Quantifying the Quality of Haptic Interfaces
Shape-Changing Haptic Interfaces
Generating Clear Vibrotactile Cues with Magnets Embedded in a Soft Finger Sheath
Salient Full-Fingertip Haptic Feedback Enabled by Wearable Electrohydraulic Actuation
Cutaneous Electrohydraulic (CUTE) Wearable Devices for Pleasant Broad-Bandwidth Haptic Cues
Modeling Finger-Touchscreen Contact during Electrovibration
Perception of Ultrasonic Friction Pulses
Vibrotactile Playback for Teaching Sensorimotor Skills in Medical Procedures
CAPT Motor: A Two-Phase Ironless Motor Structure
4D Intraoperative Surgical Perception: Anatomical Shape Reconstruction from Multiple Viewpoints
Visual-Inertial Force Estimation in Robotic Surgery
Enhancing Robotic Surgical Training
AiroTouch: Naturalistic Vibrotactile Feedback for Large-Scale Telerobotic Assembly
Optimization-Based Whole-Arm Teleoperation for Natural Human-Robot Interaction
Finger-Surface Contact Mechanics in Diverse Moisture Conditions
Computational Modeling of Finger-Surface Contact
Perceptual Integration of Contact Force Components During Tactile Stimulation
Dynamic Models and Wearable Tactile Devices for the Fingertips
Novel Designs and Rendering Algorithms for Fingertip Haptic Devices
Dimensional Reduction from 3D to 1D for Realistic Vibration Rendering
Prendo: Analyzing Human Grasping Strategies for Visually Occluded Objects
Learning Upper-Limb Exercises from Demonstrations
Minimally Invasive Surgical Training with Multimodal Feedback and Automatic Skill Evaluation
Efficient Large-Area Tactile Sensing for Robot Skin
Haptic Feedback and Autonomous Reflexes for Upper-limb Prostheses
Gait Retraining
Modeling Hand Deformations During Contact
Intraoperative AR Assistance for Robot-Assisted Minimally Invasive Surgery
Immersive VR for Phantom Limb Pain
Visual and Haptic Perception of Real Surfaces
Haptipedia
Gait Propulsion Trainer
TouchTable: A Musical Interface with Haptic Feedback for DJs
Exercise Games with Baxter
Intuitive Social-Physical Robots for Exercise
How Should Robots Hug?
Hierarchical Structure for Learning from Demonstration
Fabrication of HuggieBot 2.0: A More Huggable Robot
Learning Haptic Adjectives from Tactile Data
Feeling With Your Eyes: Visual-Haptic Surface Interaction
S-BAN
General Tactile Sensor Model
Insight: a Haptic Sensor Powered by Vision and Machine Learning
Learning Haptic Adjectives from Tactile Data
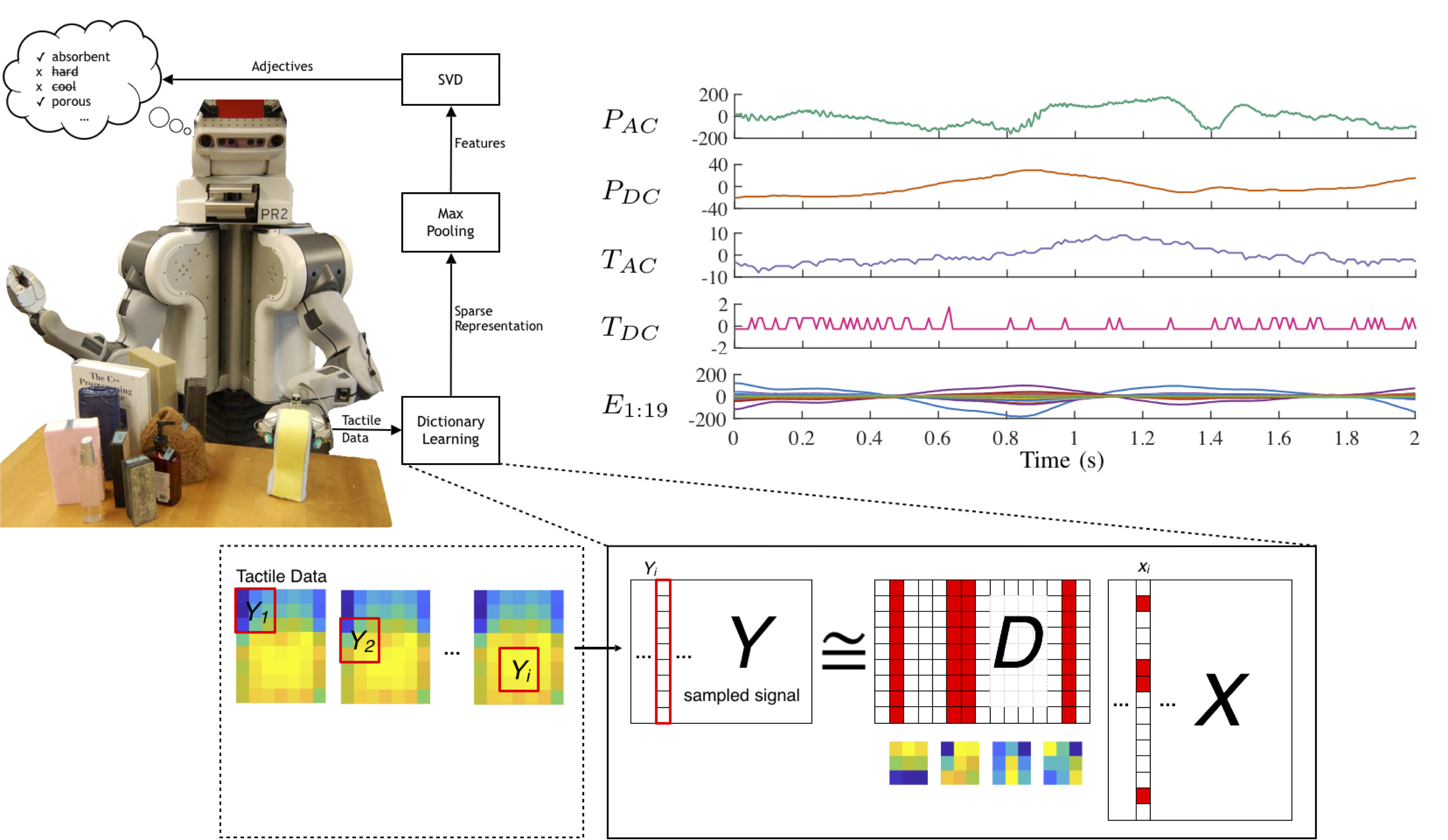
Humans can form an impression of how a new object feels simply by touching its surfaces with the densely innervated skin of the fingertips. Recent research has focused on endowing robots with similar levels of haptic intelligence, but these efforts are usually limited to specific applications and make strong assumptions about the underlying structure of the haptic signals. By contrast, unsupervised machine learning can discover important underlying structure without biases for specific applications; such representations can then be effectively applied to a variety of learning tasks.
Our work applies unsupervised feature-learning methods to multimodal haptic data (fingertip deformation, pressure, vibration, and temperature) that was previously collected using a Willow Garage PR2 robot equipped with a pair of SynTouch BioTac sensors. The robot used four pre-programmed exploratory procedures (EPs) to touch sixty unique objects, each of which was separately labeled by blindfolded humans using haptic adjectives from a finite set. The raw haptic data and human labels are contained in the Penn Haptic Adjective Corpus (PHAC-2) dataset.
We are particularly interested in how representations derived from this data set depend on EPs and sensory modalities, and how accurately they classify across the set of adjectives. Do representations learned from certain EPs and data streams classify texture-related adjectives better than others? Can variability in performance be predicted by properties of the learned representations? Is it necessary to learn a different representation for each EP, or does enough shared information exist to use a combined representation?
Our work shows that learned features are far superior to hand-crafted features. Additionally, the features learned from certain EPs and sensory modalities provide a better basis for adjective classification than those from others. We are also investigating dependencies between EPs and sensory modalities, relationships between learned representations, and ordinal (rather than binary) adjectives.
Members
Publications