Human Pose, Shape and Action
3D Pose from Images
2D Pose from Images
Beyond Motion Capture
Action and Behavior
Body Perception
Body Applications
Pose and Motion Priors
Clothing Models (2011-2015)
Reflectance Filtering
Learning on Manifolds
Markerless Animal Motion Capture
Multi-Camera Capture
2D Pose from Optical Flow
Body Perception
Neural Prosthetics and Decoding
Part-based Body Models
Intrinsic Depth
Lie Bodies
Layers, Time and Segmentation
Understanding Action Recognition (JHMDB)
Intrinsic Video
Intrinsic Images
Action Recognition with Tracking
Neural Control of Grasping
Flowing Puppets
Faces
Deformable Structures
Model-based Anthropometry
Modeling 3D Human Breathing
Optical flow in the LGN
FlowCap
Smooth Loops from Unconstrained Video
PCA Flow
Efficient and Scalable Inference
Motion Blur in Layers
Facade Segmentation
Smooth Metric Learning
Robust PCA
3D Recognition
Object Detection
Virtual Humans (2011-2015)
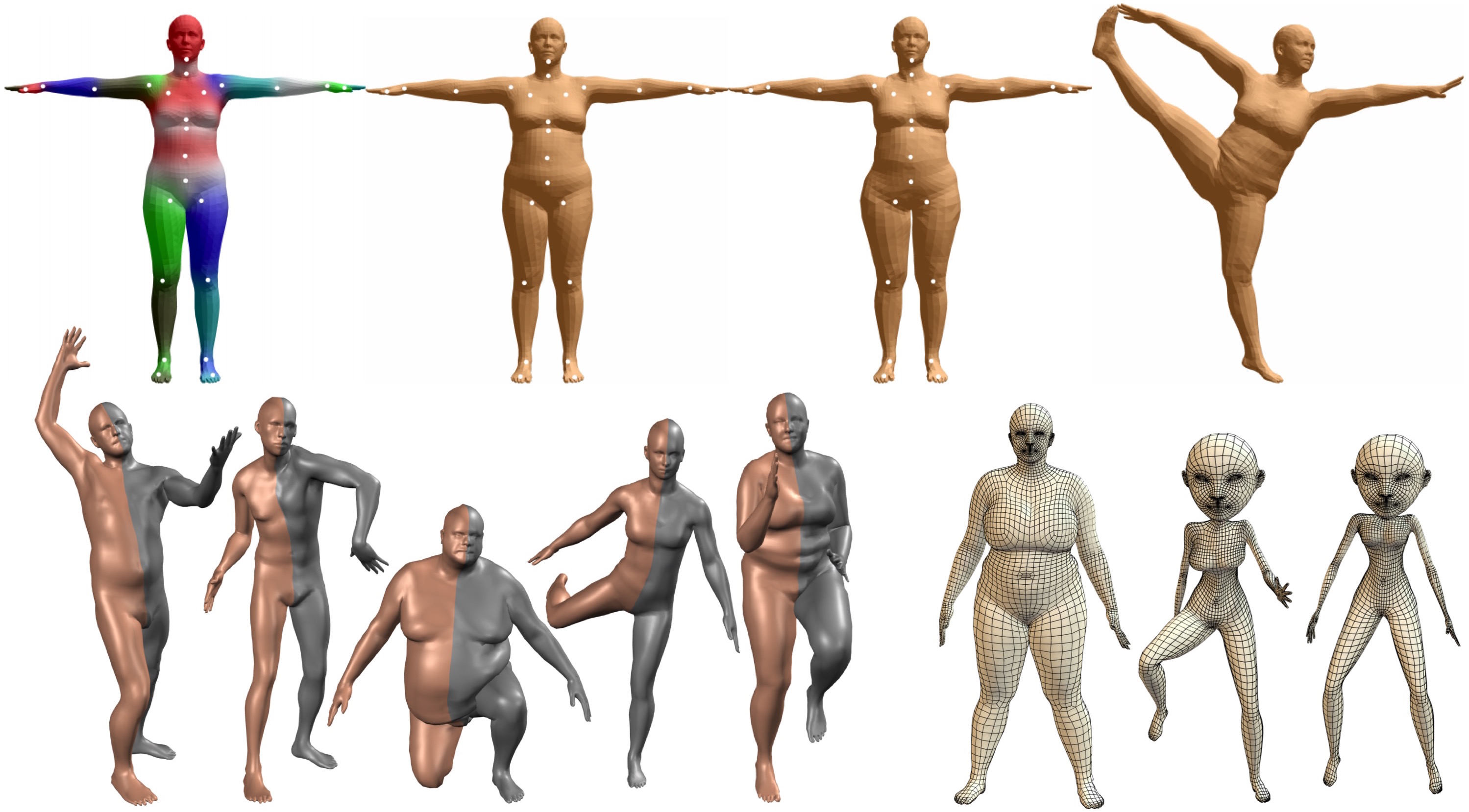
The human body is certainly central to our lives and is commonly depicted in images and video. We are developing the world's most realistic models of the body by learning their shape and how they move from data. Our goal is to make 3D models of the body look and move in ways that make them indistinguishable from real humans. Such virtual humans can be used in special effects and will play an important role in emerging virtual reality systems. They can also be used in computer vision to generate training data for learning methods or can be fit directly to sensor data. What makes this hard is that the human body is highly articulated, deforms with kinematic changes, and exhibits large shape variability across subjects.
Over the last five years we have developed a series of 3D body models that can be used for both graphics and vision: BlendSCAPE [], Delta [
], Dyna [
] and finally SMPL [
]. In particular, SMPL is a realistic human body model that is more accurate than previous SCAPE models yet is based on standard blend skinning and blend shapes. Pose blend shapes correct blend-skinning artifacts and are driven by elements of the body part rotation matrices. Shape blend shapes capture how body shape varies across people; these are computed using pose normalized 3D scans of 4000 people. Dynamic blend shapes capture how soft tissue deforms with motion.
The simplicity of our formulation means that SMPL can be trained from large amounts of data. It also means that it is compatible with current game engines and graphics software, running much faster than real time. The model is licensed commercially to Body Labs Inc. and is made freely available for research purposes.
Members
Publications