Quantifying the Quality of Haptic Interfaces
Shape-Changing Haptic Interfaces
Generating Clear Vibrotactile Cues with Magnets Embedded in a Soft Finger Sheath
Salient Full-Fingertip Haptic Feedback Enabled by Wearable Electrohydraulic Actuation
Cutaneous Electrohydraulic (CUTE) Wearable Devices for Pleasant Broad-Bandwidth Haptic Cues
Modeling Finger-Touchscreen Contact during Electrovibration
Perception of Ultrasonic Friction Pulses
Vibrotactile Playback for Teaching Sensorimotor Skills in Medical Procedures
CAPT Motor: A Two-Phase Ironless Motor Structure
4D Intraoperative Surgical Perception: Anatomical Shape Reconstruction from Multiple Viewpoints
Visual-Inertial Force Estimation in Robotic Surgery
Enhancing Robotic Surgical Training
AiroTouch: Naturalistic Vibrotactile Feedback for Large-Scale Telerobotic Assembly
Optimization-Based Whole-Arm Teleoperation for Natural Human-Robot Interaction
Finger-Surface Contact Mechanics in Diverse Moisture Conditions
Computational Modeling of Finger-Surface Contact
Perceptual Integration of Contact Force Components During Tactile Stimulation
Dynamic Models and Wearable Tactile Devices for the Fingertips
Novel Designs and Rendering Algorithms for Fingertip Haptic Devices
Dimensional Reduction from 3D to 1D for Realistic Vibration Rendering
Prendo: Analyzing Human Grasping Strategies for Visually Occluded Objects
Learning Upper-Limb Exercises from Demonstrations
Minimally Invasive Surgical Training with Multimodal Feedback and Automatic Skill Evaluation
Efficient Large-Area Tactile Sensing for Robot Skin
Haptic Feedback and Autonomous Reflexes for Upper-limb Prostheses
Gait Retraining
Modeling Hand Deformations During Contact
Intraoperative AR Assistance for Robot-Assisted Minimally Invasive Surgery
Immersive VR for Phantom Limb Pain
Visual and Haptic Perception of Real Surfaces
Haptipedia
Gait Propulsion Trainer
TouchTable: A Musical Interface with Haptic Feedback for DJs
Exercise Games with Baxter
Intuitive Social-Physical Robots for Exercise
How Should Robots Hug?
Hierarchical Structure for Learning from Demonstration
Fabrication of HuggieBot 2.0: A More Huggable Robot
Learning Haptic Adjectives from Tactile Data
Feeling With Your Eyes: Visual-Haptic Surface Interaction
S-BAN
General Tactile Sensor Model
Insight: a Haptic Sensor Powered by Vision and Machine Learning
Learning Upper-Limb Exercises from Demonstrations
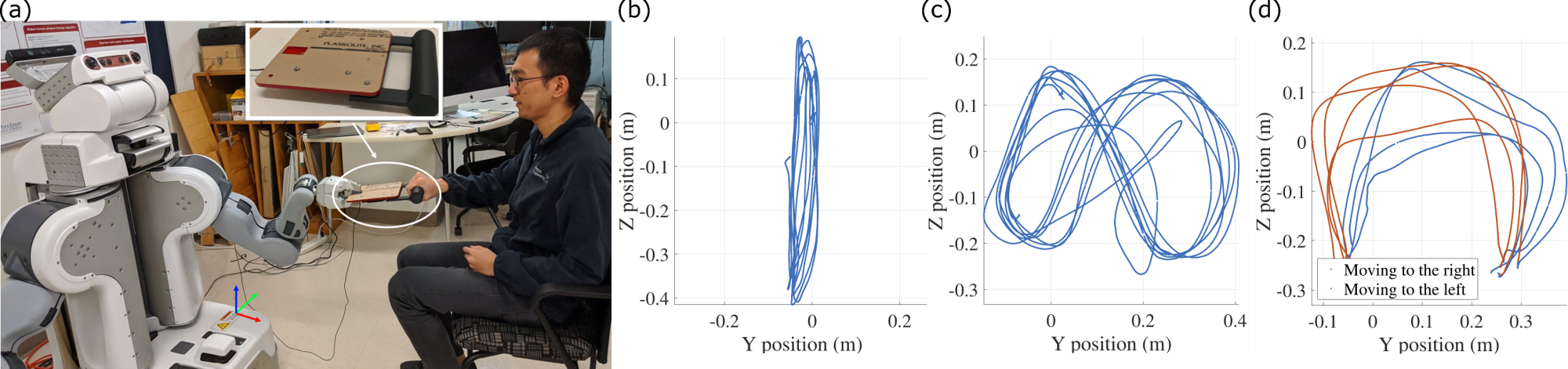
Compared to conventional upper-limb therapies, robotic devices can offer multiple advantages in physical training and rehabilitation. Automation can reduce the workload of rehabilitation professionals and augment their ability to provide care to patients by facilitating intensive and repeatable exercise. Additionally, robots can provide objective assessments of a patient’s progress using onboard sensors.
In the scope of this research, we developed a learning-from-demonstration (LfD) technique that enables a general-purpose humanoid robot to lead a user through object-mediated upper-limb exercises []. Built upon our prior research [
], our approach requires only tens of seconds of training data from a therapist teleoperating the robot to do the task with the user.
We model the robot behavior as a regression problem: during training, the joint distribution of robot position, velocity, and effort (force output at the end-effector) are modeled by a Gaussian mixture model (GMM), and during testing, desired robot effort is regressed from the current state (position and velocity) from the GMM. Compared to the conventional approach of learning time-based trajectories, our state-based strategy produces customized robot behavior and eliminates the need to tune gains to adapt to the user’s motor ability.
This approach was evaluated through a user study involving one occupational therapist and six people with stroke []. The therapist trained a Willow Garage PR2 on three example tasks for each client: i) periodic 1D motions, ii) periodic 2D motions, and iii) episodic pick and place operations. Both the person with stroke and the therapist then repeatedly performed the tasks alone with the robot and blindly compared the state- and time-based controllers learned from the training data.
Our results show that working models were reliably learned to enable the robot to do the exercise with the user. Furthermore, our state-based approach enabled users to be more actively involved, allowed larger excursion, and generated power outputs more similar to the therapist demonstrations. Finally, the therapist found our strategy more agreeable than the traditional time-based approach. More detailed descriptions of this project’s algorithms and results can be found in the Ph.D. thesis of Siyao Hu [].
Members
Publications