Enhanced Flexible Mold Lifetime for Roll‐to‐Roll Scaled‐Up Manufacturing of Adhesive Complex Microstructures
Bioinspired Microstructured Adhesives with Facile and Fast Switchability for Part Manipulation in Dry and Wet Conditions
Smart Materials for manipulation and actuation of small-scale structures
3D nanofabrication of various materials for advanced multifunctional microrobots
Liquid Crystal Mesophase of Supercooled Liquid Gallium And Eutectic Gallium–Indium
Machine Learning-Based Pull-off and Shear Optimal Adhesive Microstructures
Information entropy to detect order in self-organizing systems
Individual and collective manipulation of multifunctional bimodal droplets in three dimensions
Microrobot collectives with reconfigurable morphologies and functions
Self-organization in heterogeneous and non-reciprocal regime
Biomimetic Emulsion Systems
Giant Unilamellar Vesicles for Designing Cell-like Microrobots
Bioinspired self-assembled colloidal collectives drifting in three dimensions underwater
Machine learning methods for soft millirobot design and control
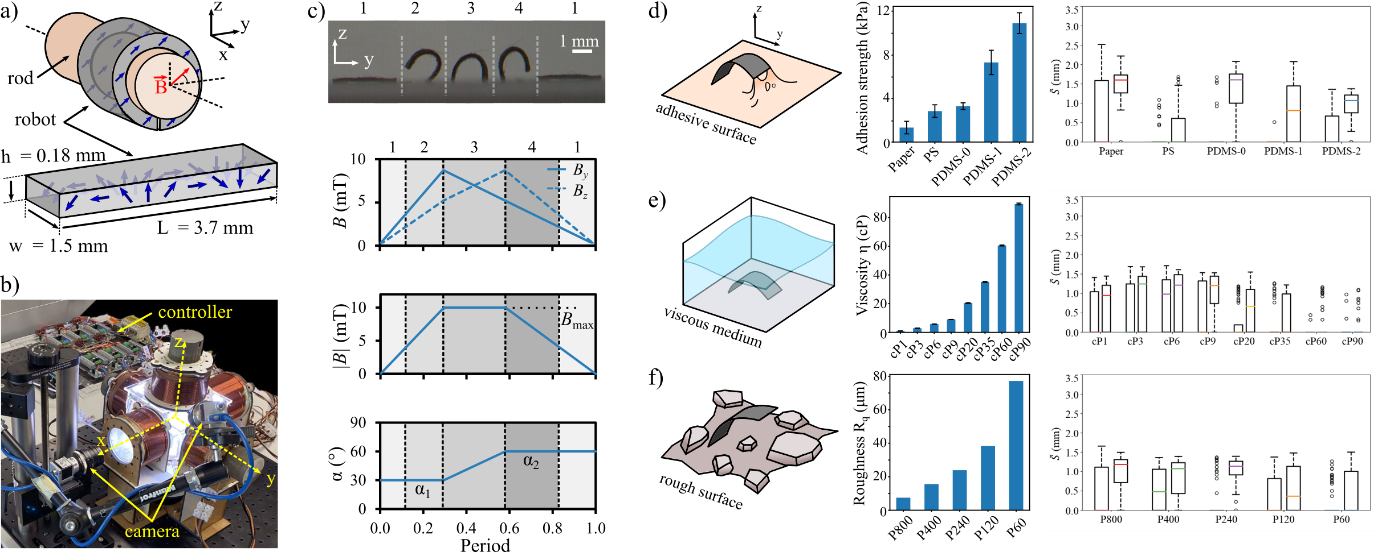
Untethered small-scale soft robots have promising applications in minimally invasive surgery, targeted drug delivery, and bioengineering applications as they can directly and non-invasively access confined and hard-to-reach spaces in the human body. For such potential biomedical applications, the adaptivity of the robot control is essential to ensure the continuity of the operations, as task environment conditions show dynamic variations that can alter the robot’s motion and task performance. The applicability of the conventional modeling and control methods is further limited for small soft robots due to their kinematics with virtually infinite degrees of freedom, inherent stochastic variability during the fabrication, and changing dynamics during real-world interactions. To address the controller adaptation challenge to dynamically changing task environments, we propose in [] using a probabilistic learning approach for a millimeter-scale magnetic walking soft robot using Bayesian optimization (BO) and Gaussian processes (GPs). Our approach provides a data-efficient learning scheme by finding the gait controller parameters while optimizing the stride length of the walking soft millirobot using a limited number of physical experiments. To demonstrate the controller adaptation, in [
], we test the walking gait of the robot in task environments with different adhesion properties, medium viscosities, and surface roughnesses. We also utilize the transfer of the learned GP model among different task spaces and robots and studied its effect on improving controller learning performance.
Members
Publications