Quantifying the Quality of Haptic Interfaces
Shape-Changing Haptic Interfaces
Generating Clear Vibrotactile Cues with Magnets Embedded in a Soft Finger Sheath
Salient Full-Fingertip Haptic Feedback Enabled by Wearable Electrohydraulic Actuation
Cutaneous Electrohydraulic (CUTE) Wearable Devices for Pleasant Broad-Bandwidth Haptic Cues
Modeling Finger-Touchscreen Contact during Electrovibration
Perception of Ultrasonic Friction Pulses
Vibrotactile Playback for Teaching Sensorimotor Skills in Medical Procedures
CAPT Motor: A Two-Phase Ironless Motor Structure
4D Intraoperative Surgical Perception: Anatomical Shape Reconstruction from Multiple Viewpoints
Visual-Inertial Force Estimation in Robotic Surgery
Enhancing Robotic Surgical Training
AiroTouch: Naturalistic Vibrotactile Feedback for Large-Scale Telerobotic Assembly
Optimization-Based Whole-Arm Teleoperation for Natural Human-Robot Interaction
Finger-Surface Contact Mechanics in Diverse Moisture Conditions
Computational Modeling of Finger-Surface Contact
Perceptual Integration of Contact Force Components During Tactile Stimulation
Dynamic Models and Wearable Tactile Devices for the Fingertips
Novel Designs and Rendering Algorithms for Fingertip Haptic Devices
Dimensional Reduction from 3D to 1D for Realistic Vibration Rendering
Prendo: Analyzing Human Grasping Strategies for Visually Occluded Objects
Learning Upper-Limb Exercises from Demonstrations
Minimally Invasive Surgical Training with Multimodal Feedback and Automatic Skill Evaluation
Efficient Large-Area Tactile Sensing for Robot Skin
Haptic Feedback and Autonomous Reflexes for Upper-limb Prostheses
Gait Retraining
Modeling Hand Deformations During Contact
Intraoperative AR Assistance for Robot-Assisted Minimally Invasive Surgery
Immersive VR for Phantom Limb Pain
Visual and Haptic Perception of Real Surfaces
Haptipedia
Gait Propulsion Trainer
TouchTable: A Musical Interface with Haptic Feedback for DJs
Exercise Games with Baxter
Intuitive Social-Physical Robots for Exercise
How Should Robots Hug?
Hierarchical Structure for Learning from Demonstration
Fabrication of HuggieBot 2.0: A More Huggable Robot
Learning Haptic Adjectives from Tactile Data
Feeling With Your Eyes: Visual-Haptic Surface Interaction
S-BAN
General Tactile Sensor Model
Insight: a Haptic Sensor Powered by Vision and Machine Learning
Enhancing Robotic Surgical Training
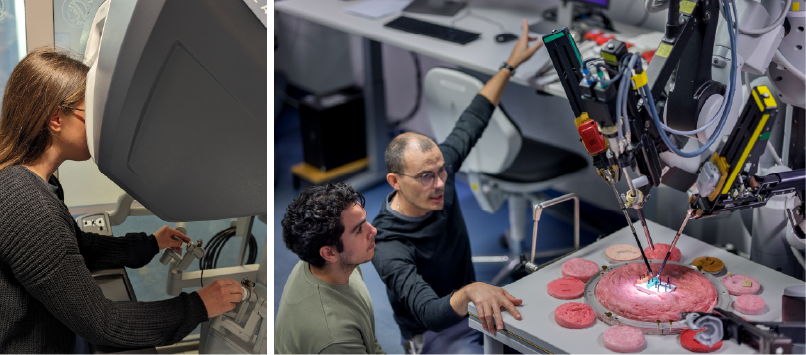
Robot-Assisted Minimally Invasive Surgery (RMIS) allows surgeons to perform procedures through tiny incisions, thus reducing healing time compared to traditional open surgery. However, surgeons' technical competence remains essential for achieving positive patient outcomes. Therefore, in collaboration with several surgeons, we are investigating a range of methods to improve RMIS training.
We explored the effects of haptic feedback while training in RMIS. First, we investigated vibrotactile feedback using VerroTouch, a previously developed system that allows the surgeon to feel the instruments' vibrations at the operator's manipulators. Trainees who were provided with the vibrotactile feedback showed reduced workload and enhanced experience during both simulation and in real post-training surgeries compared to those who trained with no vibrotactile feedback []. Ongoing work is examining the impact of this feedback on learning processes. We also explored force feedback and created bracelets that squeeze the operator's wrists proportionally to the force applied by the surgical instruments. Participants applied significantly less force on the task materials when receiving the feedback [
].
We identified two additional challenges when training in RMIS: the quantitative evaluation of surgical skills and the limits of existing simulators in facilitating self-directed learning.
Currently, surgical skill assessment is mainly conducted through manual video evaluation, which is time-consuming and subject to bias. We showed that surgical skill can be estimated through completion time, force applied to task materials, and vibrations generated at the surgical instruments []. Additionally, we explored the effect of an automated machine-learning-based skill-assessment approach on psychomotor skill development. We found that while some automated assessment tools may not enhance skill improvement rates, they can increase trainee self-awareness regarding their performance [
].
Finally, effective training programs require experienced surgeons to provide feedback, but their time is limited and expensive. Therefore, there is a growing need for simulators that enable self-directed learning and provide real-time automatic formative feedback. To facilitate self-directed learning, we developed a platform that allows expert surgeons to record surgical procedures with multiple modalities and novice surgeons to replay and train with multimodal recordings, including visual, auditory, and vibrotactile feedback []. In ongoing projects, we are investigating the use of various signals to automatically monitor the task performance of trainees and deliver real-time customized visual and/or haptic feedback.
This research project involves collaborations with Gina L. Adrales (Johns Hopkins Medical Institute), Timothy Bernard (University of Maryland Baltimore County), Jeremy D. Brown (Johns Hopkins University), Eric Cao (Johns Hopkins University), Amy Chi (Johns Hopkins University), Sean P. Cohen (University of Pennsylvania), Kristoffel R. Dumon (University of Pennsylvania Hospital System), Joshua N. Fernandez (University of Maryland Baltimore County), Ernest D. Gomez (University of Pennsylvania; Beth Israel Deaconess Medical Center; Harvard Medical School), David I. Lee (University of Pennsylvania Hospital System), Sarah C. Leung (University of Pennsylvania), Sergio Machaca (Johns Hopkins University), Conor E. O'Brien (University of Pennsylvania), Zachary Patterson (Carnegie Mellon University), Noel N. Williams (University of Pennsylvania), and Brett Wolfinger (Johns Hopkins University).
Members
Publications