Human Pose, Shape and Action
3D Pose from Images
2D Pose from Images
Beyond Motion Capture
Action and Behavior
Body Perception
Body Applications
Pose and Motion Priors
Clothing Models (2011-2015)
Reflectance Filtering
Learning on Manifolds
Markerless Animal Motion Capture
Multi-Camera Capture
2D Pose from Optical Flow
Body Perception
Neural Prosthetics and Decoding
Part-based Body Models
Intrinsic Depth
Lie Bodies
Layers, Time and Segmentation
Understanding Action Recognition (JHMDB)
Intrinsic Video
Intrinsic Images
Action Recognition with Tracking
Neural Control of Grasping
Flowing Puppets
Faces
Deformable Structures
Model-based Anthropometry
Modeling 3D Human Breathing
Optical flow in the LGN
FlowCap
Smooth Loops from Unconstrained Video
PCA Flow
Efficient and Scalable Inference
Motion Blur in Layers
Facade Segmentation
Smooth Metric Learning
Robust PCA
3D Recognition
Object Detection
Generating human motion
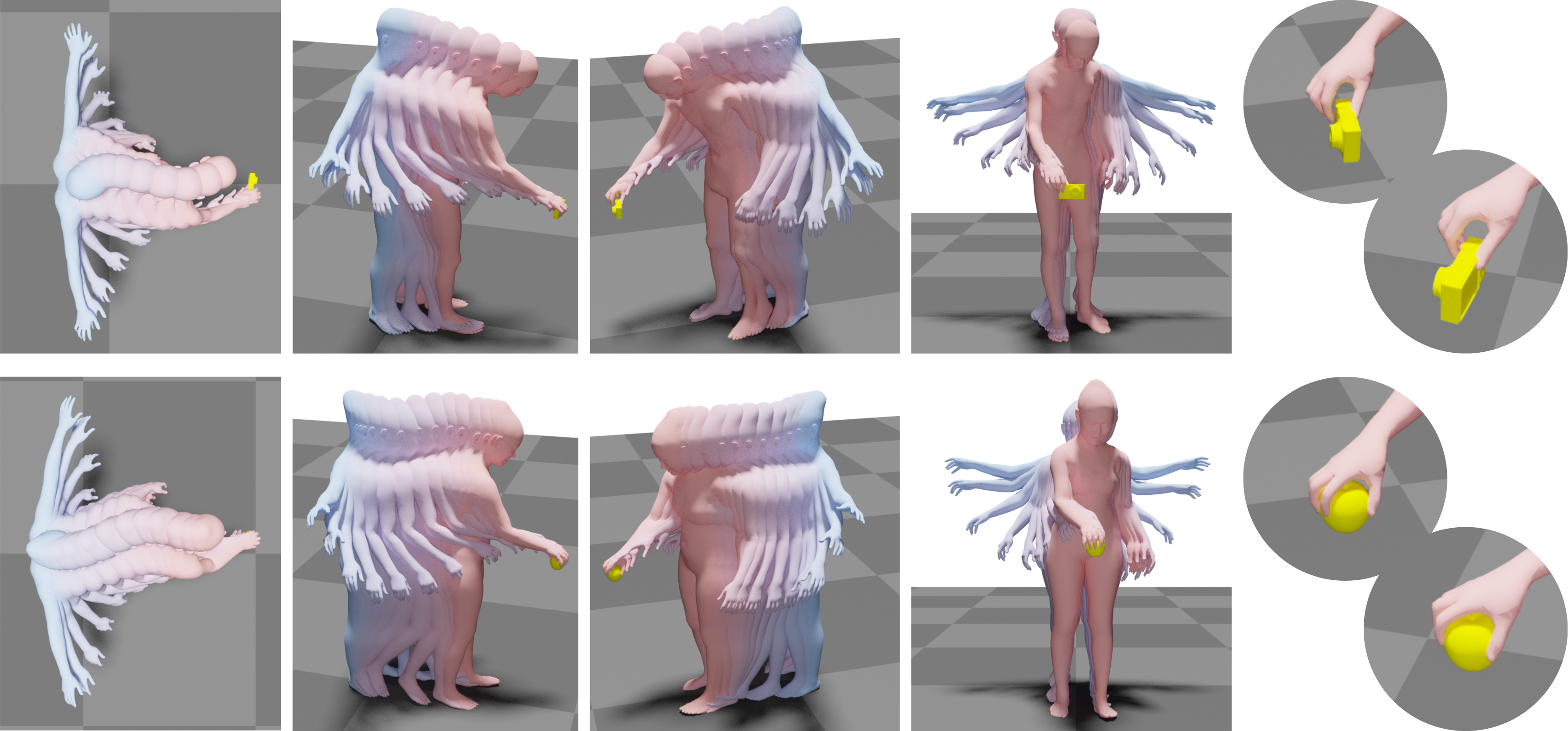
Animation of 3D humans today is mostly achieved through motion capture (mocap) or hand animation. We envision a very different world in which animation is ubiquitous, on demand, and in context. We are developing the datasets and methods to enable generation of 3D human motion with the goal of making the generated movement indistinguishable from that of real humans. To that end, we focus on several key challenges:
Motion from text: Given a textual description of a human behavior, the goal is to generate the 3D motion that matches the description. TEMOS [] is the first text-conditioned generative model to encode and decode full motions using a variational auto-econding approach based on transformers. TEMOS has been highly influential in driving the field. We leverage TEMOS to encode language and human motion into a cross-modal embedding space. We leverage this in TMR [
] to enable zero-shot retrieval of 3D motions from a mocap dataset based on text descriptions.
Motions in scenes with contact: Human movements always occur in the context of the scene, the objects in it, and the goals of the agent. To generate such human-object interaction, we developed GOAL [], the first generative model of full-body motion and grasping. It exploits two networks, one that generates a pose of the body and hand that grasps a given object and a second that generates movement from a starting pose to this goal pose. GraspXL [
] focuses on generating hand object interaction at scale by leveraging a policy learning framework that leverages physics simulation and reinforcement learning. WANDR [
] uses a conditional Variational AutoEncoder to generate realistic motion of human avatars that navigate towards an arbitrary goal location and reach for it. It is the first human motion generation model that is driven by an active feedback loop learned purely from data, without any extra steps of reinforcement learning (RL). With MIME [
] we take the novel approach that flips the problem around by generating the scene based on the human movement. Given 3D movement, we automatically infer the layout of 3D furniture that is consistent with the movement. This enables us to generate novel synthetic training data at scale.
Emotional face, hand, and body movement: We move our bodies and faces to communicate and with EMAGE [] and AMUSE [
], we explore the generation of full-body movement from audio. To do so, we created a novel dataset of full-body movements in SMPL-X format together with diverse emotional speech. Additionally, we train EMOTE [
] to provide facial animation with editable emotion control.