Human Pose, Shape and Action
3D Pose from Images
2D Pose from Images
Beyond Motion Capture
Action and Behavior
Body Perception
Body Applications
Pose and Motion Priors
Clothing Models (2011-2015)
Reflectance Filtering
Learning on Manifolds
Markerless Animal Motion Capture
Multi-Camera Capture
2D Pose from Optical Flow
Body Perception
Neural Prosthetics and Decoding
Part-based Body Models
Intrinsic Depth
Lie Bodies
Layers, Time and Segmentation
Understanding Action Recognition (JHMDB)
Intrinsic Video
Intrinsic Images
Action Recognition with Tracking
Neural Control of Grasping
Flowing Puppets
Faces
Deformable Structures
Model-based Anthropometry
Modeling 3D Human Breathing
Optical flow in the LGN
FlowCap
Smooth Loops from Unconstrained Video
PCA Flow
Efficient and Scalable Inference
Motion Blur in Layers
Facade Segmentation
Smooth Metric Learning
Robust PCA
3D Recognition
Object Detection
Groups and Crowds
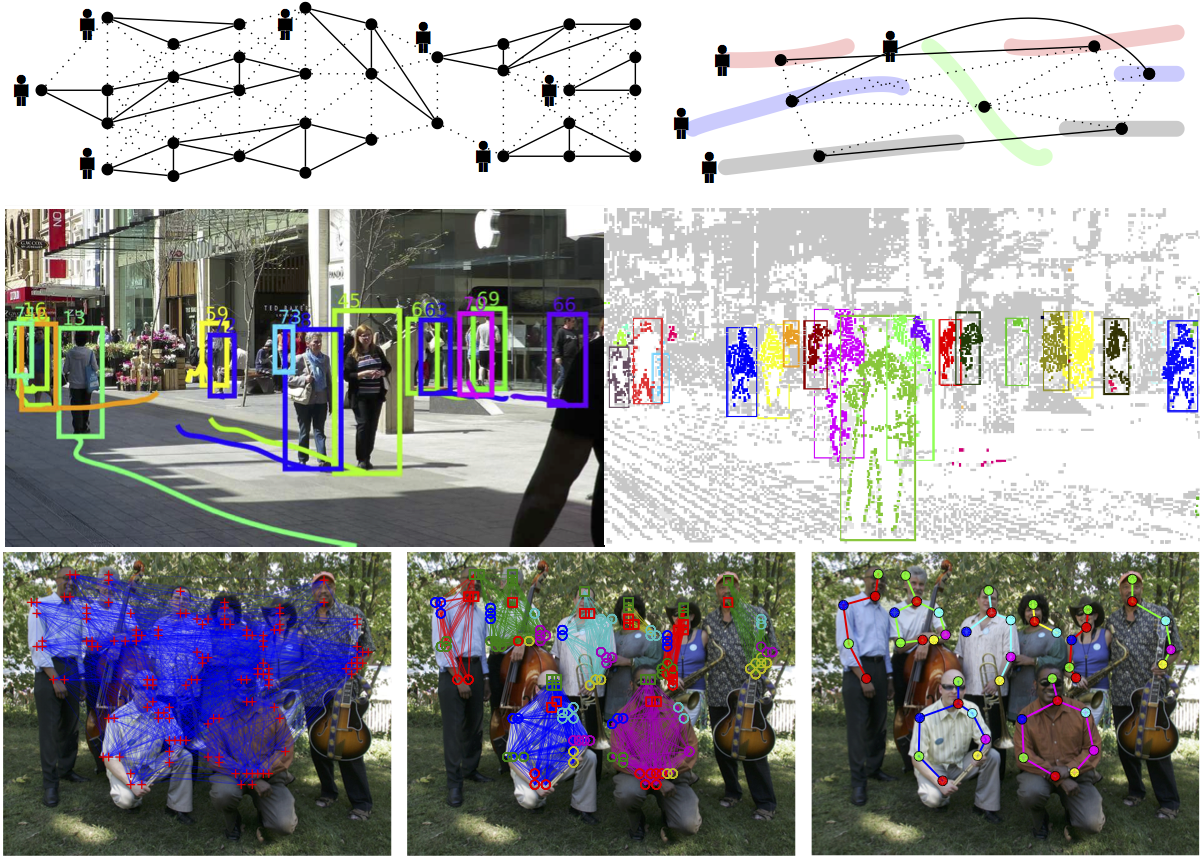
People are often a central element of visual scenes. It has been a long-standing goal in computer vision to develop computational models that enable machines to detect crowds of people, analyze their motion and poses, infer their actions and reason about the consequences. Our research addresses a wide range of challenges in visual understanding of people in real-world crowded scenes. These include multi-person tracking [] [
], multi-person pose estimation [
], segmentation [
] and person re-identification [
].
For multi-target tracking, our work [] proposed to link, cluster and track targets jointly across space and time. We defined a novel mathematical abstraction for tracking in the form of a minimum cost multicut problem. In order to avoid that distinct but similar looking targets are assigned to the same track, we formulated tracking as a minimum cost lifted multicut problem [
].
Our work [] presented a novel method to re-identify people in different images, where a second-pooling method is utilized to fuse the feature maps from the pose and the appearance estimator. The method significantly advanced the state-of-the-art on many challenging public benchmarks.
This work forms a foundation for our ongoing work on estimating detailed 3D motions of people in crowded scenes.
Members
Publications