models that enable prediction of 3D pose from images. Learned models of human pose rely on training data but we find that existing motion capture datasets are too limited to explore the full range of human poses.
In [] we advocate the inference of qualitative information about 3D human pose, called posebits, from images. Posebits are relationships between body parts (e.g. left-leg in front of right-leg or hands close to each other). The advantages of posebits as a mid-level representation are 1) for many tasks of interest, such qualitative pose information may be sufficient (e.g. semantic image retrieval), 2) it is relatively easy to annotate large image corpora with posebits, as it simply requires answers to yes/no questions; and 3) they help resolve challenging pose ambiguities and therefore facilitate the difficult task of image-based 3D pose estimation. We introduce posebits, a posebit database, a method for selecting useful posebits for pose estimation and a structural SVM model for posebit inference.
In [] we make two key contributions to estimate 3D pose from 2D joint locations. First, we collect a dataset that includes a wide range of human poses that are designed to explore the limits of human pose. We notice that joint limits actually vary with pose and learn the first pose-dependent prior of joint limits. Second, we introduce a new method to infer 3D poses from 2D using the learned prior and an over-complete dictionary of poses. This results in state of the art results on estimating 3D pose from 2D pose.
Members
Publications