Human Pose, Shape and Action
3D Pose from Images
2D Pose from Images
Beyond Motion Capture
Action and Behavior
Body Perception
Body Applications
Pose and Motion Priors
Clothing Models (2011-2015)
Reflectance Filtering
Learning on Manifolds
Markerless Animal Motion Capture
Multi-Camera Capture
2D Pose from Optical Flow
Body Perception
Neural Prosthetics and Decoding
Part-based Body Models
Intrinsic Depth
Lie Bodies
Layers, Time and Segmentation
Understanding Action Recognition (JHMDB)
Intrinsic Video
Intrinsic Images
Action Recognition with Tracking
Neural Control of Grasping
Flowing Puppets
Faces
Deformable Structures
Model-based Anthropometry
Modeling 3D Human Breathing
Optical flow in the LGN
FlowCap
Smooth Loops from Unconstrained Video
PCA Flow
Efficient and Scalable Inference
Motion Blur in Layers
Facade Segmentation
Smooth Metric Learning
Robust PCA
3D Recognition
Object Detection
Reconstructing Sign-Language Movements from Images and Bioimpedance Measurements
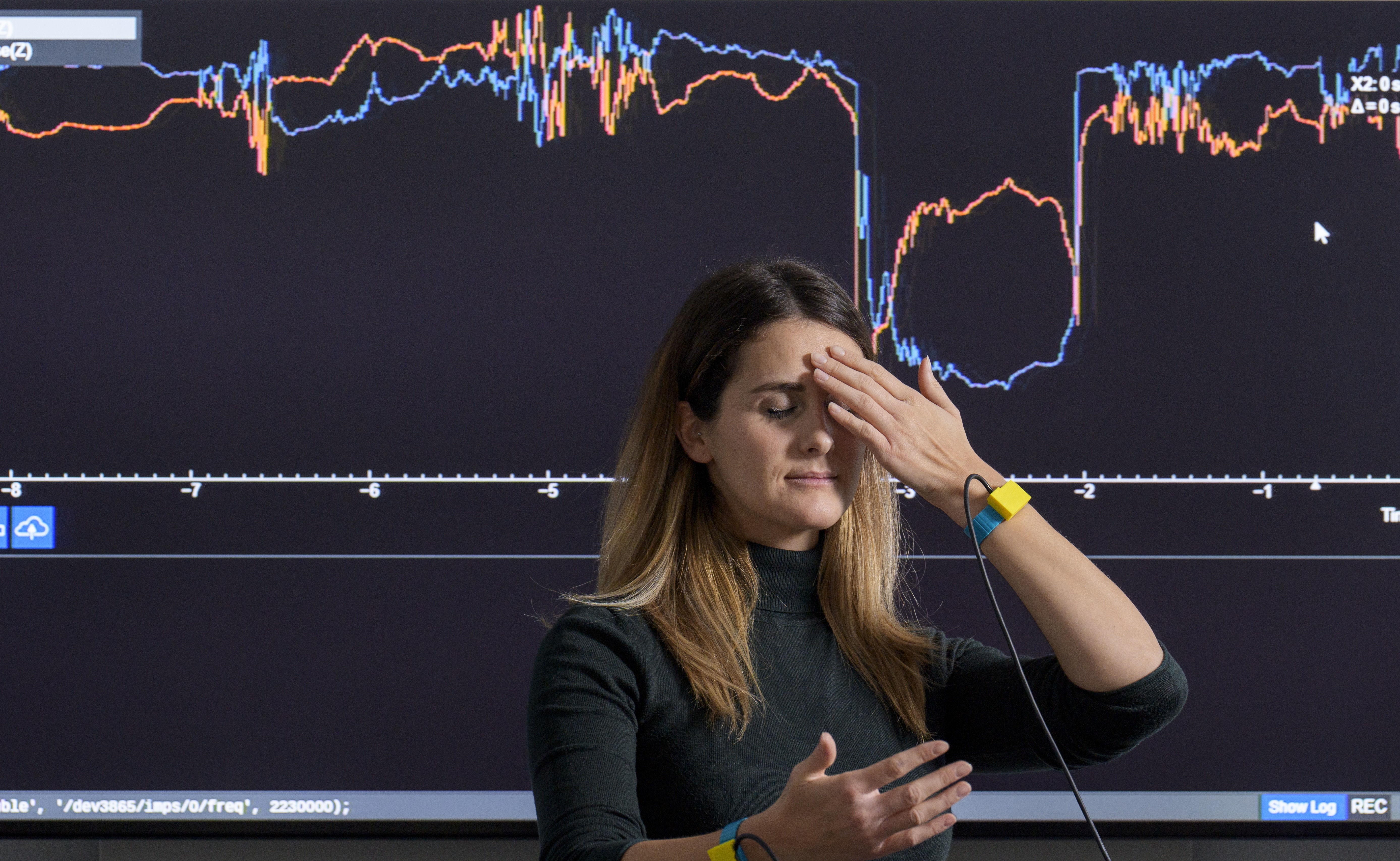
Sign language is the primary means of communication for more than 70 million Deaf people worldwide. While video dictionaries are widely used for learning signs, they have limitations in terms of viewpoint and integration into AR/VR applications. Converting these videos into expressive 3D avatars could enhance learning and accessibility, but existing reconstruction methods struggle with the inherent challenges of sign language movements, such as rapid motion, self-occlusions, and complex finger articulation.
We propose a novel approach that combines complementary sensing modalities to achieve robust sign language capture. Our research objective is to test the hypothesis that fusing computer vision with electrical bioimpedance sensing between the wrists enables accurate reconstruction of signing, even in challenging real-world conditions.
Our first work, SGNify, introduces linguistic constraints derived from universal rules of sign languages to improve 3D reconstruction from monocular video []. We evaluated our system through quantitative analysis using motion capture data as ground truth, as well as perceptual studies with fluent signers. Results showed that our approach significantly outperforms existing methods, producing more accurate reconstructions. However, the resulting avatars still suffer from depth ambiguities, especially during self-contact events.
Based on our findings that self-contact remains ambiguous in video alone, we then investigated whether electrical bioimpedance measured between the wrists could be used to detect self-contact []. We conducted a systematic data collection with 30 participants, each performing 33 different poses that involved various combinations of body parts (hands touching each other, hands touching face, hands touching chest) and contact sizes (from single fingertip to full hand) and a no-contact baseline pose. We measured their bioimpedance while sweeping frequencies from 100 Hz to 5.1 MHz. Our results demonstrated that the bioimpedance magnitude at high frequencies can be used to reliably detect skin-to-skin contact across individuals with diverse physical characteristics. Ongoing work is evaluating the extent to which direct-sensed bioimpedance improves SGNify's performance.
Members
Publications